Peacock: Tackling ML challenges by accelerating skills
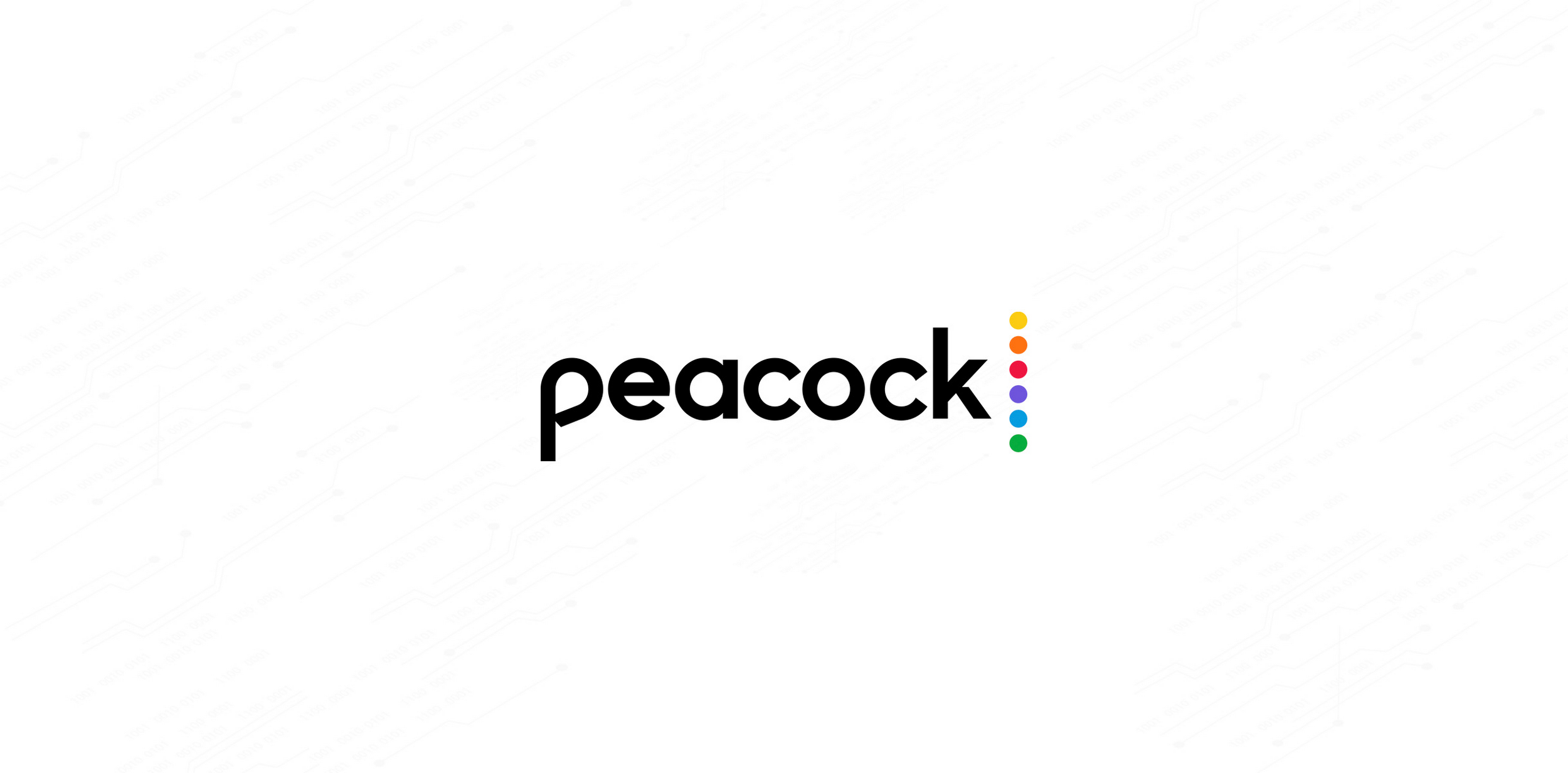
Kris Lachor
Director of Analytics Tooling, Peacock
Sharad Kala
Customer Experience Executive, Google Cloud
At Peacock, we are acutely aware of global trends and changes in adopting machine learning (ML) techniques, particularly in the field of media and entertainment. We anticipate that, within several years, most software applications will have an element of ML and will require fine tuning of a model, putting increasing demand on ML training infrastructure.
As the Director of Analytics Tooling at Peacock, I head up a team of engineers whose primary aim is to build scalability into our tools and processes, enabling us to keep up with the ever-changing field of ML engineering and create a better user experience. We use ML for a wide range of tasks, such as learning more about users’ viewing preferences and interests so we can provide more personalized content recommendations.
But the discipline of ML engineering is unique. It requires people from two significantly different backgrounds, applied ML and software engineering, to meet in the middle, collaborate, and learn from each other. Both groups face challenges they have not experienced before, regularly pushing them out of their comfort zones and catalyzing true innovation.
Continuous education in life is empowering and beneficial, but in the case of remaining competitive in our industry, it is necessary. As we build, scale, and iterate our tools and processes, we rely on training, resources, and education from Google Cloud. Here’s a look at how we invest in upskilling initiatives to scale our data science organization.
Accelerated training with Google Cloud Advanced Solutions Lab
As a relatively new and complex discipline, ML engineering has not yet seen established patterns and standards, making ongoing training essential for keeping up with industry changes. We have worked closely with Google Cloud for several years to develop our data analytics based on solutions like BigQuery, so when it came to providing ongoing education to our data scientists and engineers, Google Cloud was the natural choice.
We encourage our engineers and data scientists to earn Google Cloud certifications, such as the Professional Cloud Architect and Professional Data Engineer. In addition, Google Cloud offers on-demand training to understand, implement, and scale data science and ML tools, like these role-based learning paths for Data Engineers and ML Engineers. We also use Cloud Hero, a gamified Google Cloud training experience that uses hands-on labs to teach skills in an interactive learning environment.
Perhaps the most impactful offering has been the Advanced MLOps workshop from the Google Cloud Advanced Solutions Lab. Participants were split roughly fifty-fifty between engineers and data scientists, from six or seven teams across multiple organizations. Being in the same workshop allowed us to establish a baseline among multidisciplinary teams, and to create a common vocabulary and a shared understanding of the problems we face.
This immersive MLOps deep dive offered something for everyone, no matter the participant’s background. The first week focused on containers, Kubernetes, CI/CD, and ML pipelines—in other words, the topics that would have been a refresher to a good engineer but unfamiliar to some data scientists. This changed in the second week, as we moved on to building models and the basics of TensorFlow and TFX components. At this point, the data scientists felt more in their element, while some engineers were experiencing it for the first time. The Advanced Solutions Lab served as a melting pot for teams that previously had not collaborated with each other, helping everyone learn and grow together.
"Peacock’s ML Engineering team has done an amazing job in creating and implementing a robust training program and we are proud to be their Partner of choice. The team members have thoroughly adopted a growth mindset and are continually pursuing strategic opportunities to learn and grow their technical capabilities, and we’re excited to be a part of it."—Heather Remek, Head of Customer Experience - Telecommunications Industry, Google Cloud
Educating today to build for tomorrow
We are operating in a new and fast-changing industry, but tooling, processes, or compute resources should not stand in the way of creating better ML models. With the right tools, techniques, and mindset, data scientists and ML engineers can develop the skills necessary to excel and progress in our field.
As ML evolves, we’ll continue to stay informed, practice on real-world problems, and invest in our upskilling programs. Our collaboration with the Google Cloud team on our ML journey has allowed us to increase the knowledge and competency of our data science teams as they build, productionalize, and scale end-to-end ML solutions. By educating today, we can empower our organization to build for tomorrow and make better data-driven decisions.
Learn more about the Google Cloud Advanced Solutions Lab and explore on-demand role based training for data scientists and ML engineers.