Simplifying MLOps using Weights & Biases with Google Kubernetes Engine
Jason Zhao
Director of Engineering, Weights & Biases
Nathan Beach
Group Product Manager, Google Kubernetes Engine
The recent boom in the AI landscape has seen larger and more complex models give rise to mind-blowing AI capabilities across a range of applications. At the same time, these larger models are driving up costs for AI compute astronomically; state-of-the-art LLMs cost tens of millions of dollars (or more) to train, with hundreds of billions of parameters and trillions of tokens of data to learn.
ML teams need access to compute that is both scalable and price-efficient. They need the right infrastructure to operationalize ML activities and enhance developer productivity when working with large models. Moreover, they must maintain guardrails for orchestration and deployment to production.
Developing, refining, optimizing, deploying, and monitoring ML models can be challenging and complex in the current AI landscape. However, the efficient orchestration, cost-effective performance, and scalability present in Google Kubernetes Engine (GKE), in tandem with Weights & Biases (W&B) Launch's user-friendly interface, simplifies the model development and deployment process for machine learning researchers. This integration seamlessly connects ML researchers to their training and inference infrastructure, making the management and deployment of machine learning models easier.
In this blog, we show you how to use W&B Launch to set up access to either GPUs or Cloud Tensor Processing Units (TPUs) on GKE once, and from then easily grant ML researchers frictionless access to compute.
W&B Launch
W&B is an ML developer platform designed to enable ML teams to build, track, and deploy better models faster. As the system of record for ML activities, from experiment tracking to model registry management, W&B improves collaboration, boosts productivity, and overall helps simplify the complexity of modern ML workflows.
W&B Launch connects ML practitioners to their cloud compute infrastructure. After a one-time configuration by an ML platform team, ML researchers can then select the target environment in which they want to launch training or inference jobs.
W&B Launch automatically packages up all the code and dependencies for that job and sends it to the target environment, taking advantage of more powerful compute or parallelization to execute jobs faster and at greater scale. With jobs packaged up, practitioners can easily rerun jobs with small tweaks, such as changing hyperparameters or training datasets. ML teams also use W&B Launch to automate model evaluation and deployment workflows to manage shared compute resources more efficiently.
“We’re using W&B Launch to enable easy access to compute resources to dramatically scale our training workloads,” said Mike Seddon, Head of Machine Learning and Artificial Intelligence at VisualCortex. “Having that ability to create queues to each cluster and activate them is exactly what we want to do.”
Creating a GKE Cluster
GKE offers a fully-managed environment for deploying, managing, and scaling containerized applications using Kubernetes. ML teams often choose GKE over managing an open-source Kubernetes cluster because it provides the industry's only fully managed Kubernetes with a 99.9% Pod-level SLA backed by Google SREs, which reduces operational overhead and can improve an organization's security posture.
To start using W&B Launch with GKE, create a GKE cluster with TPUs or with GPUs
W&B Launch Jobs with GKE
The W&B Launch agent builds container images inside of GKE, capturing all the dependencies for that particular run.
Once W&B Launch is configured with the GKE cluster, ML engineers can easily start training jobs by accessing powerful GPUs or Google Cloud TPUs to accelerate and supercharge AI development.
To get started, create an account, install W&B and start tracking your machine learning experiments in minutes. You can then set up your W&B Launch queue and W&B Launch agent on your GKE cluster.
W&B Launch queues must be configured to point to a specific target resource, along with any additional configuration specific to that resource. A Launch queue that points to a GKE cluster would include environment variables or set a custom namespace for its Launch queue configuration. When an agent receives a job from a queue, it also receives the queue configuration.
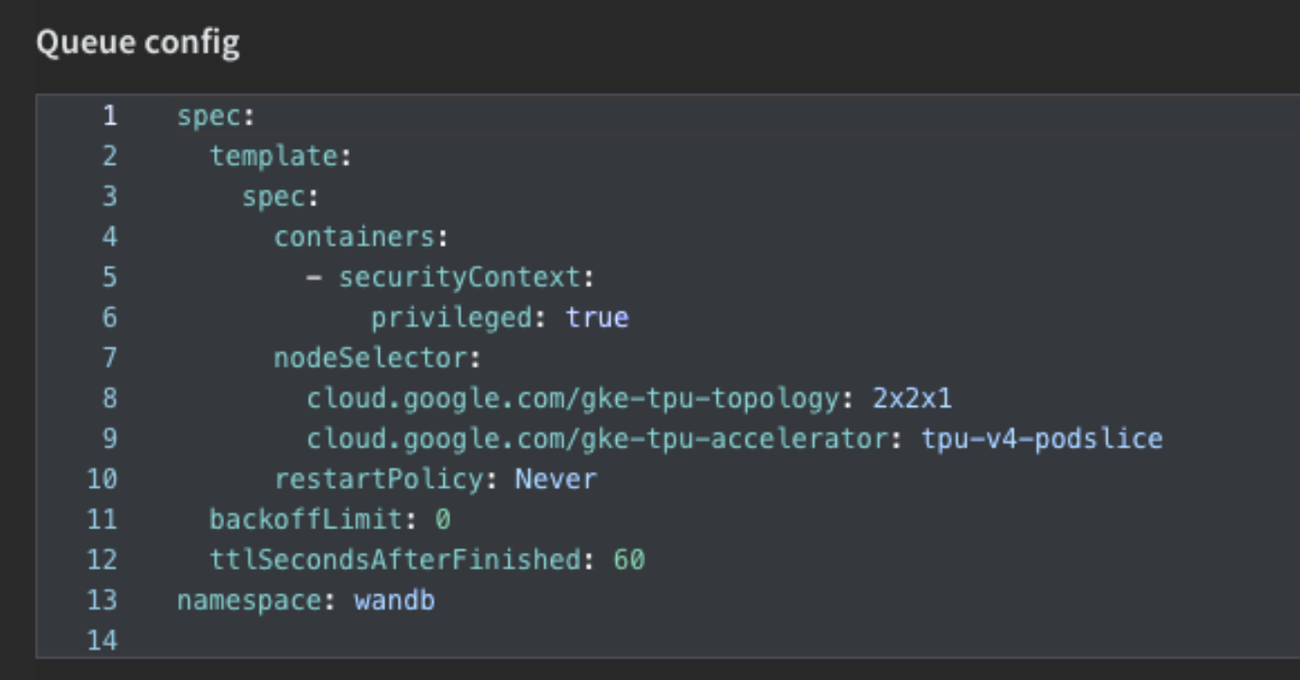
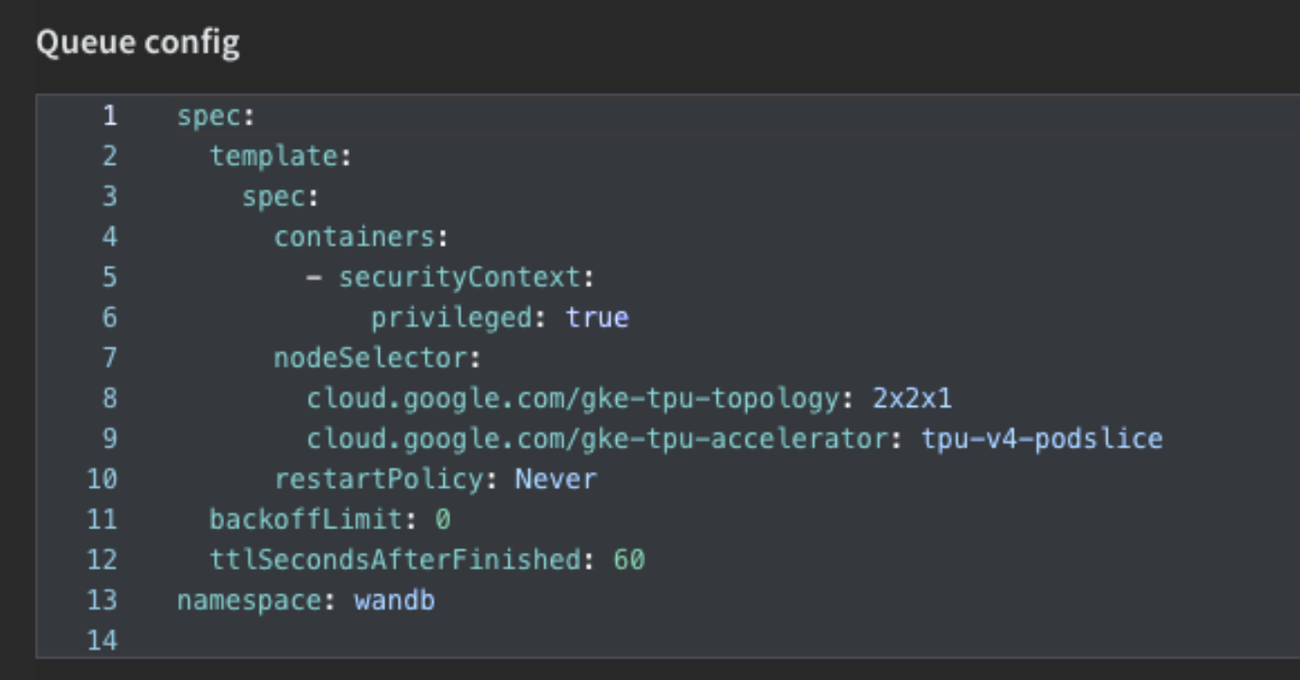
Once you’ve created your queue, you can set up your W&B Launch agent, which are long running processes that poll one or more W&B Launch queues for jobs, in a first in first out (FIFO) order. The agent then submits the job to the target resource — your Cloud TPU nodes within your GKE cluster — along with the configuration options specified.
Check out our documentation for more information on setting up your GKE cluster and agent.
Creating a W&B Launch job
Now that W&B Launch is set up with GKE, job execution can be handled through the W&B UI.
- Identify the previously executed training run that has been tracked in W&B.
- Select the specific code version used for the job under the version history tab.
- You will see the W&B Launch button on the upper right hand corner of the Python source screen.
- After clicking on the W&B Launch button, you’ll be able to change any parameters for the experiment, and select the GKE environment under the “Queue” menu.
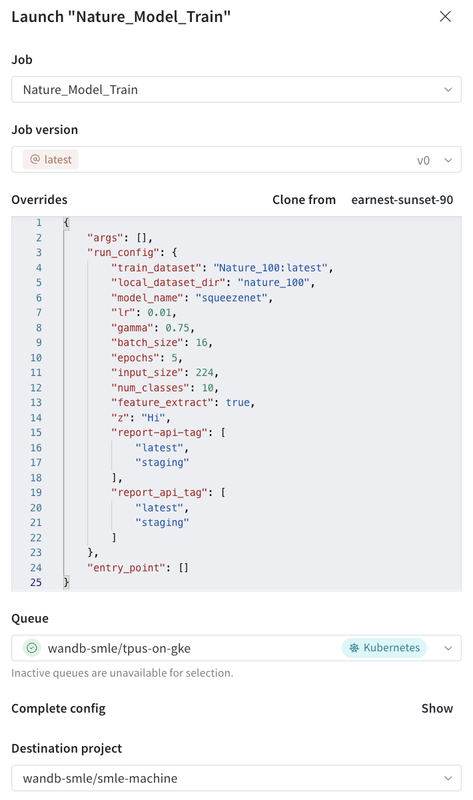
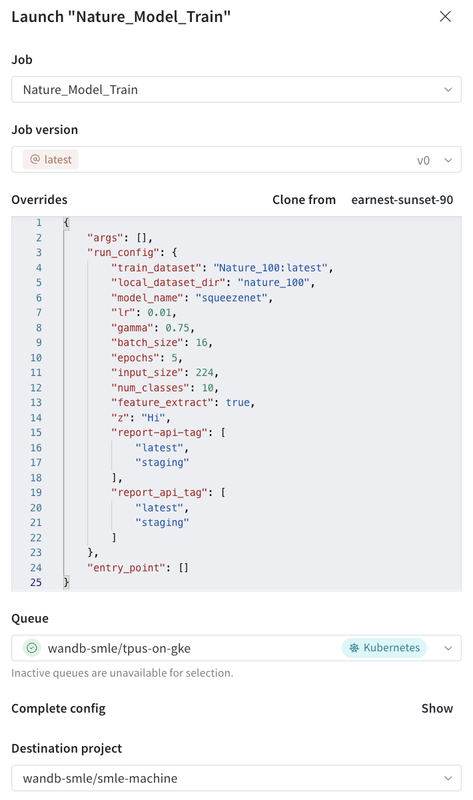
A common use case for W&B Launch is to execute a number of hyperparameter tuning jobs in parallel. Setting up a hyperparameter sweep is simple: select “Sweep” on the left-hand toolbar, enter the range of the sweep for the hyperparameters, and select the “GKE” queue for the environment.
Conclusion
W&B Launch with GKE is a powerful combination to provide ML researchers and ML platform teams the compute resources and automation they need to rapidly increase the rate of experimentation for AI projects. To learn more, check out the full W&B Launch documentation and this repository of pre-built W&B Launch jobs.