Delivering greater insights for insurance underwriters with BigQuery geospatial analytics
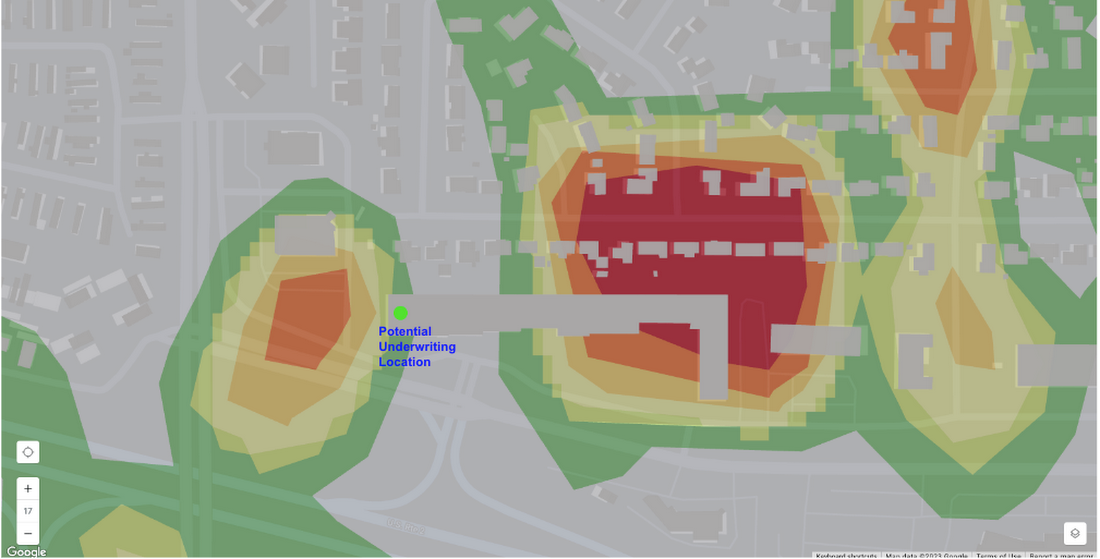
Gaganpreet Randhawa
AVP Enterprise Architecture - Data Analytics, CNA
Damian Graham
Principal Architect, Google Cloud
About CNA
A key goal for insurers is to provide the best match between customer need, product offering and the premium charged. However, the price charged and eventual profitability is partially dependent on how well the insurer understands and assesses the risk being insured. This is usually a time-consuming and often subjective process that relies on the unique skills of the individual underwriter along with their ability to capture, analyze, and interpret vast amounts of associated data points.
CNA is one of the largest commercial property and casualty insurance companies in the U.S. With over 120 years of experience, CNA provides a broad range of standard and specialized insurance products and services for businesses and professionals in the United States, Canada and Europe. Over the last three years, CNA has been building its data-analytics foundation on Google’s Data Cloud. This has been accomplished by consolidating hundreds of global data sources, leveraging Vertex AI automation to accelerate time-to-market for machine learning models, and delivering a series of executive reporting dashboards on key business performance metrics.
Challenge: Underwriting flood risk for commercial properties
With the building of their analytics foundation complete, CNA has increasingly focused their attention on providing value-added analytic products at scale to their business units, specifically, to enhance the underwriting experience by using advanced analytics technologies to deliver more robust insights within the underwriting ecosystem. This will enable underwriters to quickly and more accurately evaluate property risk and perform additional analysis to better understand the implications of various decisions and provide quality insurance products.
Effectively assessing underwriting flood risk was a priority area for CNA. This is a difficult process that must take into consideration a couple key factors:
Flood hazards events are multidimensional in nature, with threats coming from coastal surges, fluvial (river), and pluvial (surface) sources. Coastal and fluvial risks are directly related to their proximity to water bodies. However, surface flooding is often spontaneous and typically occurs when intense rainfall or surface run-off overwhelms an urban drainage system. Pluvial flooding is often difficult to predict and urban areas are particularly vulnerable and have high levels of exposure to this hazard event.
Flood hazards require a comprehensive understanding of the complex spatial relationships that exist between the commercial property being insured and its surrounding environment. The ability to identify any associated risk due to adjacent property location is also important.
“We needed a way to improve how we assessed and understood flood risk. Shifting to a process that incorporates more robust floodplain data sources and applying geospatial analytics technologies to better understand the complex spatial relationships that exist is critical to providing underwriters with the accurate and timely insights that they need.” - Tom Stone, VP Aggregation and Catastrophe Management, CNA |
CNA’s current process for assessing flood risk had limitations across the following areas:
Limited data coverage: The data used for analysis had gaps and only took into consideration fluvial and coastal water sources. Pluvial sources that accounted for two thirds of flood losses were not a part of the risk assessment process.
Limited geographic extent: The process relied on a geocoded point location (property address) that did not capture the geographic extent of the entire site. The impact of the hazard on the entire site or even adjacent ones was challenging.
Challenge with associated risk: Geocoded point locations could be anywhere on a property. These locations may intersect with existing floodplain data. However, in the absence of a precise intersection, the actual proximity of a property to a floodplain (close by, near to) could not be assessed.
Solution: Powering flood risk assessment with BigQuery geospatial analytics
To solve this challenge CNA worked with Google Cloud and several third-party data vendors to develop a more sophisticated solution that addressed the current challenges with underwriting flood risk assessment
Floods and their associated impacts have a strong location component and geospatial analytics that uses location data (latitude, longitude) to better understand spatial relationships and patterns is the foundation of the solution. Improving the accuracy of geospatial analysis requires access to comprehensive data. CNA acquired several datasets on the natural and anthropogenic environment, including buildings, flood risk (coastal, fluvial and pluvial), land parcels, and more.
These were large datasets that had both national and global geographic coverage. Because of this, CNA needed a data analytics solution that could store, process, and perform large-scale and computationally expensive geospatial analytics to better understand the complex relationships that exist between these datasets and the combined impact to both their current and future portfolio of business.
“Over the years we have worked with Google Cloud to accelerate our data journey. BigQuery geospatial analytics was a natural fit for this flood risk assessment challenge that requires robust data management, powerful geospatial analytics functionality, and extensive computational scale to quickly analyze these large datasets.” - Dr. Pierre Braganza, Chief Enterprise Architect, CNA
Based on previous strategic investments, CNA was in a unique position to solve this challenge. They had already achieved data sufficiency at scale with 90% of their data residing in a highly governed data warehouse built on BigQuery. BigQuery has the added benefit of possessing powerful capabilities for both geospatial data management and geospatial analytics. Using BigQuery for geospatial analytics lets you analyze geographic data using standard SQL geography functions. As these functions execute they harness the compute power of BigQuery, which makes this an ideal platform for rapidly analyzing the complex spatial relationships that exist within and between the datasets.
Building an intelligent geospatial flood risk API
The solution uses Dataflow and geobeam to land terabytes of geospatial data in raster and vector formats into BigQuery. Underpinning the solution is an intelligent API built on Google Kubernetes Engine that dynamically executes several BigQuery geospatial analytics functions to explore the spatial relationships between a potential underwriting location (latitude, longitude), the underlying associated parcel, the geographic extent of the related buildings, and their intersection with the floodplain. A multilevel flood risk score is automatically generated based on the locations’ spatial intersection (ST_Intersects) or proximity (ST_Buffer) to the floodplain zones, parcels and associated buildings.
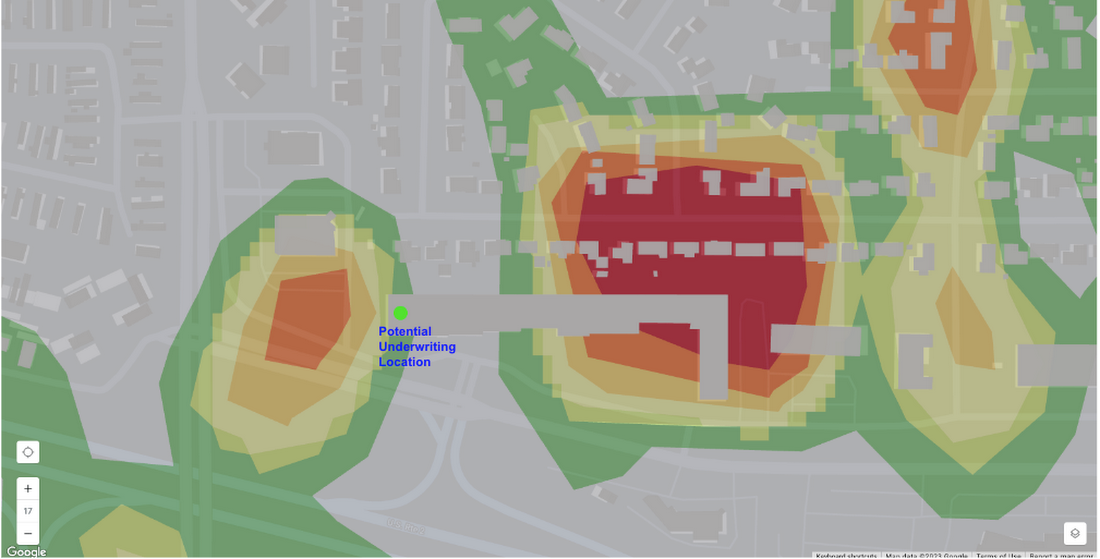
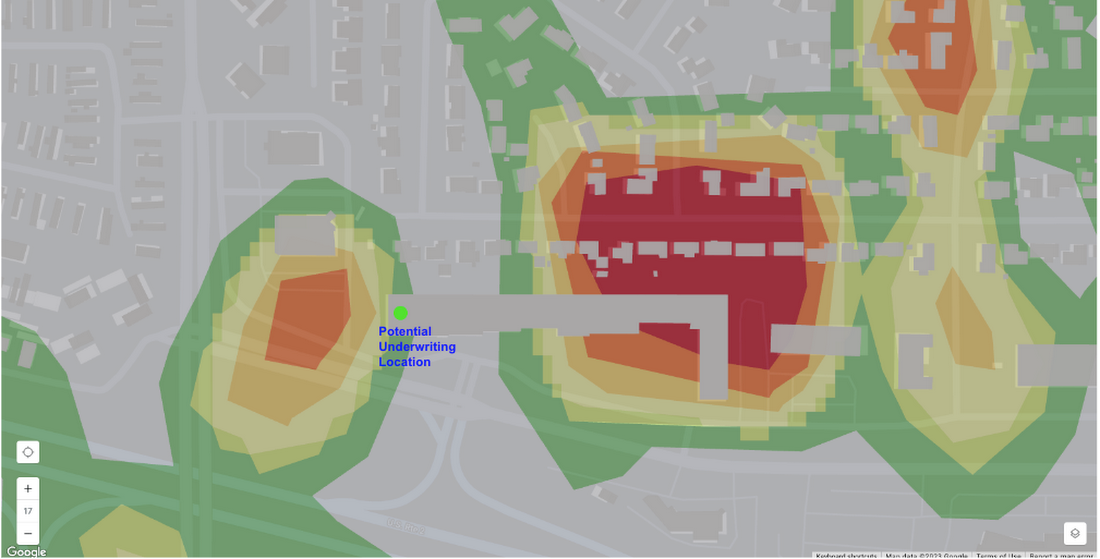
This will be a significant improvement over the old process.
“Previously when we geocoded a property address (latitude, longitude) it could be found anywhere on a property, which may not overlay with the floodplain. Now we have an intelligent API that assesses risk at multiple levels based on the geographic extent of the entire property.” - Tom Stone
CNA can now understand flood hazards that affect any portion of a property as opposed to a single point location. This is of particular importance in instances where an insured location may be found on a site that has a geographic scope that crosses multiple floodplain zones. In such a scenario, a seemingly low-risk property could be determined to be more high risk based on this spatial relationship.
The API is being integrated with CNA’s underwriting system and related business data. During the underwriting process, underwriters will automatically be provided with the various risk scores so more informed decisions can be made about the commercial property to be insured. The API will also allow CNA to analyze its entire business portfolio to better understand flood risk.
Results: Improved risk decisions with geospatial technology
CNA’s underwriting flood risk solution will drive improved insights for underwriters, which should provide numerous benefits:
Underwriters are empowered with new functionality that quickly and accurately alerts them to locations on a property schedule that are susceptible to flooding while allowing for further analysis on potential implications.
Through the solution, CNA will gain a more complete flood risk dataset that includes pluvial risk information on which to make more informed decisions. This should help reduce losses that are occurring based on inadequate pricing for this type of hazard.
CNA will better understand the relationship between a location and the associated property, buildings and floodplain zones to which it belongs. This is critical for being able to classify both the direct and associated risks for a property that may span multiple floodplain zones. Preliminary analysis has shown an approximate 25% increase in the ability to provide a comprehensive view of flood risk.
In the future, CNA plans to build additional geospatial visualization capabilities to allow for further exploration. The solution is designed to incorporate additional hazard types as business needs arise. CNA also has the ability to quickly perform a scenario-based analysis on an entire portfolio of business and its susceptibility to flood risk.
By leveraging BigQuery for geospatial analytics, CNA tackled the spatial problem of being able to better understand and measure flood risk. With 90% of all data possessing a location component, geospatial analytics can be applied to other business areas and problem sets.