Generative AI with enterprise controls for business users in 24 Hours
Dr. Ali Arsanjani
Director, Applied AI Engineering, Google
Arijit Sengupta
Founder and CEO, Aible
Aible is a leader in generating business impact from AI in less than 30 days, helping teams use AI to extract enterprise value from raw enterprise data with solutions for customer acquisition, churn prevention, demand prediction, preventative maintenance, and more. After previously leveraging BigQuery’s serverless architecture to reduce analytics costs, Aible is now collaborating with Google Cloud to enable customers to build, train, and deploy generative AI models on their own data, securely and with confidence.
As awareness of the potential of generative AI expands in the market, the following key considerations have emerged:
-
Enabling enterprise-grade control: Organizations want to enable gen AI experiences on their enterprise data, but they also want to ensure they have control over their data, so it’s not inadvertently used to train AI models without their knowledge.
-
Minimizing and mitigating hallucinations: Another specific gen AI risk is the potential for models to hallucinate — generate non-factual or nonsensical content.
-
Empowering business users: While gen AI enables numerous enterprise use cases, some of the most valuable use cases focus on enabling and empowering business users to leverage gen AI models with as little friction possible.
-
Scaling gen AI use cases: Enterprises need a way to harvest and operationalize their most promising use cases at scale and set up consistent best practices and controls.
Most organizations have a low-risk tolerance when it comes to data privacy, policy, and regulatory compliance. At the same time, they don’t consider delaying gen AI adoption as a viable option due to market and competitive pressures, especially given its promise for driving transformation. As a result, Aible wanted an AI approach that a wide variety of enterprise users could adopt and adjust quickly to a rapidly evolving landscape — all while keeping customer data secure.
Aible decided to leverage Vertex AI, Google Cloud’s AI platform, to ensure customers have confidence and full control over how their data is used and accessed when developing, training, or fine-tuning AI models.
Enabling enterprise-grade controls
Google Cloud’s design approach means customer data is secure by default on day 1 without requiring customers to take any additional actions. Google AI products and services provide security and privacy directly in your Google Cloud tenant projects. For instance, Vertex AI Agent Builder, Enterprise Search, and Conversation AI can all access and use secure customer data in Cloud Storage, which you can further secure using customer-managed encryption keys (CMEK).
Aible’s Infrastructure as Code approach allows you to leverage all of the benefits of Google Cloud directly in your own projects in a matter of minutes. The end-to-end experience is completely secured in the Vertex AI Model Garden, whether you choose Google gen AI models like Gemini, third-party models from Anthropic and Cohere, or open models, such as LLama or Gemma.
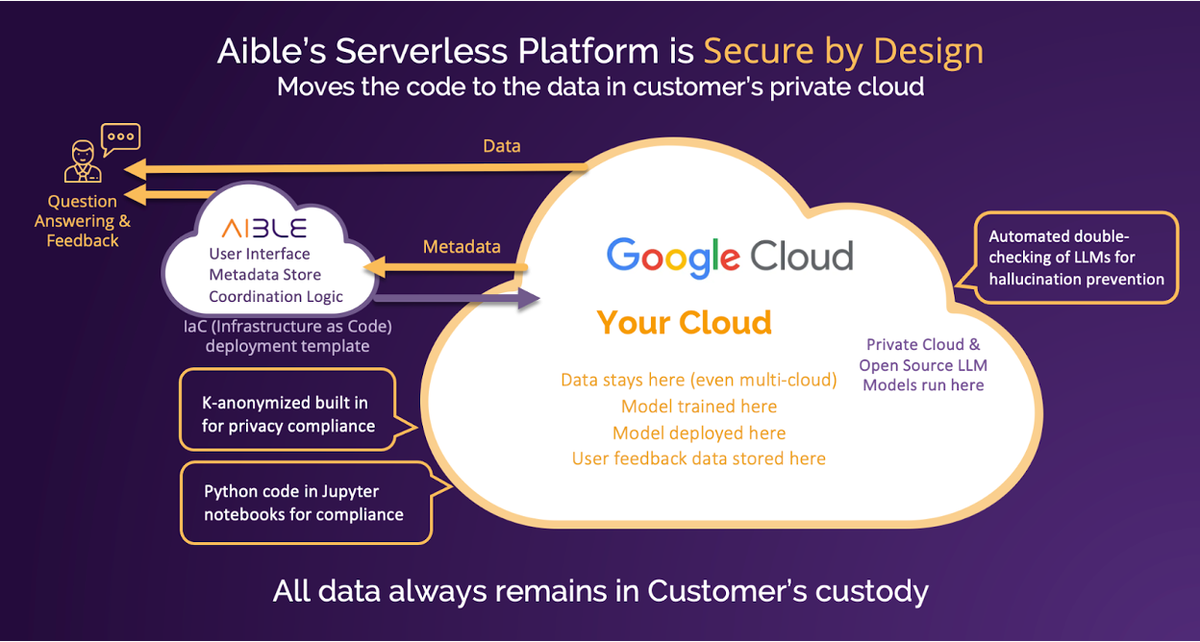
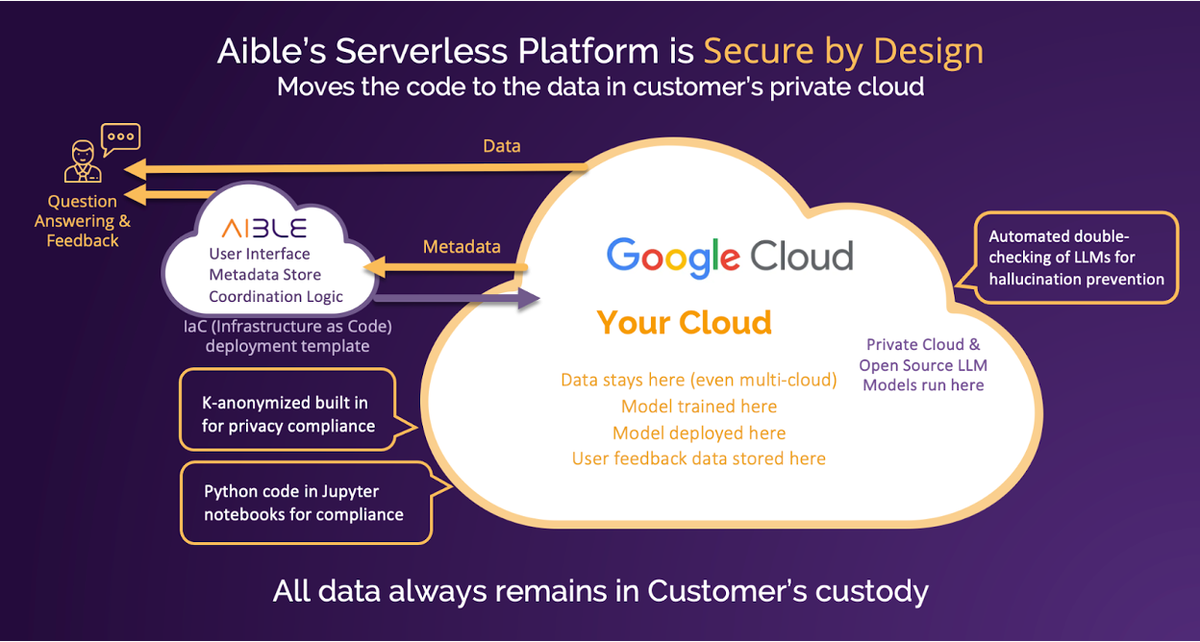
Aible also worked with its customer advisory board, which includes Fortune 100 companies, to design a solution that can invoke third-party gen AI models without exposing proprietary data outside of Google Cloud. Instead of sending raw data to an external model, Aible only sends high-level statistics on clusters, and this information can be masked as required. For example, it might send counts and averages based on geography or product instead of sending raw sales data.
This leverages a privacy technique called k-anonymity, which ensures data privacy by never providing information on groups of individuals smaller than k. You can change the default setting of k; the higher the k, the more private the information transfer. If masking is applied, Aible changes the name of a variable like “Country” to “Variable A” and a value like “Italy” to “Value X,” making the data transfer even more secure.
Mitigating hallucination risk
With gen AI, it’s critical to mitigate and reduce the risk of hallucinations using grounding, retrieval augmented generation (RAG), and other techniques. As a Built with Google Cloud AI partner, Aible provides automated analysis to augment human-in-the-loop review processes, empowering human experts with appropriate tools that can scale beyond manual efforts.
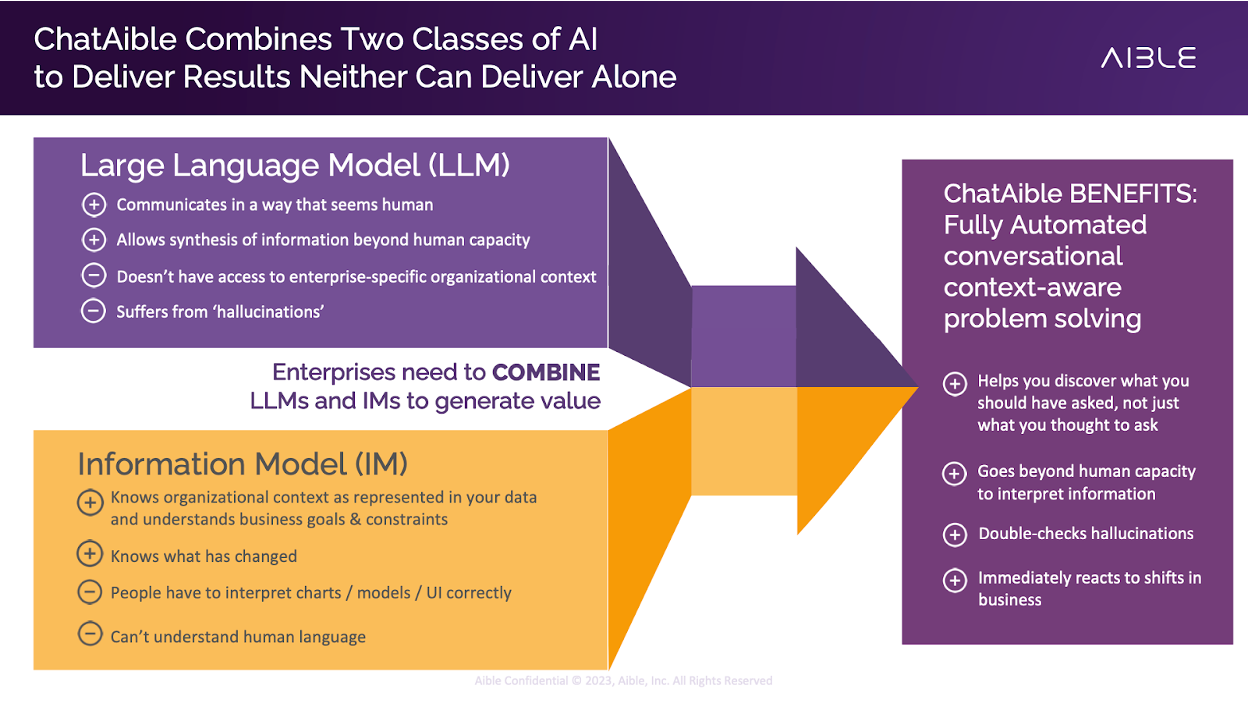
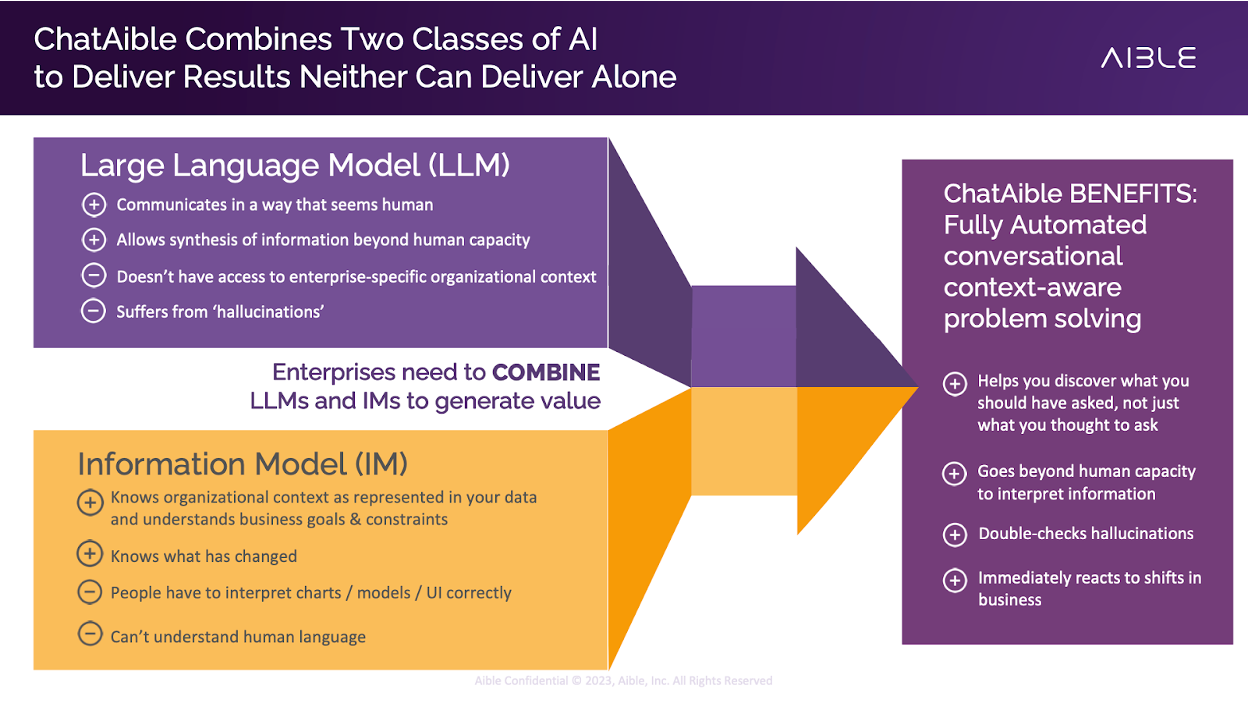
One of the primary ways Aible helps reduce hallucinations is by using its auto-generated Information Model (IM) — an explainable AI that double checks gen AI responses and confirms facts based on the context represented in your structured enterprise data at scale to prevent wrong decisions.
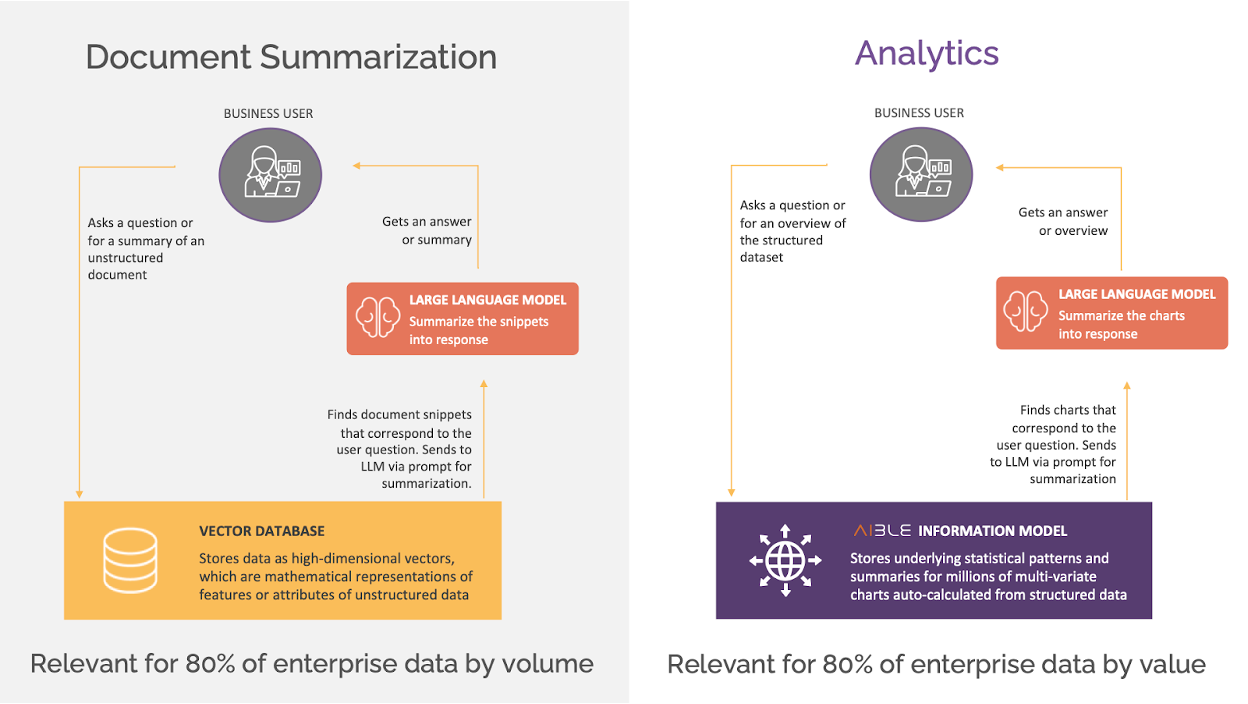
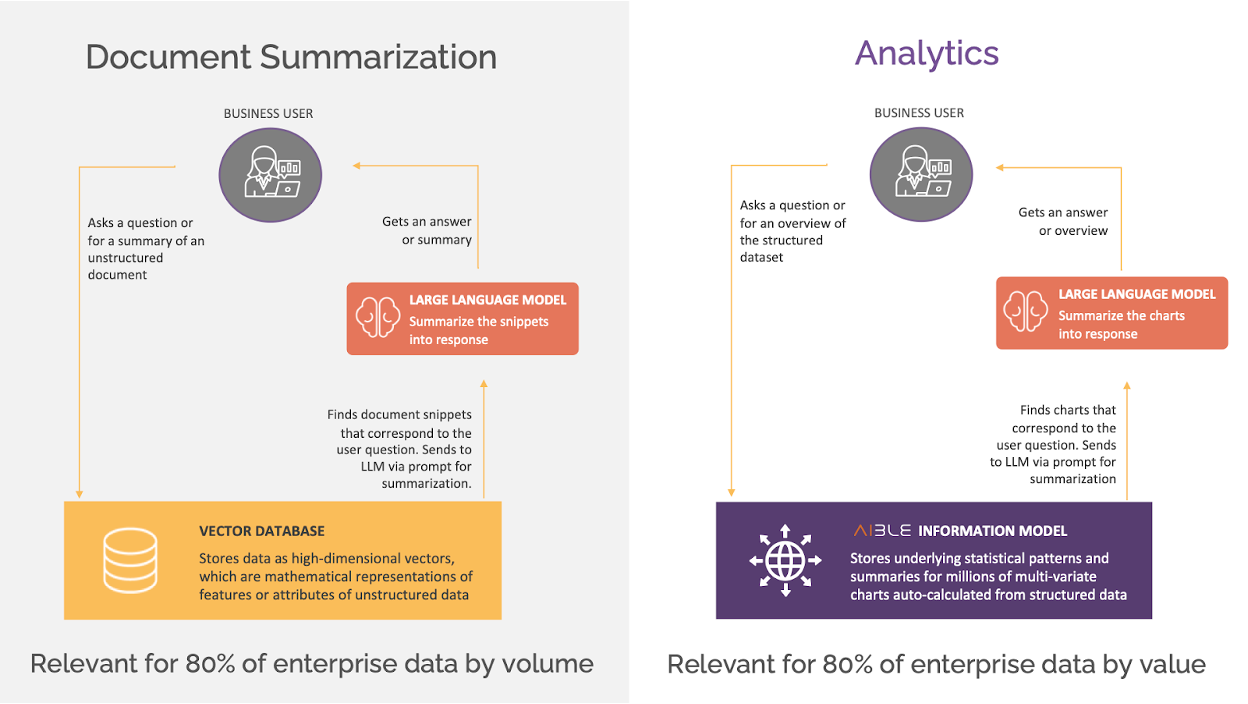
Aible’s Information Model addresses hallucinations in two ways:
-
The IM grounds gen AI models in a relevant subset of information, which has been shown to help reduce hallucination.
-
Aible parses through gen AI outputs and compares it to millions of answers already known to the Information Model to double check each fact.
This is similar to Google Cloud’s grounding capabilities on Vertex AI, which enables you to connect models to verifiable sources of information like your enterprise documents or the Internet to anchor responses in specific data sources. If a fact is automatically confirmed, it’s highlighted in blue — “If it’s blue, it’s true.” You can also check a specific pattern or variable and view a corresponding chart generated solely by the Information Model.
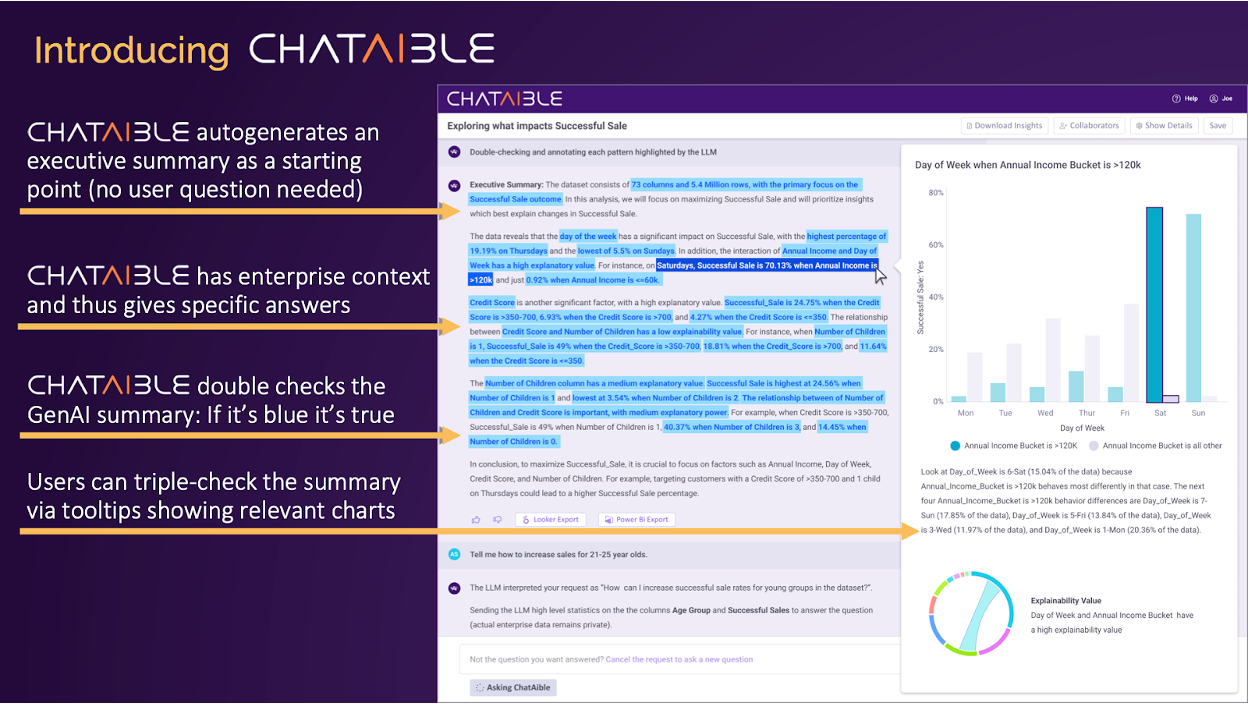
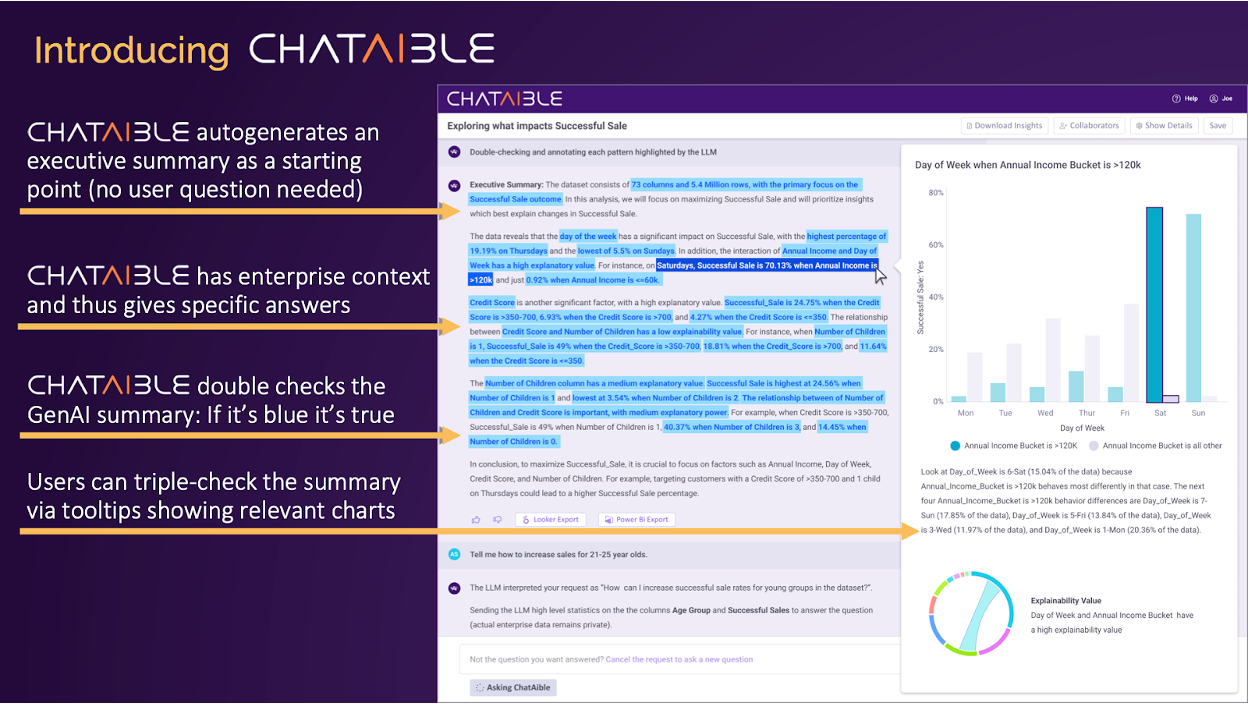
The diagram below shows how Aible and Google Cloud work together to deliver an AI-first end-to-end serverless environment. As Aible uses BigQuery to analyze and run serverless queries across millions of variable combinations with high efficiency, it is able to analyze any size dataset. For example, one of Aible and Google Cloud’s joint Fortune 500 customers was able to automatically analyze more than 75 datasets, with 100 million rows of data across 150 million questions and answers. The total cost of that evaluation was just $80.
By leveraging Vertex AI, Aible also has access to Model Garden, which includes Gemini and other leading open-source and third-party models.This means Aible is able to access non-Google gen AI models while benefiting from additional security layers, such as k-anonymity and masking.
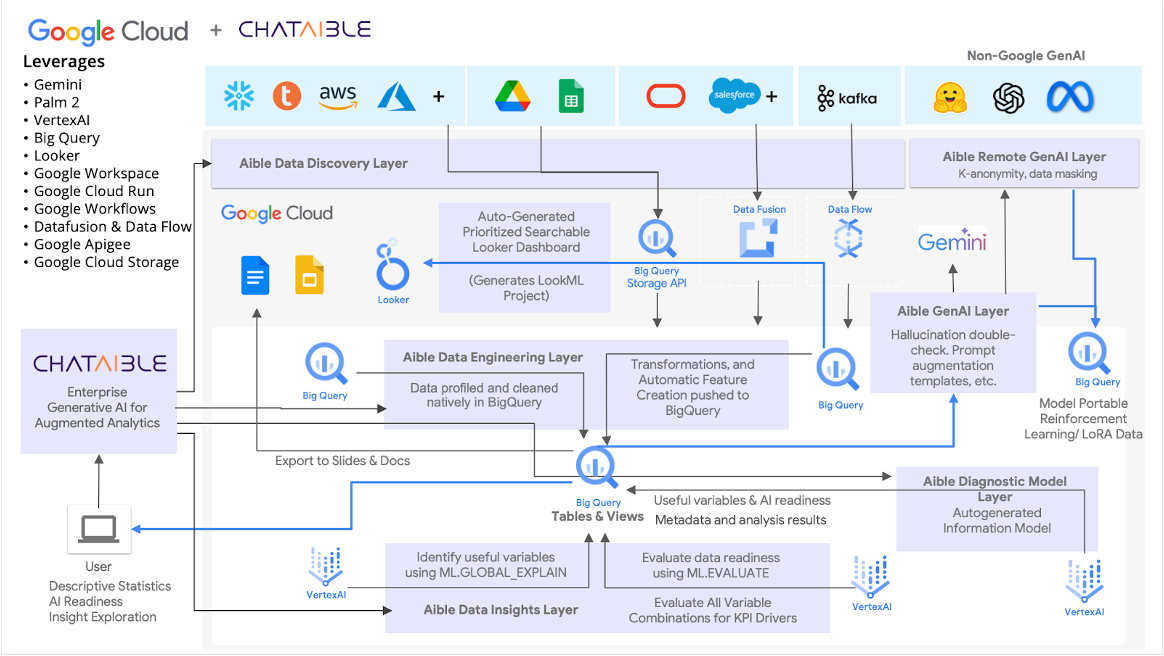
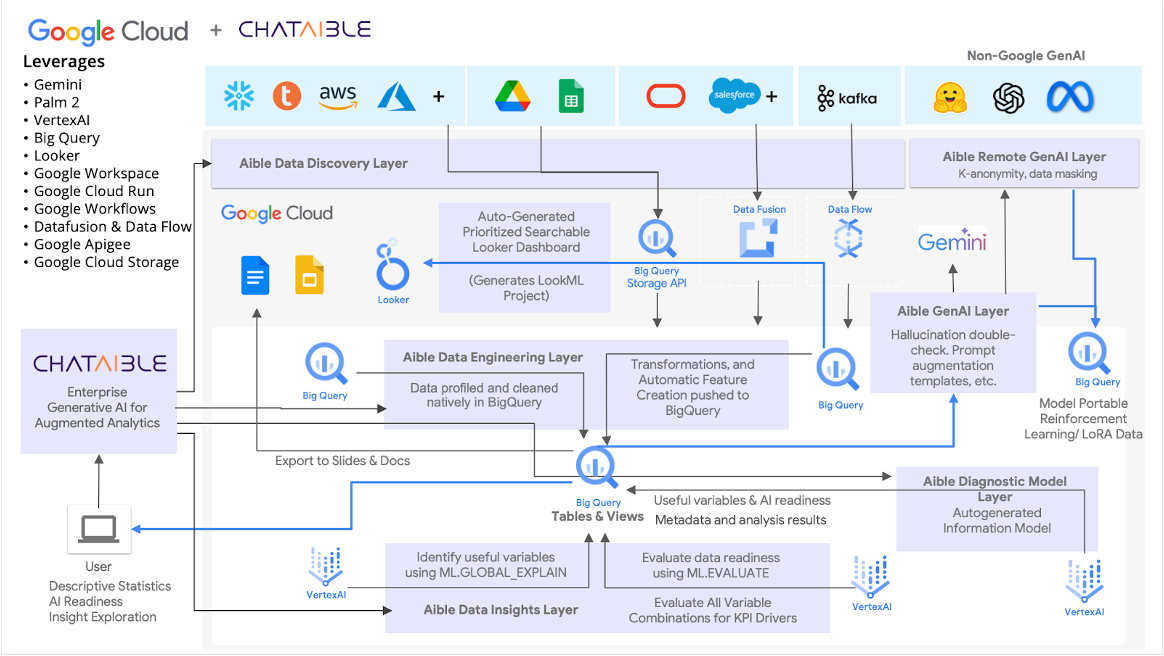
Aible never gets access to your data, which remains securely in your Google Cloud project, along with all feedback, reinforcement learning, and Low-Rank Adaptation (LoRA) data.
Empowering business users
Today, the standard gen AI journey starts with identifying a shortlist of use cases, identifying the relevant datasets, collecting reinforcement learning examples from business users, and developing a gen AI application or experience for each specific use case. Gen App Builder massively simplifies this process, automating many of the data science and development tasks. However, one key issue remains with this entire process — business users are not currently empowered to do these tasks themselves even though they are the best suited for determining viable use cases, providing feedback on output, and evaluating gen AI models.
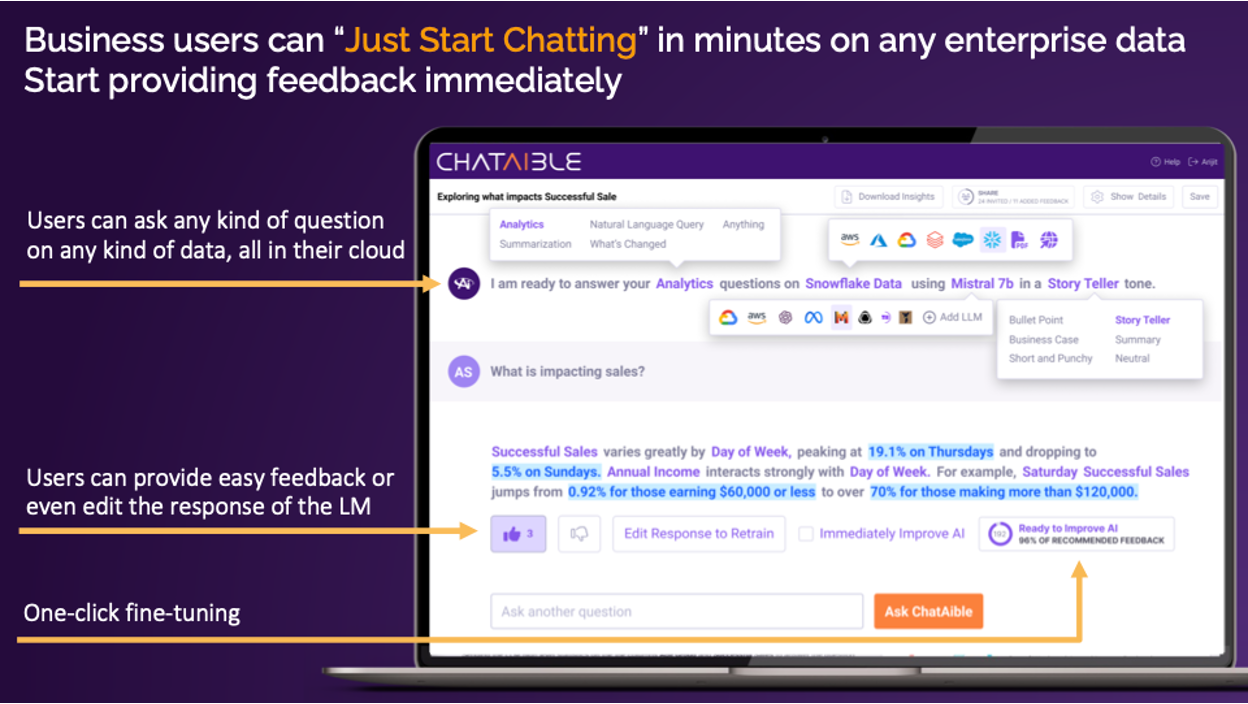
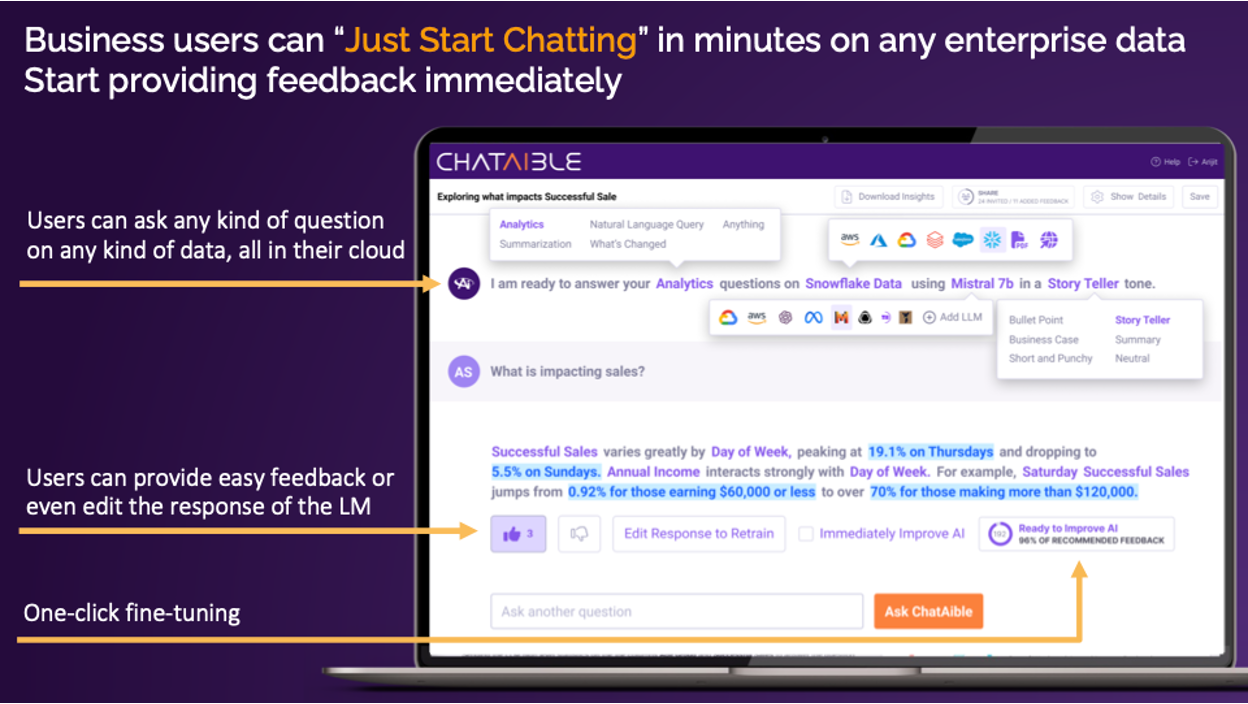
To unlock the innovation potential of your business users, it’s imperative that they understand gen AI techniques, how to use gen AI tools, and best practices for prompting. While investing in training is a valid solution, the rise of the citizen data scientist has taken a decade at this point. Instead of training, Aible approached this problem with technology designed specifically for business users.
Aible’s ChatAible system replaces months of manual work, providing a new gen AI experience that allows business users to “just start chatting” with their enterprise data.
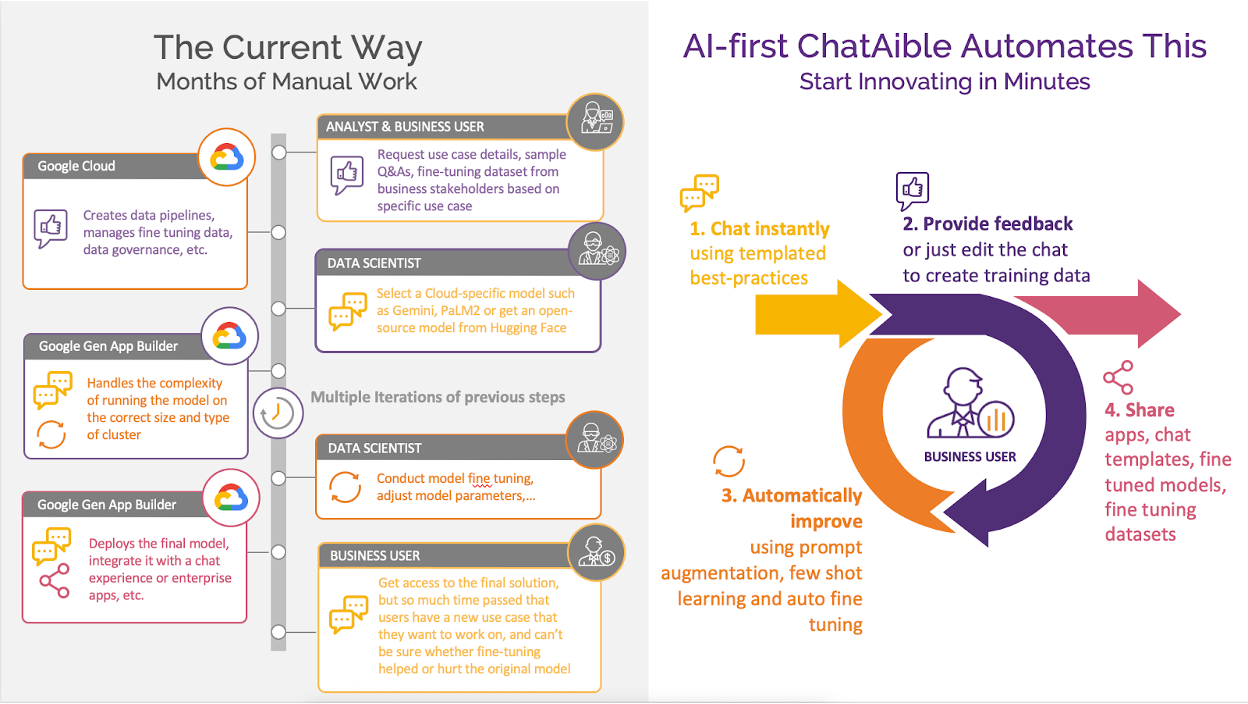
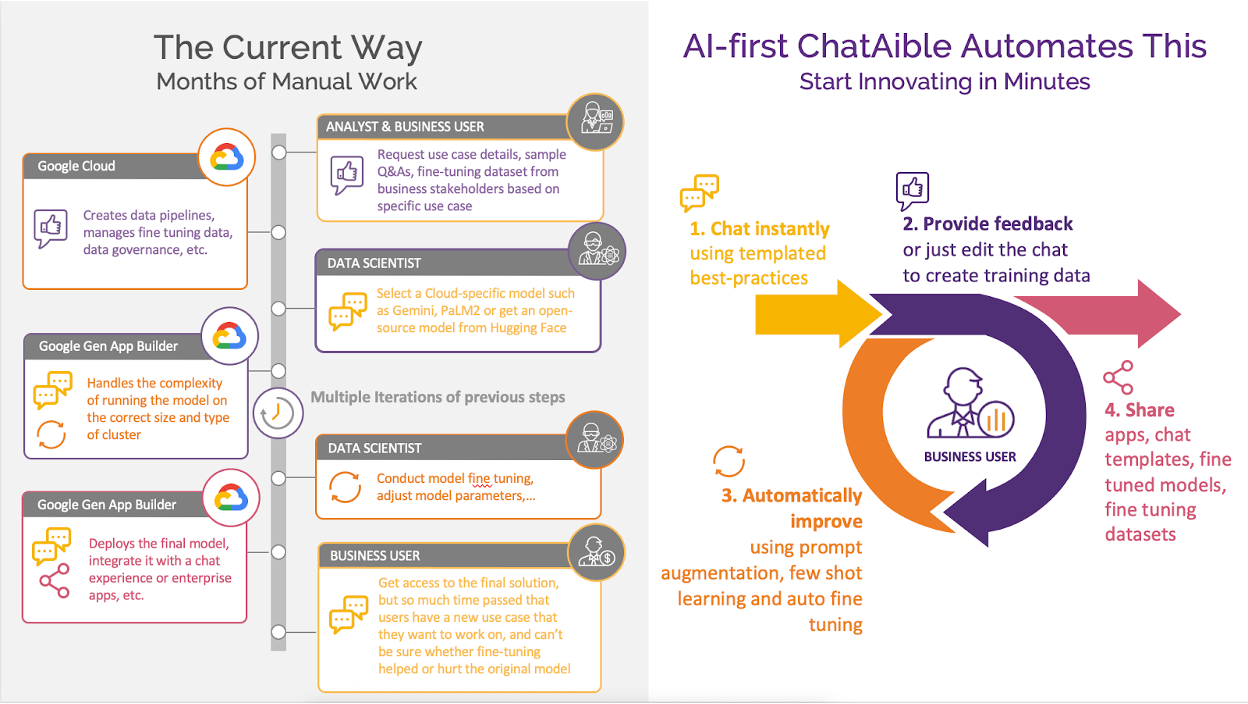
Aible worked with Google to iterate and discover the right Gemini and PaLM prompts for common prototype use cases, such as document summarization and question answering, and built them into Chat Templates. These templates automatically augment the actual user prompt to add in best practices. For example, you might choose an analytics template and simply ask, “How can I improve revenue?”
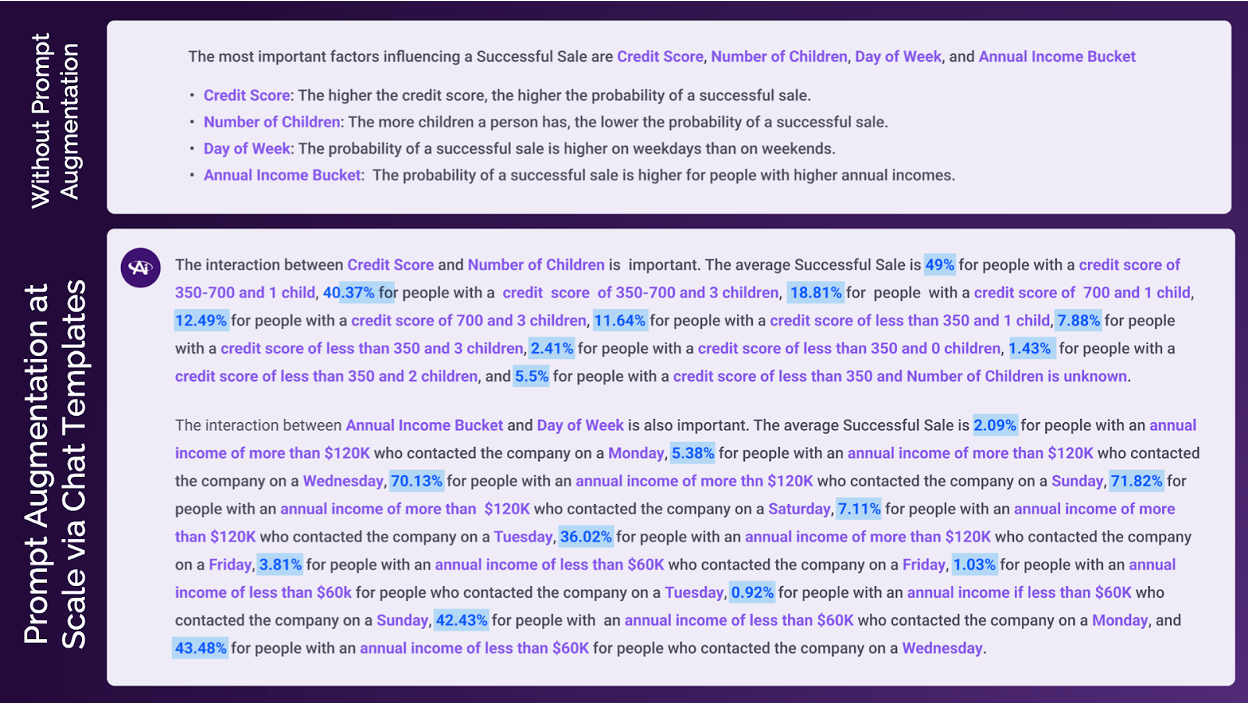
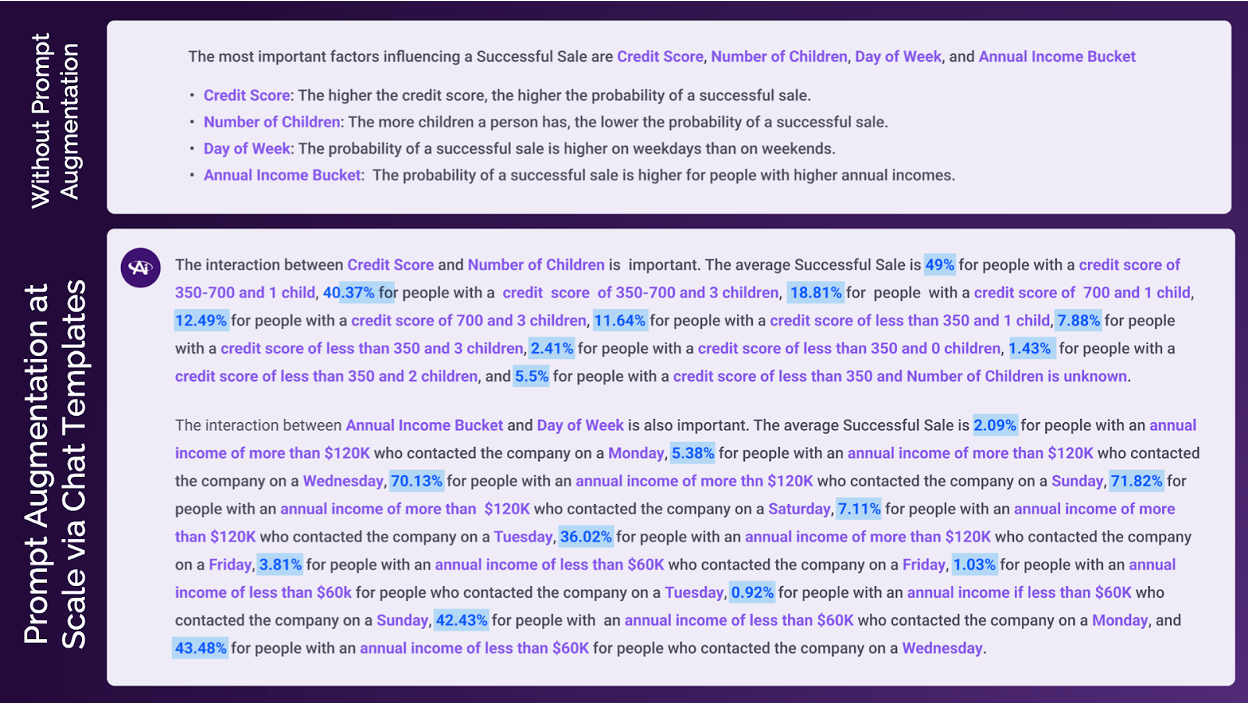
ChatAible detects existing enterprise data that can potentially help answer the question and analyzes the data with your permission or sends the data to VertexAI for summarization. It will then augment your original prompt, instructing the gen AI model to pay attention to the relevant data when it responds. ChatAible also applies model settings like temperature, according to best practices based on the model and use case. As different gen AI models evolve, Aible can simply update the Chat Templates, allowing all Aible customers to benefit instantly.
In addition, ChatAible also allows you to customize your personal preferences. For example, some users may prefer responses in bulleted list form while others prefer long-form descriptions. You can even choose to chat in a different language. The result is that ChatAible is personalized for each user, providing a better Day 1 experience that enables business users to get the most out of gen AI without having to go through extensive, targeted training.
1. Provide Feedback: Users are used to providing thumbs up / down feedback on ChatGPT or Gemini. They can do exactly the same thing with ChatAible and the feedback data is stored in the customer’s Google Cloud project so it can be later used to improve models. But, some kinds of model improvements require examples of bad and good chat responses. Such feedback typically has to be collected by Data Scientists from Business Users - usually manually using spreadsheets. In ChatAible, business users can simply edit the chat response into what they would have preferred the response to have been and the system stores the feedback data (along with any relevant Retrieval Augmented Generation context) in the customer’s Google Cloud project so it can be later used to improve models. This is a far simpler process for gathering business user feedback and the more simple you make this process, the more relevant examples you can gather for model improvement.
2. Automatically Improve: There are many ways to improve models such as reinforcement learning, fine tuning, data encoding, Low-Rank Adaptation (LoRA) etc. Each of these techniques require different amounts of data. Improvements from some of these techniques such as LoRa can be stacked. For example, if I developed a LoRA that understands comedy better, and you developed one that understood Spanish better, the two LoRA combined should understand Spanish comedy better. New approaches and best practices for improving models are being developed everyday. For business users this is too much complexity.
With ChatAible, users just specify whether they want the improvements to be stackable and ChatAible informs them how much more data they need to collect for different improvement techniques. Once they have sufficient data, they click a button and the model improvement is conducted using all of the capabilities of Vertex AI and the relevant Google tools for improving models.
Users can also edit the default prompt augmentations or model settings of the Chat Templates to see if they can improve the GenAI output. This is simple trial and error with immediate feedback under the control of the business users. Because Aible is end-to-end serverless, users can easily try different combinations of VectorDB settings, model settings, prompt augmentation, etc. in parallel as A-Z testing. Combinations with lower ratings, higher costs, higher latencies can then be pruned easily and user requests can be directed to better performing combinations.
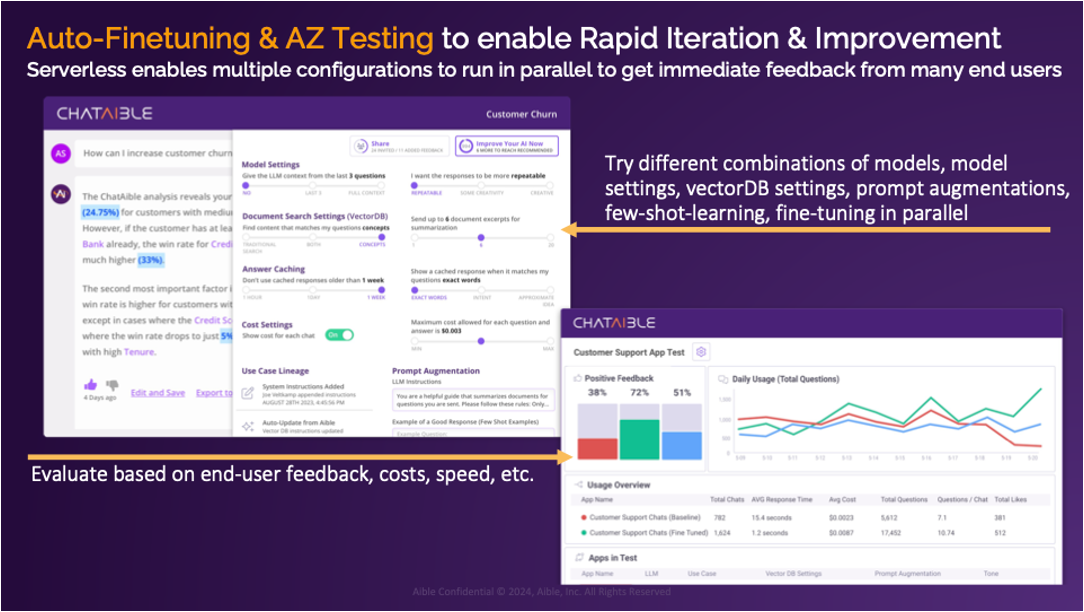
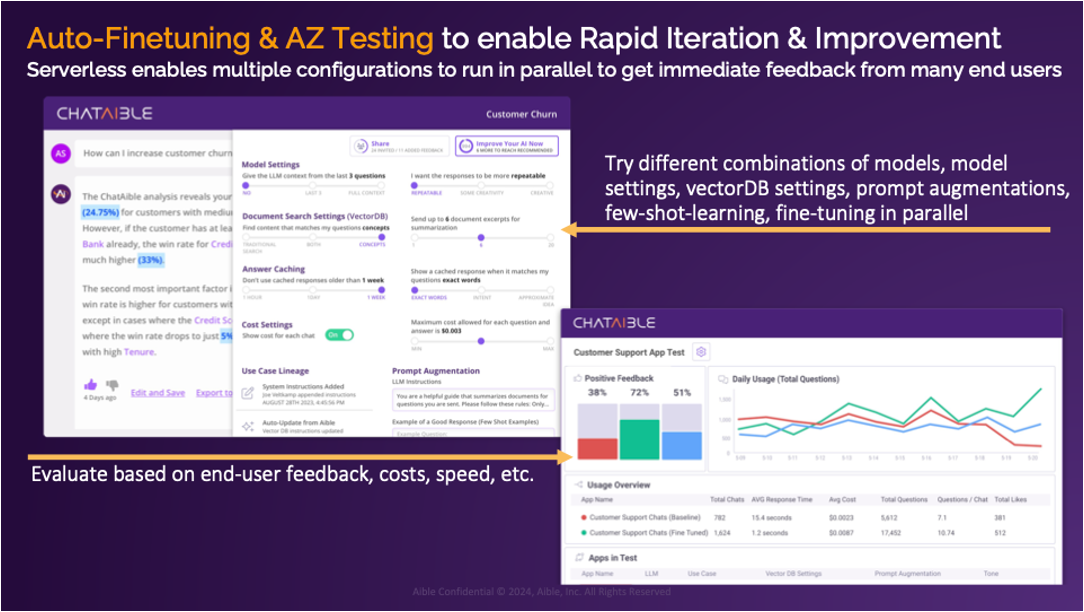
3. Secure: Organizations are concerned about users accidentally getting access to data that they are not authorized for through the fine-tuning process. Because of Aible’s LORA-stacking and serverless design, fine-tuning can be done based on feedback provided by users with specific roles and users can be restricted to fine-tuned models that were never trained on data that the users would not have had access to. Roles with higher privilege levels can then use models that stack LORA trained on feedback from users at lower permission levels. This way organizations can ensure that fine-tuned models don’t accidentally breach their data security expectations.
4. Share: Today business users can share individual chats and collaborate on the chats. They can also share model improvements or updated Chat Templates with other users in their organization. Aible’s services partners can share Chat Templates and updated models with their customers (with appropriate shared-customer permissions). Eventually, users will also be able to share chat templates and model improvements across organizations.
Scaling business use cases
Gen AI will be transformative for your business. The faster you get large numbers of people across your organization safely experimenting with it, the faster you can gain competitive advantage from it. As more teams identify use cases, it’s likely you will need an easy way to manage and scale gen AI across your organization.
Leveraging Google Cloud technologies, which are built for scale, Aible makes it easy to adapt and scale gen AI use cases and gen AI applications for wider adoption. For example, ChatAible’s Chat Templates make it easy to fine-tune and improve gen AI models, allowing you to easily change chat context according to new use cases simply by changing your templates. This allows you to provide a personalized, consistent experience for many different types of users across multiple gen AI use cases and enterprise applications.
The proof of the pudding is truly in how quickly organizations can prototype new gen AI solutions. For example, multiple joint customers presented at the Gartner Data and Analytics Summit, sharing how they built gen AI solutions in a matter of days with Aible. Given recent estimates that as much as 30% of gen AI proof of concepts will be abandoned by 2025, there is an even greater need than ever for rapid implementation and iteration. A failure that is encountered in a matter of days is never a catastrophe, merely a lesson learnt on the way to gen AI success.
Gen AI success from day 1
Aible sets organizations up for success, empowering all users — including business users — to quickly get started on their generative AI use cases. Aible’s capabilities (backed and secured by Google Cloud technologies), such as templated best practices, prompt augmentation at scale, hallucination double-checking, and more ensure business users have a productive and safe experience from the moment they start using gen AI. This kind of bottoms-up, business-user-led innovation is key to realizing rapid gen AI value at scale.
Learn more about Google Cloud’s open and innovative generative AI partner ecosystem. To get started with Aible on Google Cloud, check out the solution on Marketplace.