Celebrating 20 years of Bigtable with exciting announcements at Next
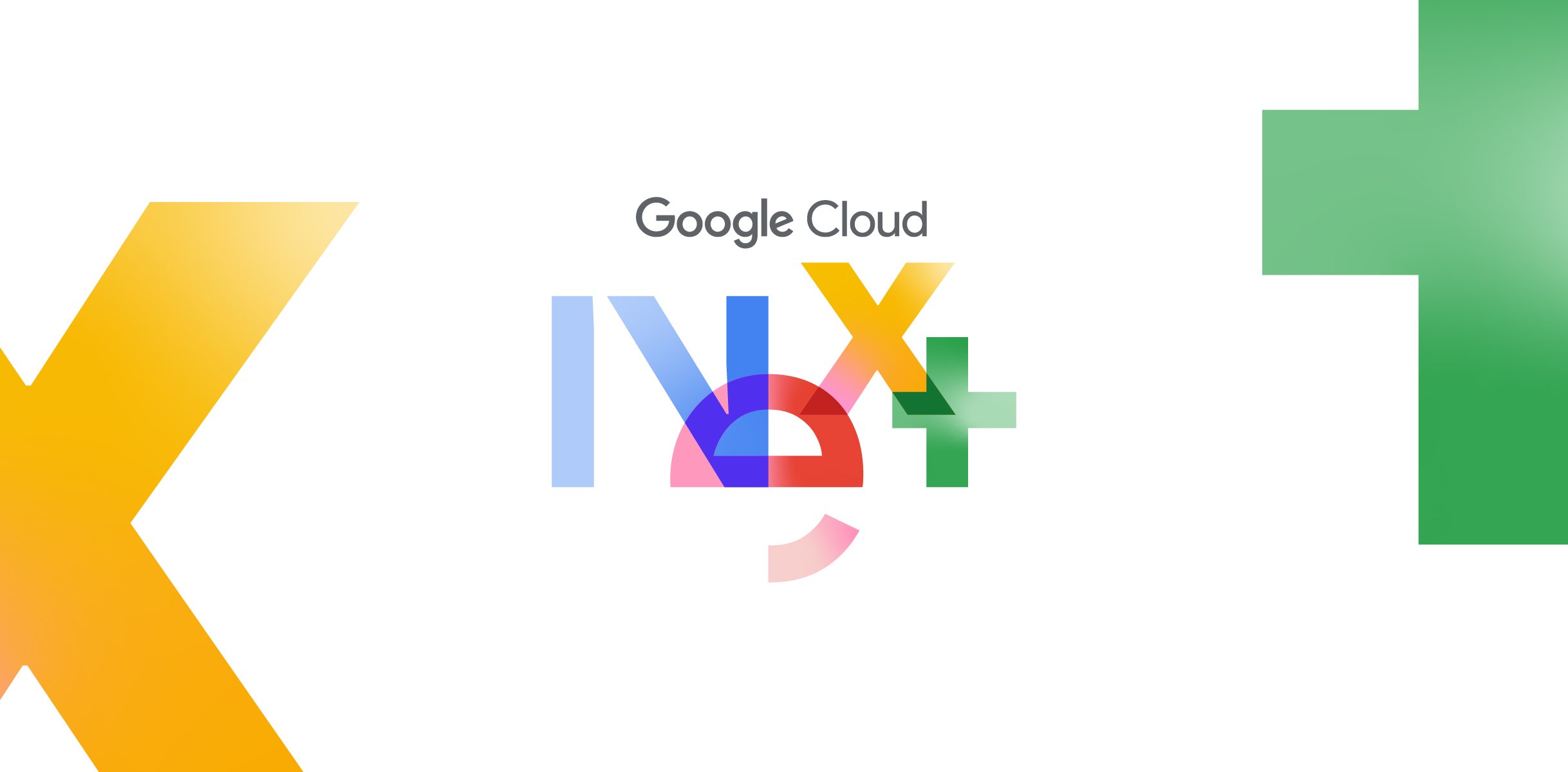
Bora Beran
Group Product Manager, Bigtable
How do you store the entire internet? That’s the problem our engineering team set out to solve in 2004 when it launched Bigtable, one of Google’s longest serving and largest data storage systems. As the internet — and Google — grew, we needed a new breed of storage solution to reliably handle millions of requests a second to store the ever-changing internet. And when we revealed its design to the world in a 2006 research paper, Bigtable kicked-off the Big Data revolution, inspiring the database architectures for NoSQL systems such as Apache HBase and Cassandra.
Twenty years later, Bigtable doesn’t just support Google Search, but also latency-sensitive workloads across Google such as Ads, Drive, Analytics, Maps, and YouTube. On Google Cloud, big names like Snap, Spotify and Shopify rely on Bigtable, now serving a whopping 7 billion queries per second at peak. On any given day, it is nearly impossible to use the internet without interacting with a Bigtable database.
Bigtable isn’t just for Big Tech, though. This year, our goal is to bring Bigtable to a much broader developer audience and range of use cases, starting with a number of capabilities that we announced this week at Google Cloud Next.
Introducing Bigtable Data Boost and Authorized Views
For one, Bigtable now supports Data Boost, a serverless way for users to perform analytical queries on their transactional data without impacting their operational workloads. Currently in preview, Data Boost makes managing multiple copies of data for serving and analytics a thing of the past. Further, Data Boost supports a requester-pays model, billing data consumers directly for their usage — a unique capability for an operational database.
Then, new Bigtable Authorized Views enable many data sharing and collaboration scenarios. For example, retailers can securely share sales or inventory data with each of their vendors, so they can more accurately forecast demand and resupply the shelves — without worrying about how much server capacity to provision. This type of use case is quite common for organizations with multiple business units, but sharing this level of data has traditionally required keeping copies of data in multiple databases, building custom application layers and billing components. Instead, with Bigtable Authorized Views and Data Boost, each vendor will get their own bill for the amount of data they process, with no negative impact on retailer’s operations. Bigtable Authorized Views make it easier to serve data from a single source of truth, with improved data governance and quality.
These features, along with the existing Request Priorities, stand to transform Bigtable into an all-purpose data fabric, or a Digital Integration Hub. Many Google Cloud customers already use Bigtable for their data fabrics, where its strong write performance, horizontal scalability and flexible schema make it an ideal platform for projects that ingest large amounts of data in batch from multiple sources or collate real-time streaming events. But businesses and their data evolve over time. New data sources are added through acquisitions, partnerships, new product launches, additional business metrics and ML features. To get the value out of data, you need to combine all the pieces and see the big picture — and do it in real-time. Bigtable has already solved the latency and database scaling problems, but features like Authorized Views and Data Boost help to solve data and resource governance issues.
During the preview, Data Boost is offered at no cost.
Boosting Bigtable performance for next-gen workloads
At Next, we also announced several Bigtable price-performance improvements. Bigtable now offers a new aggregate data type optimized for increment operations, which delivers significantly higher throughput and can be used to implement distributed counters and simplify Lambda architectures. You can also choose large nodes that offer more performance stability at higher server utilization rates, to better support spiky workloads. This is the first of workload-optimized node shapes that Bigtable will offer. All of these changes come on the heels of an increase in point-read throughput from 10K to 14K reads per second per node just a few months ago. Overall, these improvements mean lower TCO for a database already known for its price-performance.
These improvements could help power your modern analytics and machine learning (ML) workloads: ML is going real-time, and models are getting larger, with more and more variables that require flexible schemas and wide data structures. Analytics workloads are also moving towards wide-table designs with the so-called one big table (OBT) data model. Whether you need its flexible data model for very wide, gradually evolving tables; its scalable counters’ ability to provide real-time metrics at scale, or features like Data Boost and Request Priorities that allow seamless backfills and frequent model training (thereby combining real-time serving and batch processing into a single database), Bigtable simplifies the ML stack and reduces concept and data drift, uplifting ML model performance.
With 20 years of learnings from running one of the world’s largest cloud databases, Bigtable is ready to tackle even more demanding workloads. If you’re at Google Cloud Next, stop by the following sessions to learn how Ford uses Bigtable for its vehicle telemetry platform, how Snap uses it their latency-sensitive workloads, how Shopify uses Bigtable to power its recommendation system, and about Palo Alto Networks’ journey from Apache Cassandra to Bigtable.