Your guide to Generative AI support in Vertex AI
Warren Barkley
Sr. Director of Product Management
Okay, so I initially suggested that I deliver the content of this blog as an interpretive dance video. My suggestion was turned down, and I'm sure you’re as disappointed as I am. But dancing or not, I’m really excited about Generative AI support in Vertex AI.
Vertex AI was launched in 2021 to help fast-track ML model development and deployment, from feature engineering to model training to low-latency inference, all with enterprise governance and monitoring. Since then, customers like Wayfair, Vodafone, Twitter, and CNA have accelerated their ML projects with Vertex AI, and we’ve released hundreds of new features.
But we didn’t stop there — Vertex AI recently had its biggest update yet. Generative AI support in Vertex AI offers the simplest way for teams to take advantage of an array of generative models. Now it’s possible to harness the full power of generative AI built directly in our end-to-end machine learning platform.
Generative AI support in Vertex AI
In the last few months, consumer-grade generative AI has captured the attention of millions, with intelligent chatbots and lifelike digital avatars. Realizing the potential of this technology means putting it in the hands of every developer, business, and government. To date, it’s been difficult to access generative AI and customize foundation models for business use cases because managing these large models in production is a difficult task, requiring an advanced toolkit, lots of data, specialized skills, and even more time.
Generative AI support in Vertex AI makes it easier for developers and data scientists to access, customize, and deploy foundation models from a simple user interface. We provide a wide range of tools, automated workflows, and starting points. Once deployed, foundation models can be scaled, managed, and governed in production using Vertex AI’s end-to-end MLOps capabilities and fully-managed AI infrastructure.
Vertex AI recently added two new buckets of features: Model Garden, and Generative AI Studio. In this blog, we dive deeper into these features and explore what’s possible.
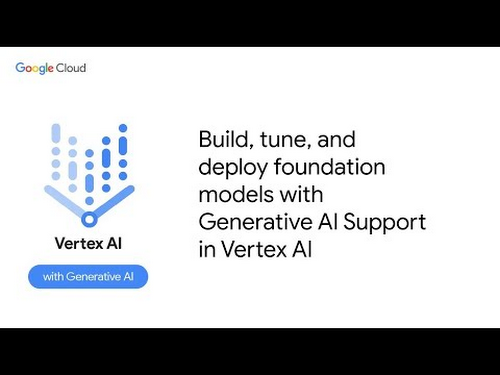
Model Garden: Discover and use the widest variety of model types available
Model Garden provides a single environment to search, discover, and interact with Google’s own foundation models, and in time, hundreds of open-source and third-party models. Users will have access to more than just text models — they will be able to build next-generation applications with access to multimodal models from Google across vision, dialog, code generation, and code completion. We’re committed to providing choice at every level of the AI stack, which is why Model Garden will include models from both open-source partners and our ecosystem of AI partners. With a wide variety of model types and sizes available in one place, our customers will have the flexibility to use the best resource for their business needs.
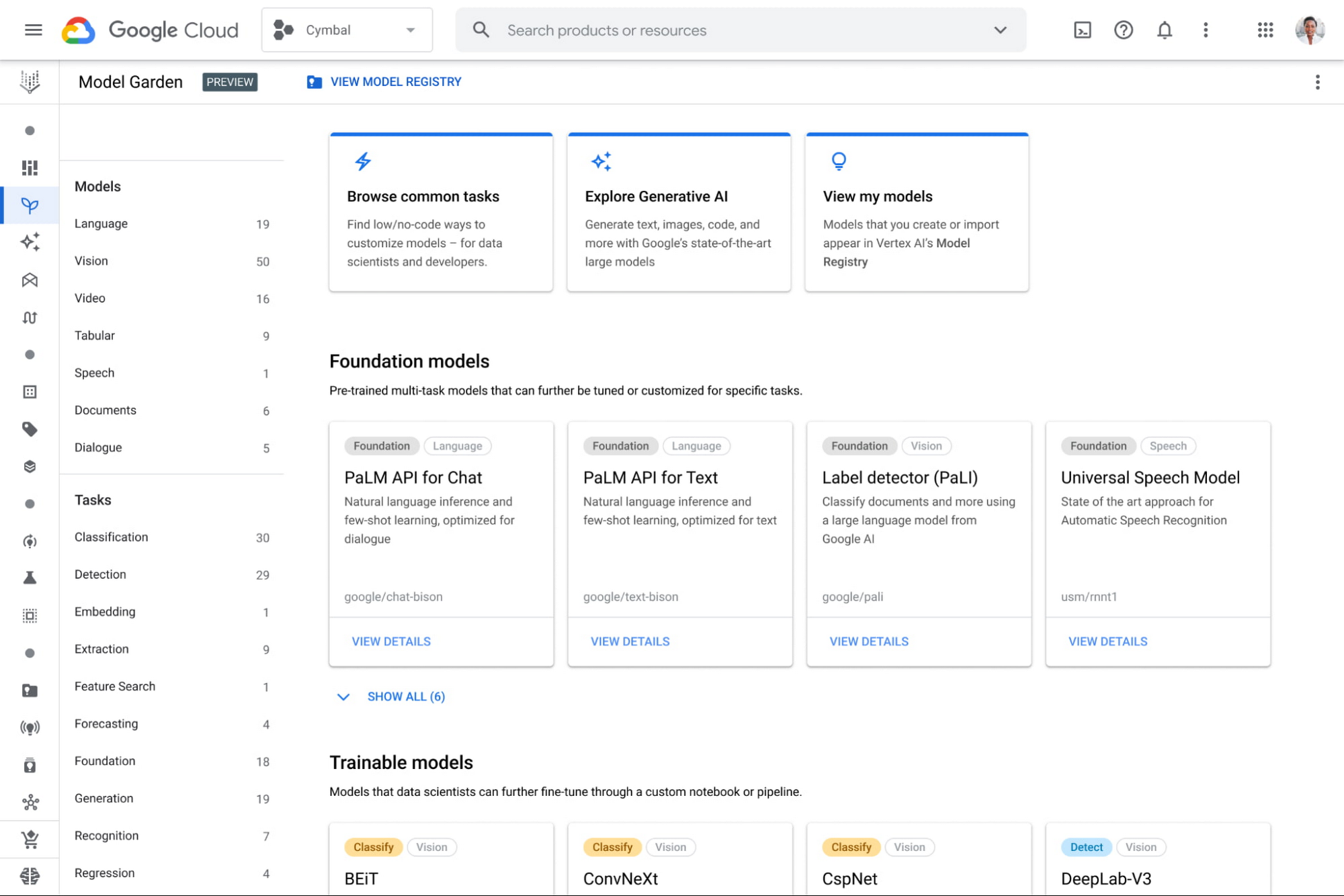
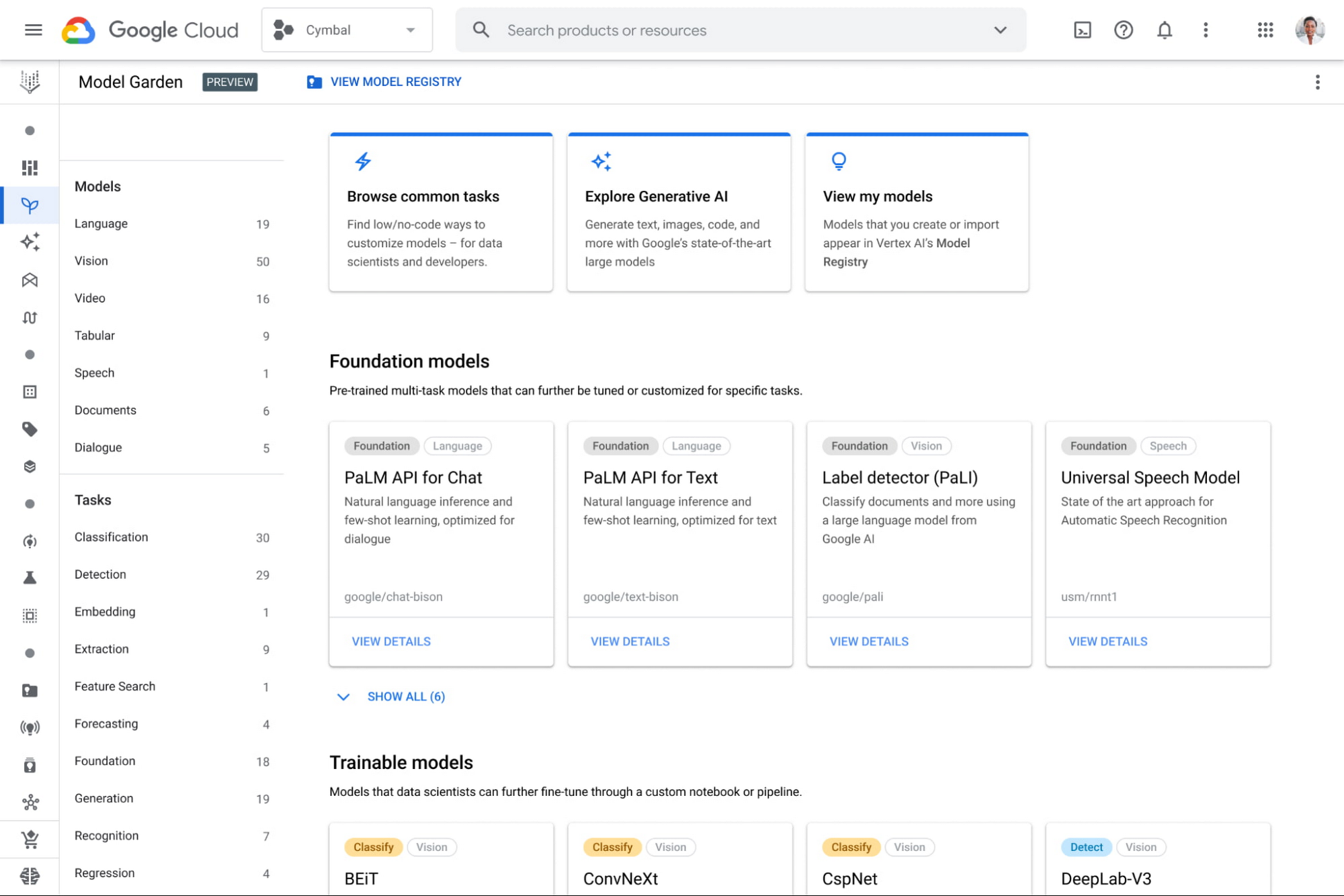
From Model Garden, users can kick off a variety of workflows, including using the model directly as an API, tuning the model in Generative AI Studio, or deploying the model directly to a data science notebook in Vertex AI.
Generative AI Studio: Easily tune and deploy foundation models
Generative AI Studio is a managed environment in Vertex AI where developers and data scientists can interact with, tune, and deploy foundation models. Generative AI Studio provides a wide range of capabilities including a chat interface, prompt design, prompt tuning, and even the ability to fine-tune model weights. From Generative AI Studio, users can implement newly-tuned models directly into their applications or deploy models to production on Vertex AI’s ML platform. With both tools that help application developers and data scientists contribute to building generative AI, organizations can bring the next generation of applications to production faster, and with more confidence.
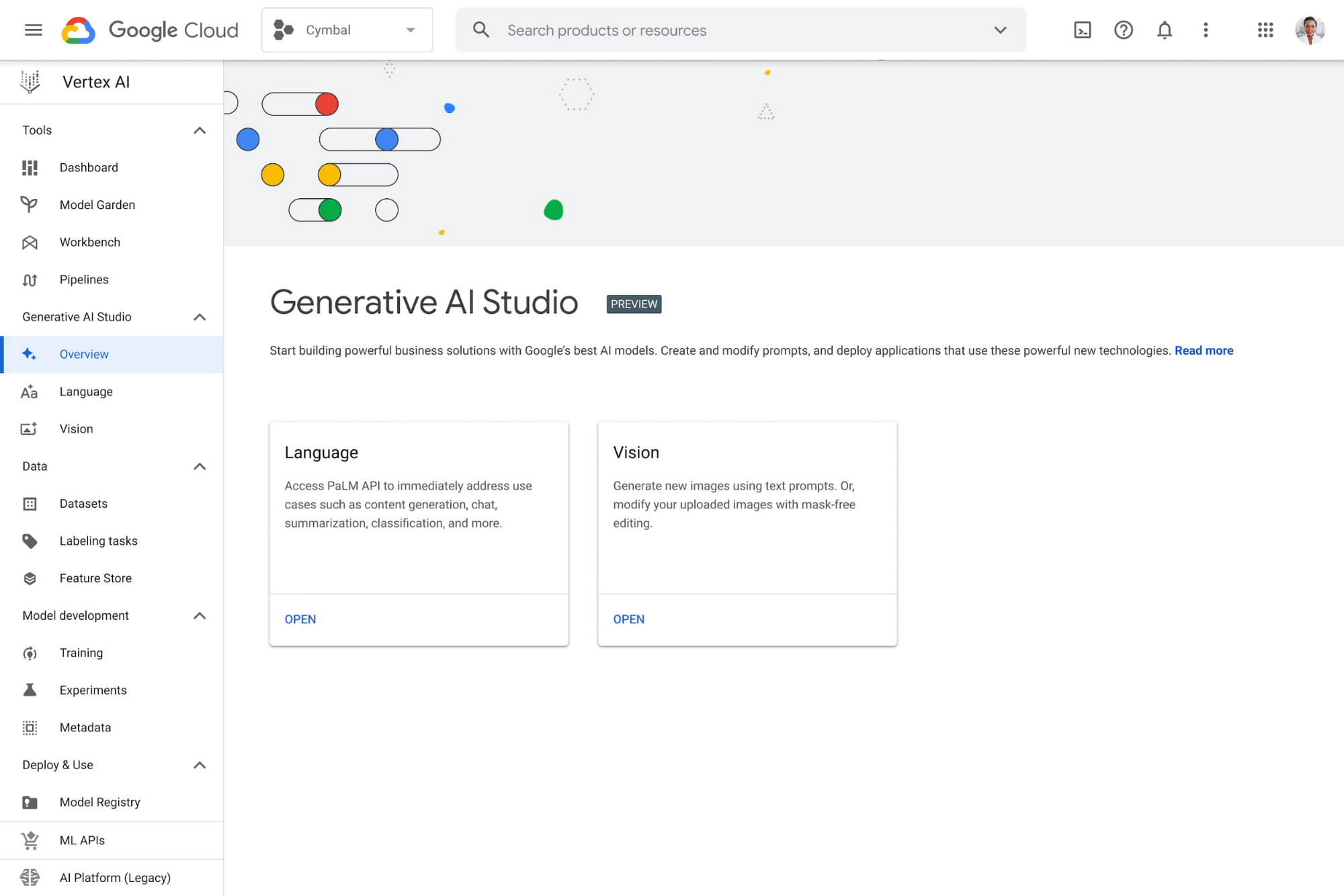
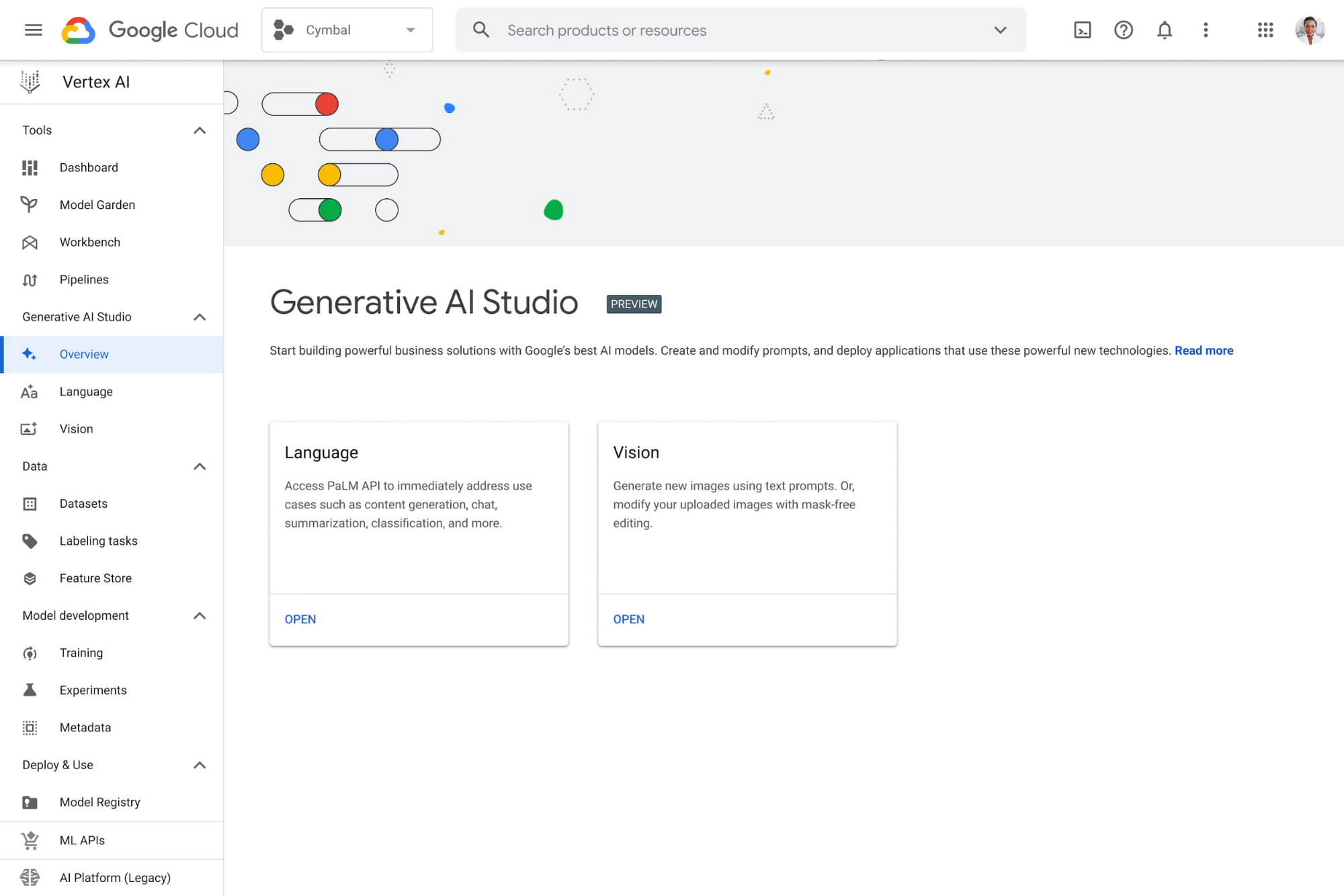
5 ways to interact with foundation models in Vertex AI
1. Use foundation models as APIs: We’re making Google’s foundation models available to use as APIs, including text, dialogue, code generation and completion, image generation, and embeddings. Vertex AI's managed endpoints make it easy to build generative capabilities into an application, requiring only a few lines of code, just like any other Google Cloud API. Developers do not need to worry about the complexities of provisioning storage and compute resources, or optimizing the model for inference.
2. Prompt design: Generative AI Studio provides an easy-to-use interface for prompt design, which is the process of manually creating text inputs, or prompts, that inform a foundation model. The familiar chat-like experience enables people without developer expertise to interact with a model. Users can also configure the system well beyond the chat interface. For example, they can control the temperature of responses, which means they can control whether the responses have higher accuracy or higher creativity.
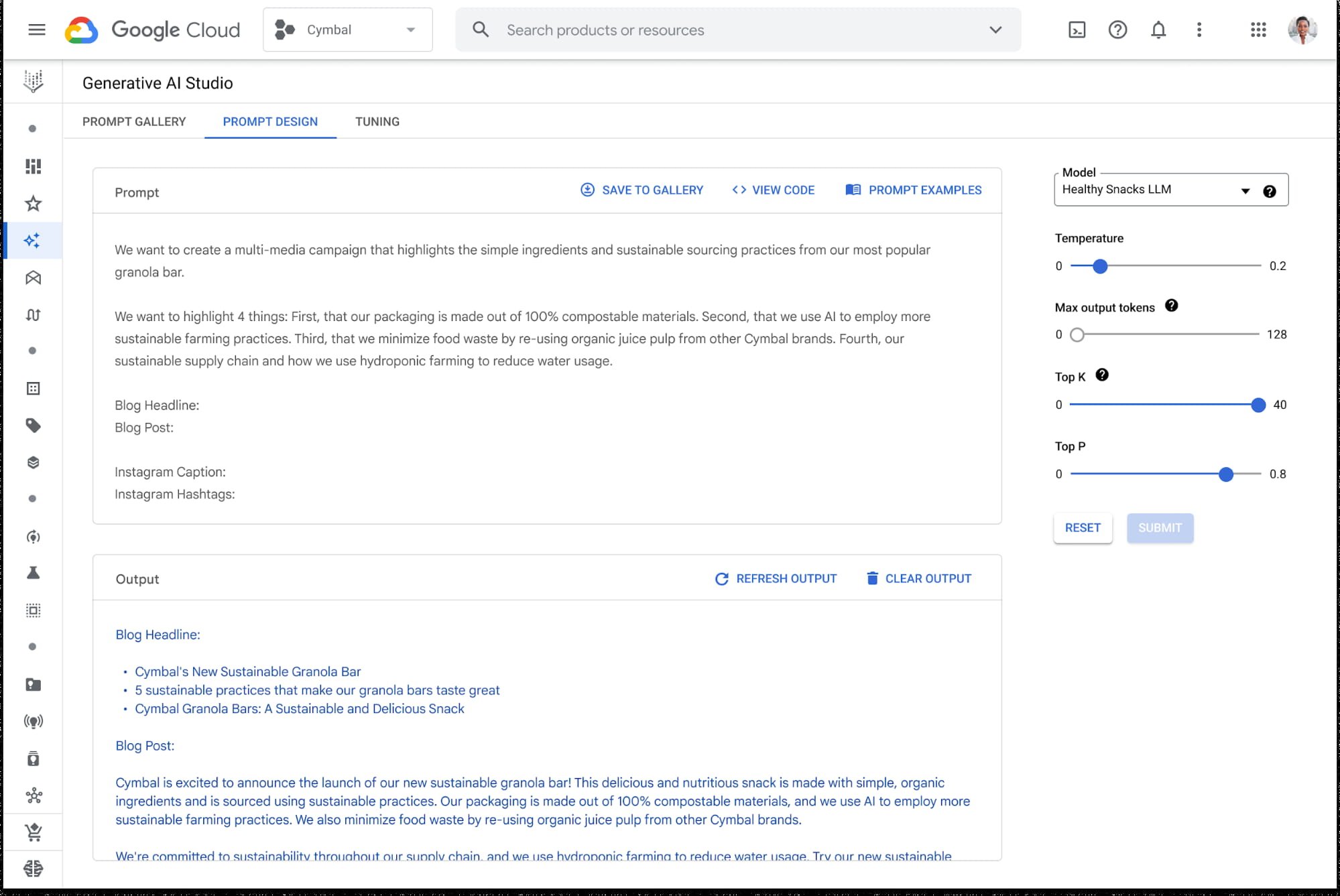
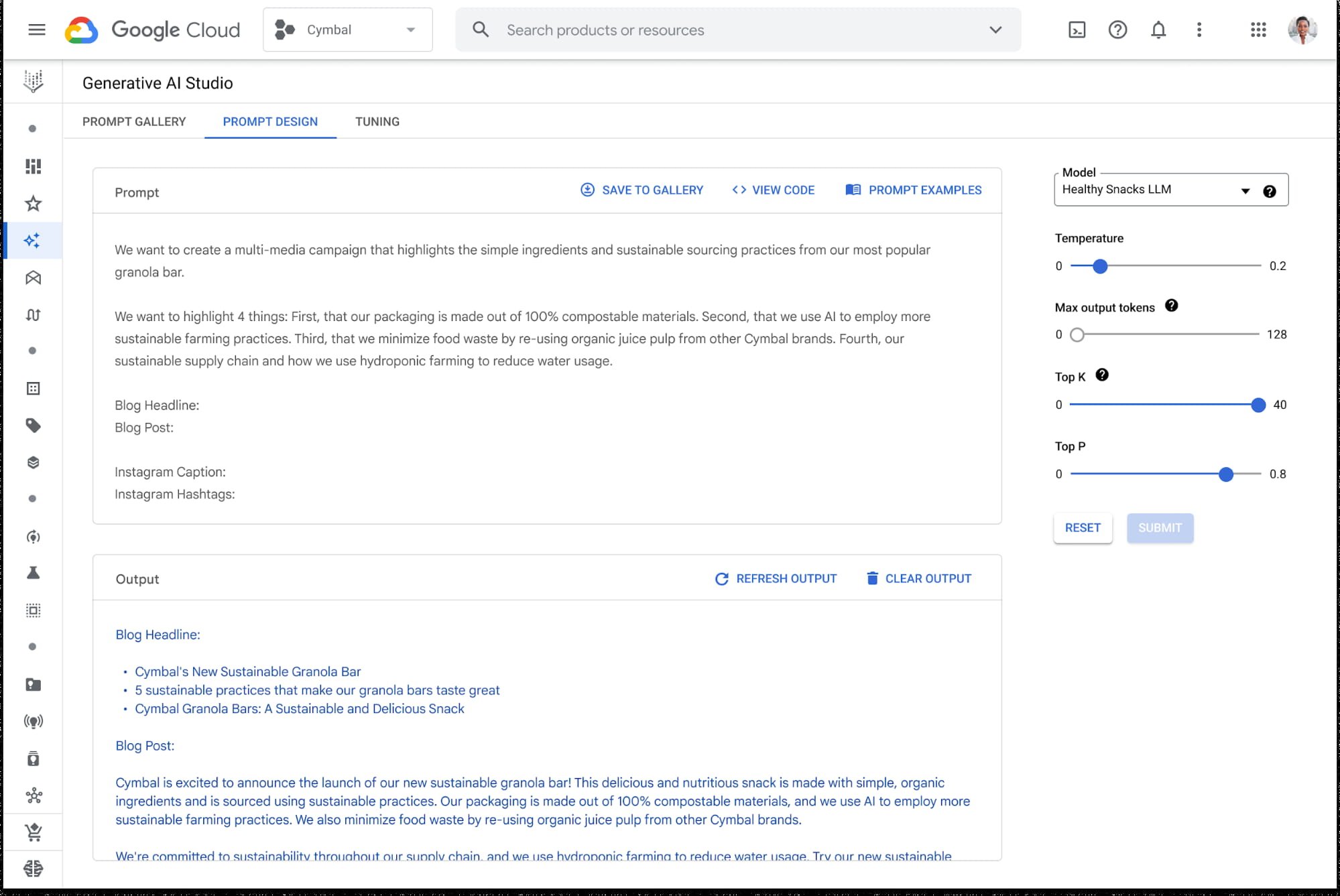
3. Prompt tuning: Prompt tuning is an efficient, low-cost way of customizing a foundation model without retraining it. Prompts are how we guide the model to generate useful output, using natural language rather than a programming language. In Generative AI Studio, it’s easy to upload user data that is then used to prompt the model to behave in a specific way. For example, if a user wants to update the PaLM language model to speak in their brand voice, they can simply upload brand documents, tweets, press releases, and other assets to Generative AI Studio.
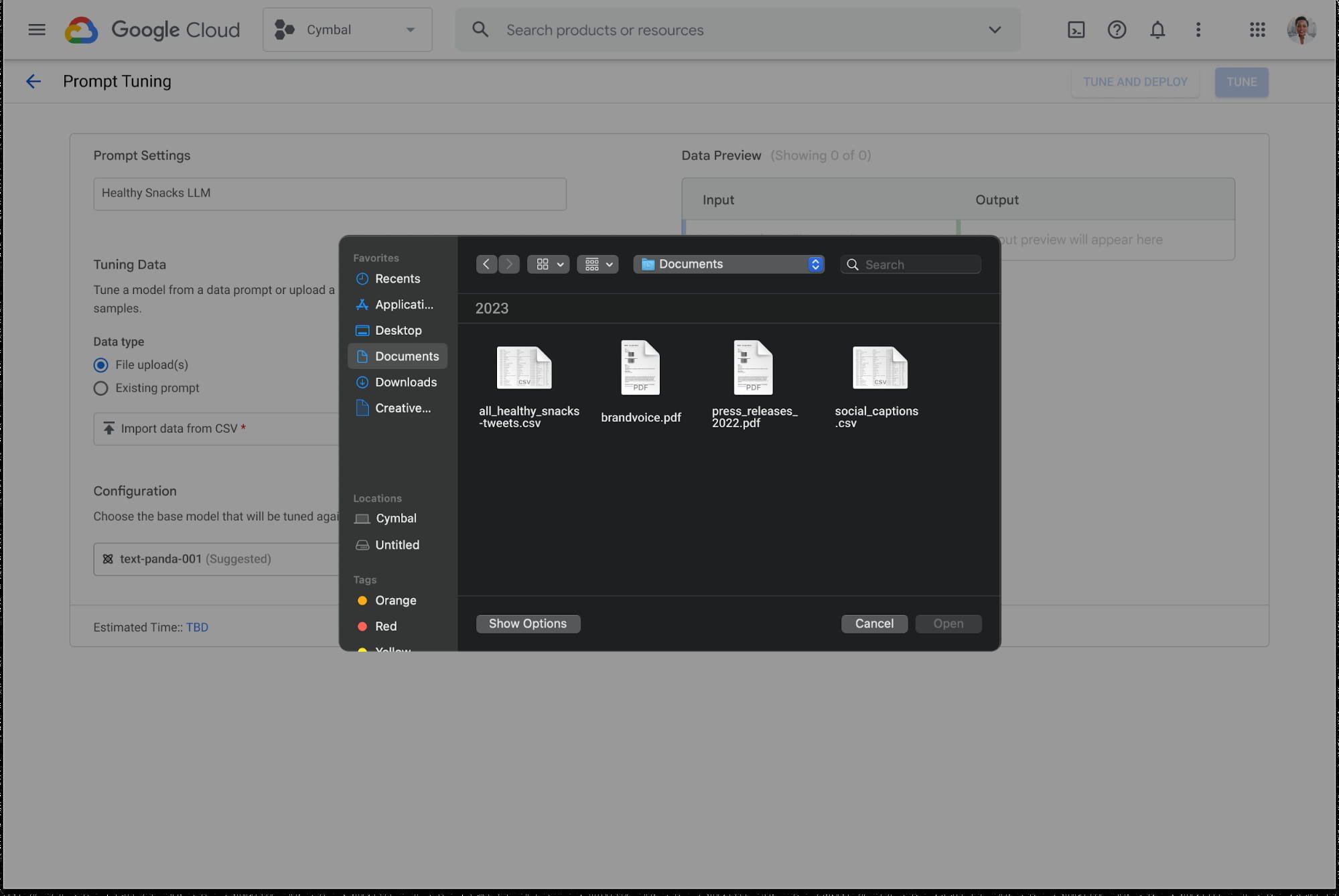
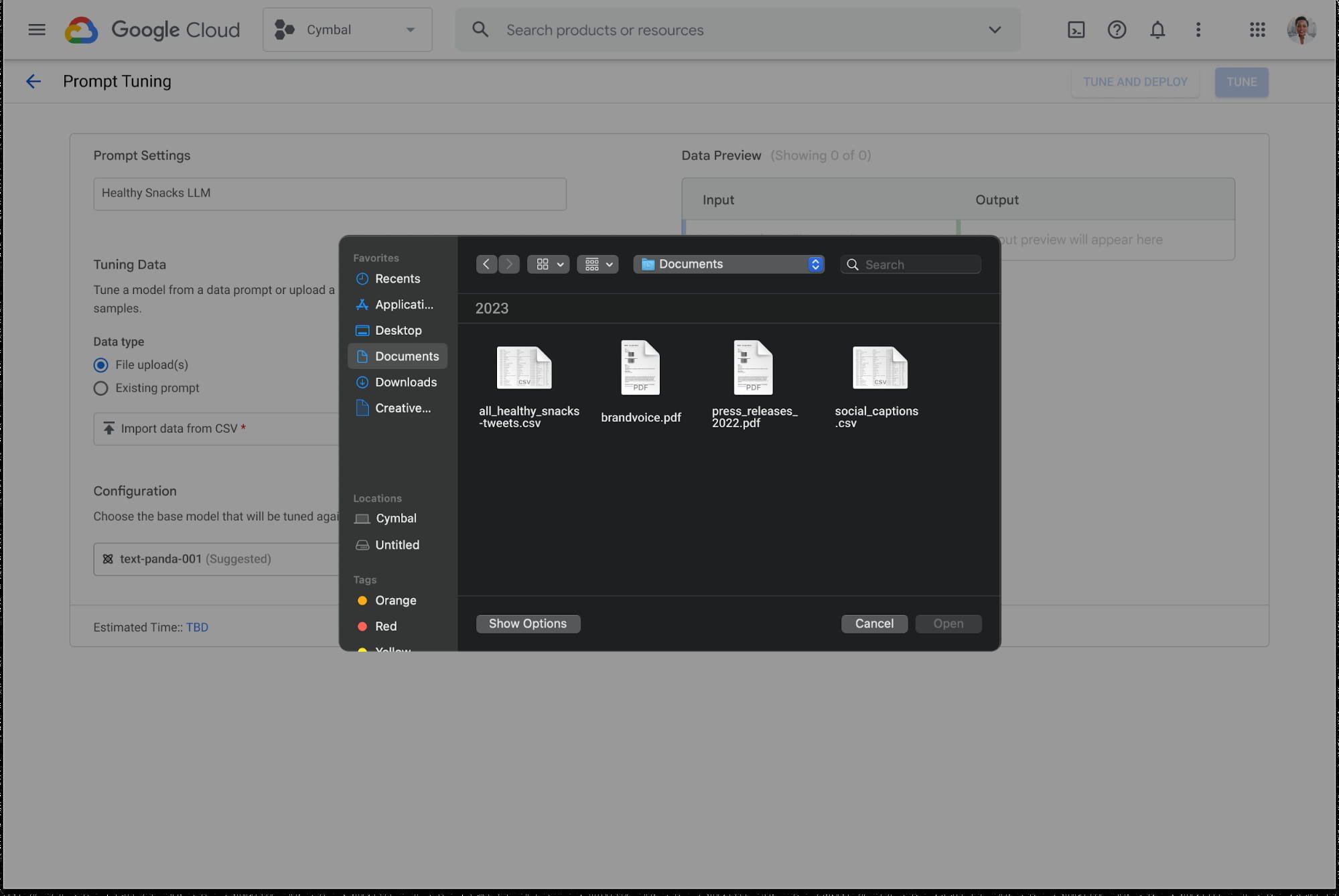
4. Fine-tuning: Fine-tuning in Generative AI Studio is a great option for organizations that want to build highly differentiated generative AI offerings. Fine-tuning is the process of further training a pre-trained model on new data, resulting in changes to the model’s weights. This is helpful for use cases that require outputs with specialized results, like legal or medical vocabulary. In Vertex AI Generative AI Studio, users can upload large data sets and re-train models using Vertex AI Training. Google Cloud offers you the ability to fine-tune your model without exposing the changes in the weights outside your protected tenet. This enables you to use the power of foundation models without your data ever leaving your control.
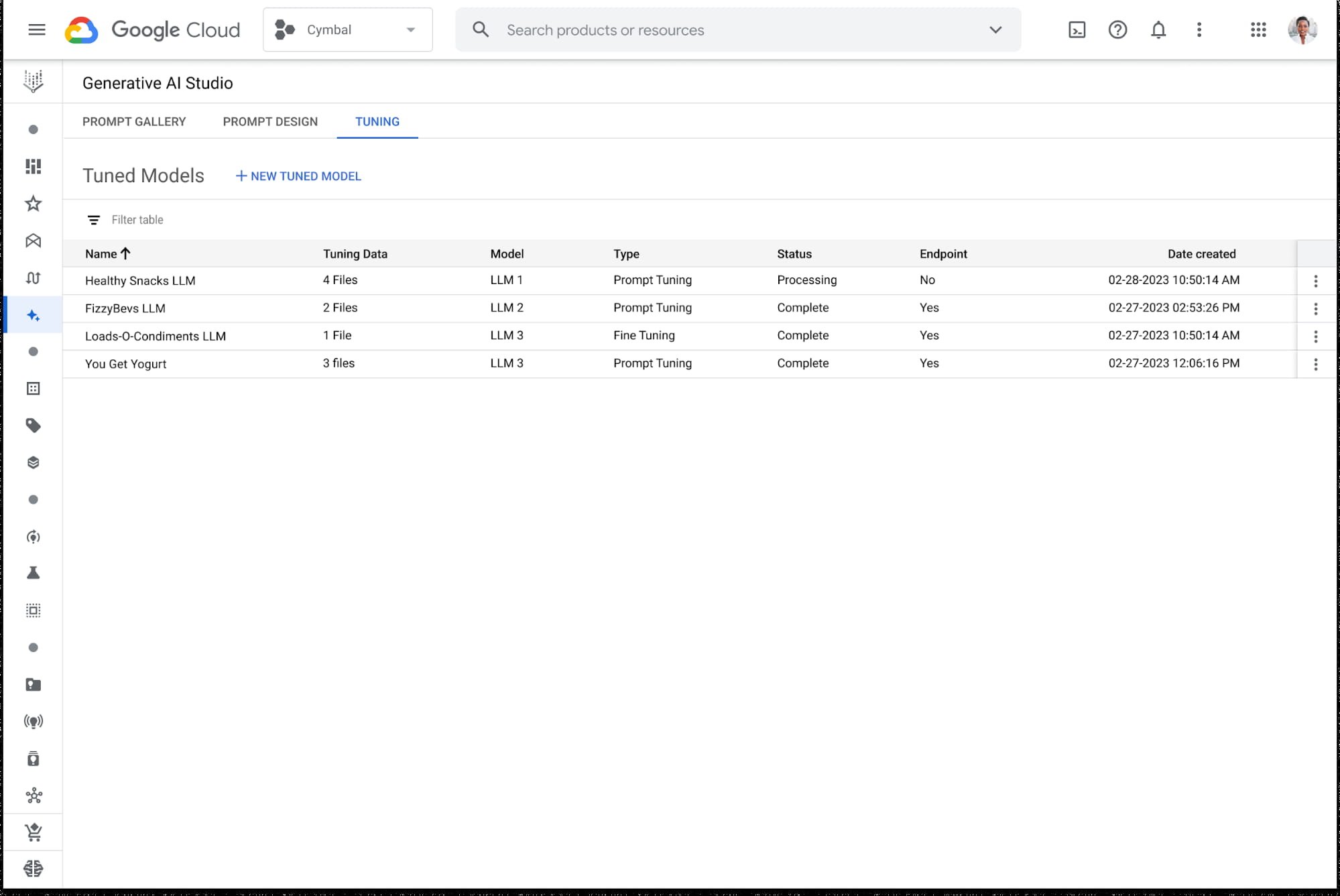
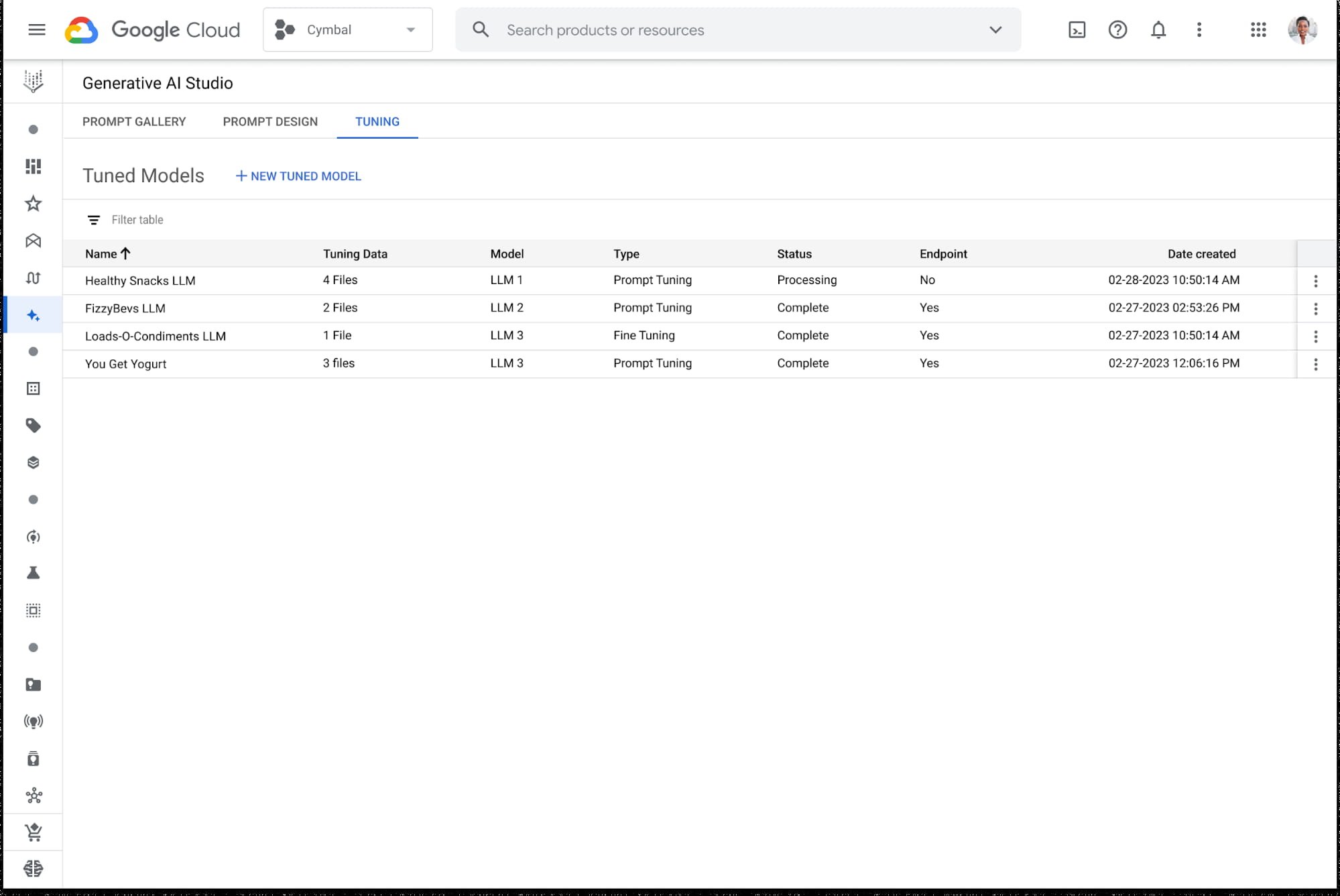
5. Cost optimization: At Google, we have run these models in our production workloads for several years, and in that time, we’ve developed several techniques to optimize inference for cost. We offer optimized model selection (OMS), which looks at what is being asked of the model and routes the request to the smallest model that can effectively respond to it. When enabled, this happens in the background and is invoked based on different conditions.
Early customers are excited about Generative AI support in Vertex AI
“Since its launch, Vertex AI has helped transform the way CNA scales AI, better managing machine learning models in production,” says Santosh Bardwaj, SVP, Global Chief Data & Analytics Officer at CNA. “With large model support on Vertex AI, CNA can now also tailor its insights to best suit the unique business needs of customers and colleagues.”
“Google Cloud has been a strategic partner for Deutsche Bank, working with us to improve operational efficiency and reshape how we design and deliver products for our customers,” says Gil Perez, Chief Innovation Officer, Deutsche Bank. “We appreciate their approach to Responsible AI and look forward to co-innovating with their advancements in generative AI, building on our success to date in enhancing developer productivity, boosting innovation, and increasing employee retention.”
New business-ready generative AI products are available today to select developers in the Google Cloud trusted tester program.
Visit our AI on Google Cloud webpage or join me at the Google Data Cloud & AI Summit, live online March 29, to learn more about our new announcements. Who knows, I may even throw in some dance moves.