Google Cloud’s AI Adoption Framework: Helping you build a transformative AI capability
Donna Schut
Head of Technical Solutions, Applied AI Engineering
We believe that enterprises that invest in building AI solutions are better positioned to be the industry leaders of tomorrow. Artificial intelligence (AI) can help organizations improve, scale, and automate the decision-making process across most business functions, while machine learning (ML) can create new opportunities and help you develop new revenue streams to grow your business. Together, they can drive significant value and give you a competitive advantage in your market.
However, building an effective AI capability in your organization can present challenges, including: the technology you need to support building platforms and solutions, the people you need to implement and operate them, the data you need to fuel them, and the processes you need to govern them.
When building an AI capability, executives often ask us:
“Which skills should we hire and how should we structure our teams?”
“What ML projects should we prioritise? ”
“How do we implement responsible and explainable AI?”
On the other hand, engineering leads often question:
“How can we make data and ML assets discoverable, shareable, and reusable?”
“How can we utilize cloud-native services to scale?”
“How can we operationalize data processing and ML pipelines in production?”
We created the Google Cloud AI Adoption Framework to help answer all these questions and more. This whitepaper aims to provide a guiding framework for technology leaders who want to leverage the power of AI to transform their business. It’s informed by Google’s own evolution, innovation, and thought leadership in AI, as well as many years of experience helping cloud customers—from startups to enterprises, in various industries—solve complex challenges.
The AI Adoption Framework builds a structure on four areas: people, process, technology, and data. The interplay between these areas highlights six themes that are critical for success: Lead, Learn, Access, Scale, Automate, and Secure.
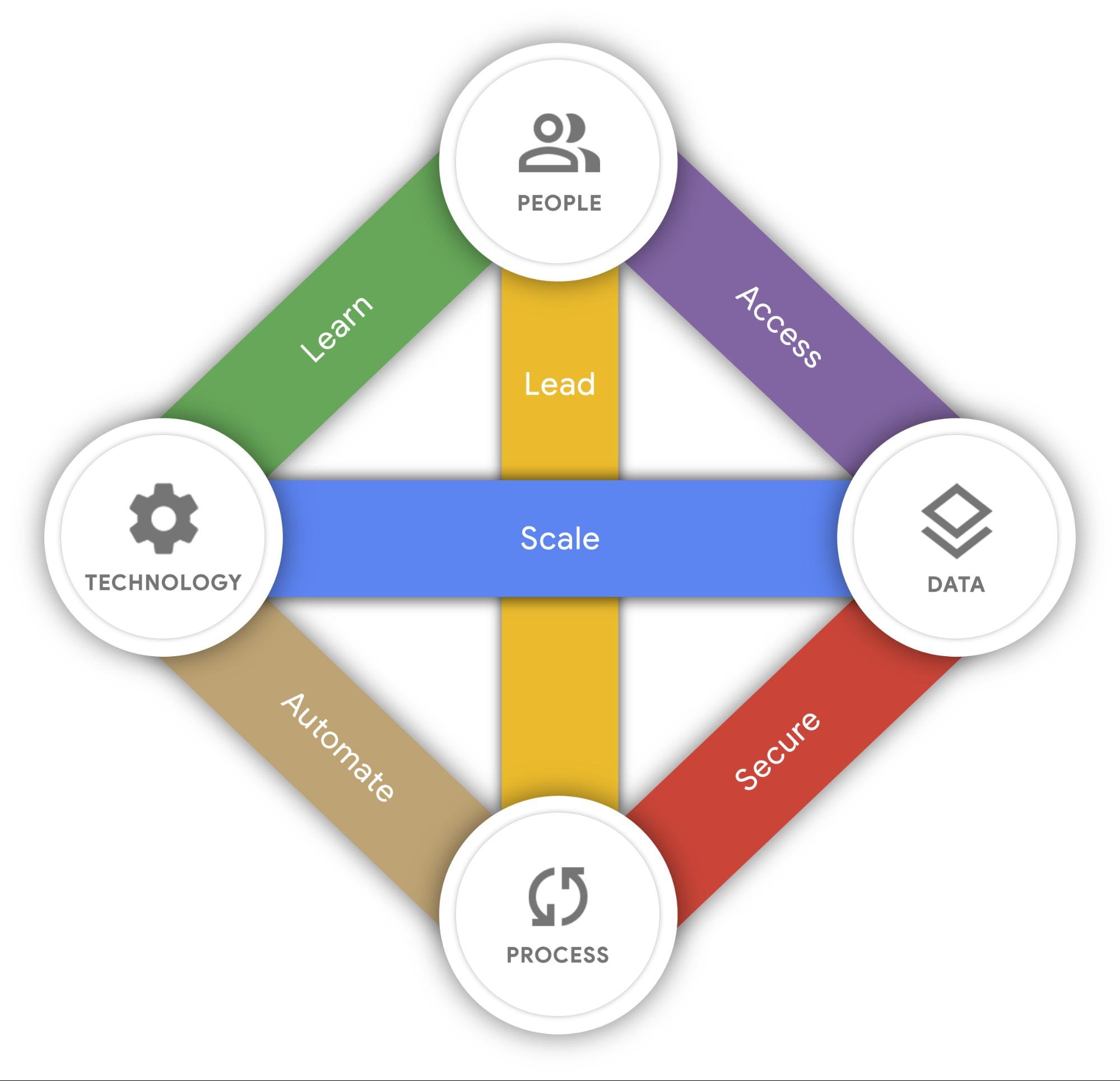
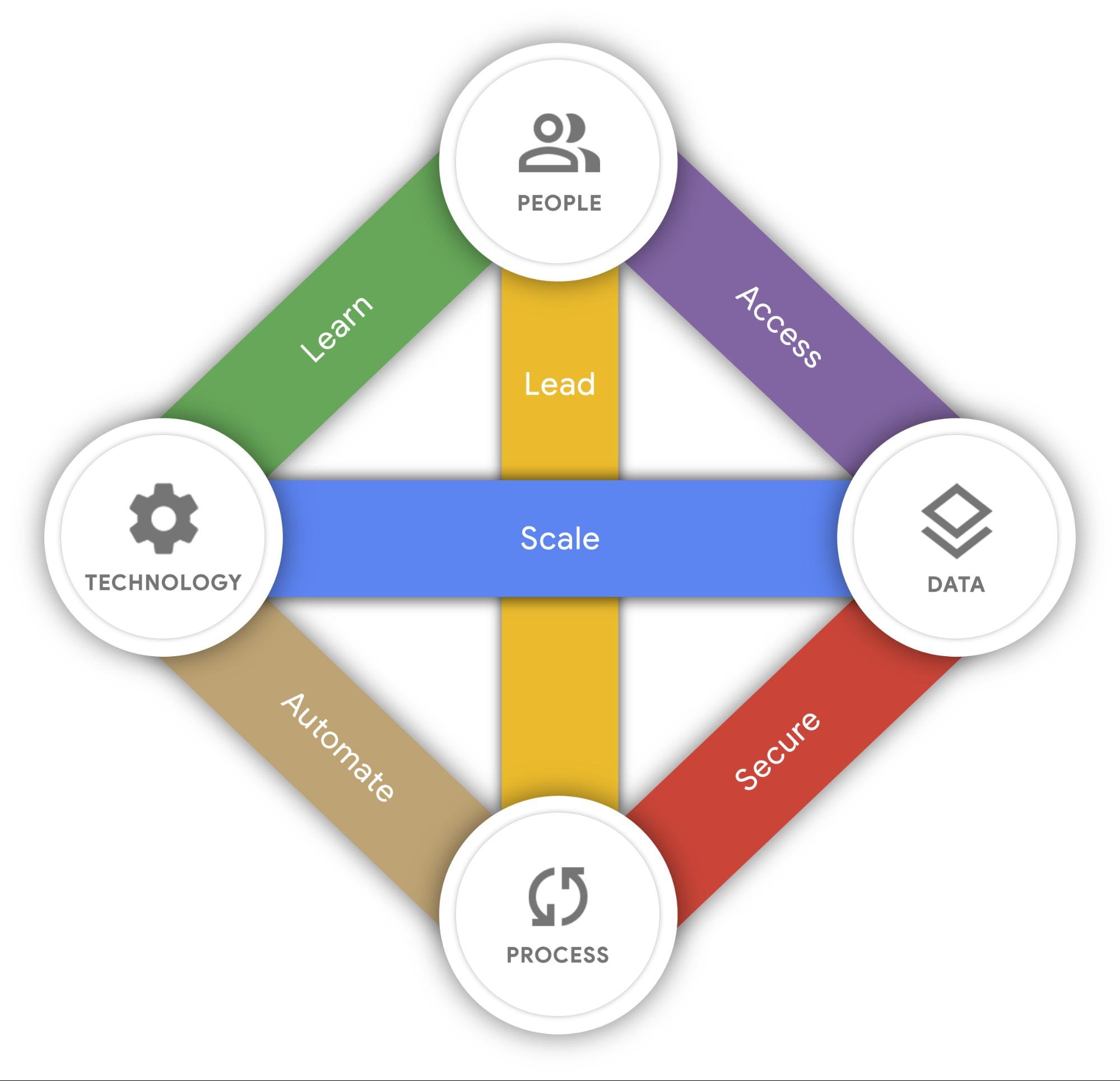
Lead refers to the extent that leadership provides support and encouragement for data scientists and engineers to apply ML to business use cases, and the degree to which they are cross-functional, collaborative, and self-motivated.
Learn entails the quality and scale of learning programs to upskill your staff, hiring talented people, and augmenting your data science and ML engineering staff with experienced partners.
Access is about recognizing data management as a key element for enabling AI, and the degree to which data scientists and ML engineers can share, discover, and reuse data assets and other ML artifacts.
Scale covers how you can use cloud-native services to scale with big datasets and a large number of data processing and ML jobs to reduce operational overhead.
Secure addresses how you can classify and protect your sensitive data, as well as ensure that you’re implementing responsible and explainable AI practices.
Automate encompasses the ability to deploy, execute, and operate technology for data processing and ML pipelines in production efficiently, frequently, and reliably.
Successfully adopting AI in your business is determined by your current business practices in these areas, each of which will fall into one of three maturity phases: tactical, strategic, or transformational. Knowing how mature your business is in each of these categories can help you determine where you are on your AI adoption journey today, and where you’d like to be.
Download the whitepaper to learn more.
Acknowledgements
This whitepaper was authored by Donna Schut, Khalid Salama, Finn Toner, Barbara Fusinska, Valentine Fontama, and Lak Lakshmanan with valuable contributions from many teams across Google, including the Office of the CTO, ML Specialists, Solution Engineering, Professional Services, and Cloud AI.