The Prompt: Why grounding is the foundation of successful enterprise AI
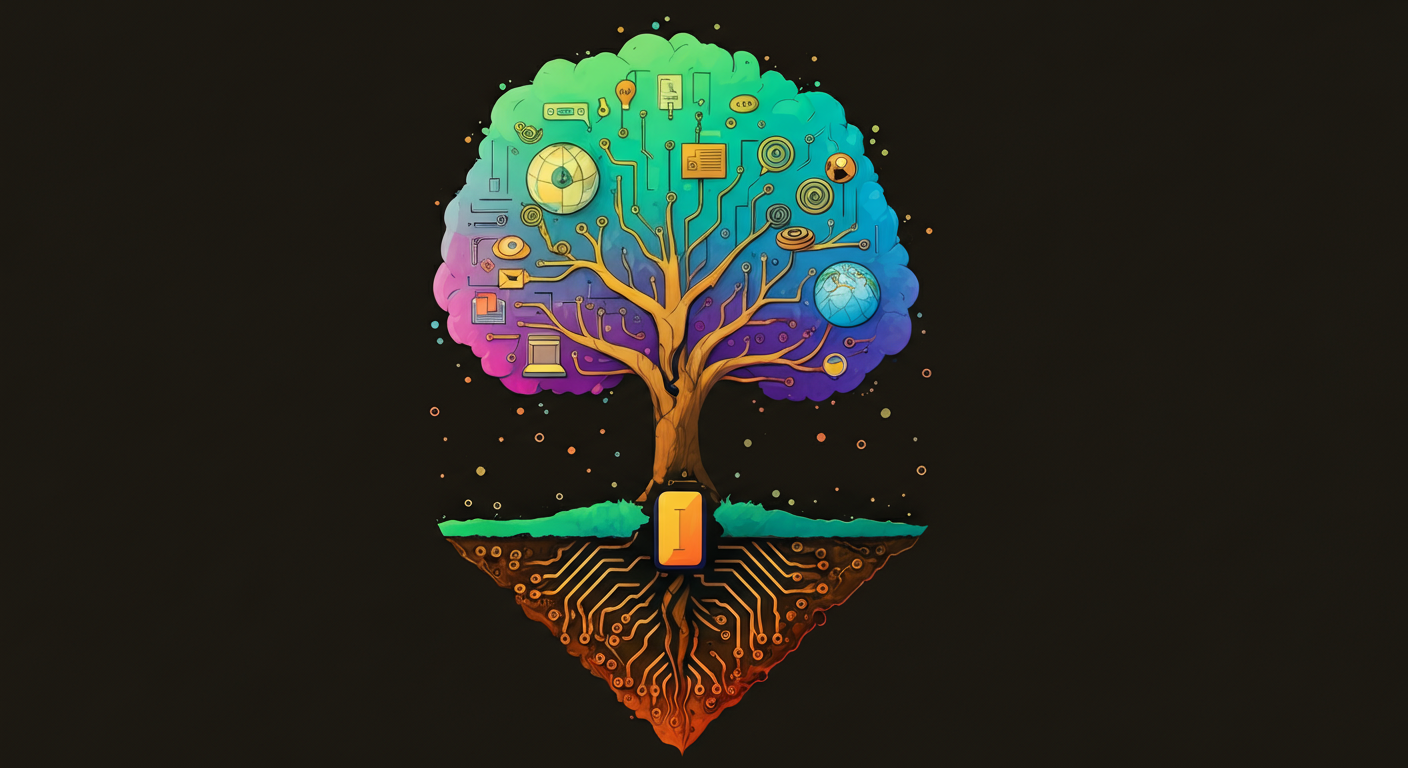
Warren Barkley
Sr. Director of Product Management
If you want to build your organization's trust in AI outputs, grounding in enterprise truth and real-world data is an essential ingredient.
Business leaders are buzzing about generative AI. To help you keep up with this fast-moving, transformative topic, our regular column “The Prompt” brings you observations from the field, where Google Cloud leaders are working closely with customers and partners to define the future of AI. In this edition, Warren Barkley, Vertex AI product leader, looks at how organizations can ground gen AI models in factual data to provide more reliable responses that build trust and confidence in all users, whether customers or employees.
Generative AI is a business game-changer, but with a notable caveat: it needs access to real-world context and information to be truly useful in the enterprise.
Though powerful, gen AI models don’t come primed for your industry or know the inner workings of your business. They are limited to what they know from their training data, which often lacks the necessary information and domain expertise for specific business tasks and use cases. Models also have a knowledge cutoff, meaning they are not aware of new developments and information that occurs beyond their training. More critically, these gaps in knowledge can contribute to models generating irrelevant, factually incorrect, or, in rarer cases, hallucinations — completely made up responses.
In other words, foundation models are trained to predict the most probable answer based on training data, but that’s not the same thing as citing facts. To unlock the full potential of gen AI, organizations need to ground model response in what we call “enterprise truth“ — fresh, real-time data and enterprise systems. This approach lets models retrieve the context they need from external systems, so they can find the latest, most relevant information instead of relying on their limited and potentially outdated training knowledge.
Over the last year, grounding has come to the forefront in many of our conversations with customers and partners alike, especially as more and more move from experimenting with gen AI to putting AI into production. Increasingly, executives are realizing foundation models are simply a starting point, and they are exploring how to use grounding approaches like retrieval augmented generation (RAG) to add context from reliable information sources, their own first-party data, and the latest information from the web.
In this column, we’ll explore some of the benefits and challenges of RAG for grounding models, and how they relate to the solutions we’re building at Google Cloud.
Bringing facts to abstract knowledge
In general, the quickest and easiest way to provide additional background information to models is through prompting. It’s possible to give models an extensive amount of information, with context windows — the amount of information a model can recall before it starts “forgetting” previous interactions — now reaching up to a staggering two million tokens.
For example, you could put an entire employee handbook into a long context window and create a context cache to handle multiple prompts for the same information, which can pull relevant information for more accurate model output, similar to RAG. However, manual efforts don’t scale well, especially when querying frequently updated data or a large corpus of enterprise knowledge bases.
To automatically enable gen AI models to retrieve relevant, factual information, you will need some form of grounding solution, such as RAG.
Suppose you want to create an AI agent to help employees choose the right benefits package to fit their needs. Without grounding, the agent would only be able to generally discuss how the most common employee benefits work based on its training data, but it wouldn’t have any awareness about the benefits your organization offers. Plus, even if an agent’s training data included all your employee benefits, individual programs change all the time; it’s likely a model would quickly fall out of date without any way to reference newly updated information.
In this scenario, RAG could connect your models to plan policies, detailed benefit summaries, carrier contracts, and other relevant documentation, allowing agents to answer specific questions, provide recommendations, or enroll employees directly in online portals — all without the need to retrain or fine-tune models.
These advantages are why RAG is now the primary approach being pursued by organizations seeking to ground their gen AI applications and agents in enterprise truth. With an increasingly robust ecosystem of products and integration available, we’re seeing more and more possibilities emerge that can help tackle use cases that demand domain-specific knowledge and deep contextual awareness. There are several different ways to incorporate RAG into gen AI, ranging from simpler approaches like linking models directly to the internet for recency to more complex custom-built RAG systems.
To build or not to build
While it’s likely you’ve heard or read somewhere how “easy” RAG is to do, I’ve yet to meet a customer that agreed. Despite the obvious benefits, many leaders and executives tell us they are struggling to build RAG systems that are good enough to trust for grounding their gen AI applications and agents.
In many ways, building your own RAG is similar to building a search engine that can pull results from a carefully curated dataset. Custom RAG solutions involve converting data into vectors (numerical representations) using embedding models. Vectors help to map high-dimensional semantic relationships between words, inferring concepts like “dog” has a different relationship to “wolf” due to their shared ancestry than the word “cat,” which is also a domesticated pet. This enables you to look up and identify concepts that are semantically related to each other.
With RAG, vectors are stored in a specialized vector store for efficient search, retrieved based relevancy to user queries, and then fed to a language model as context. This not only requires selecting what data sources to include but also defining the most important points within it. It’s a tough undertaking for any organization, no matter the level of maturity.
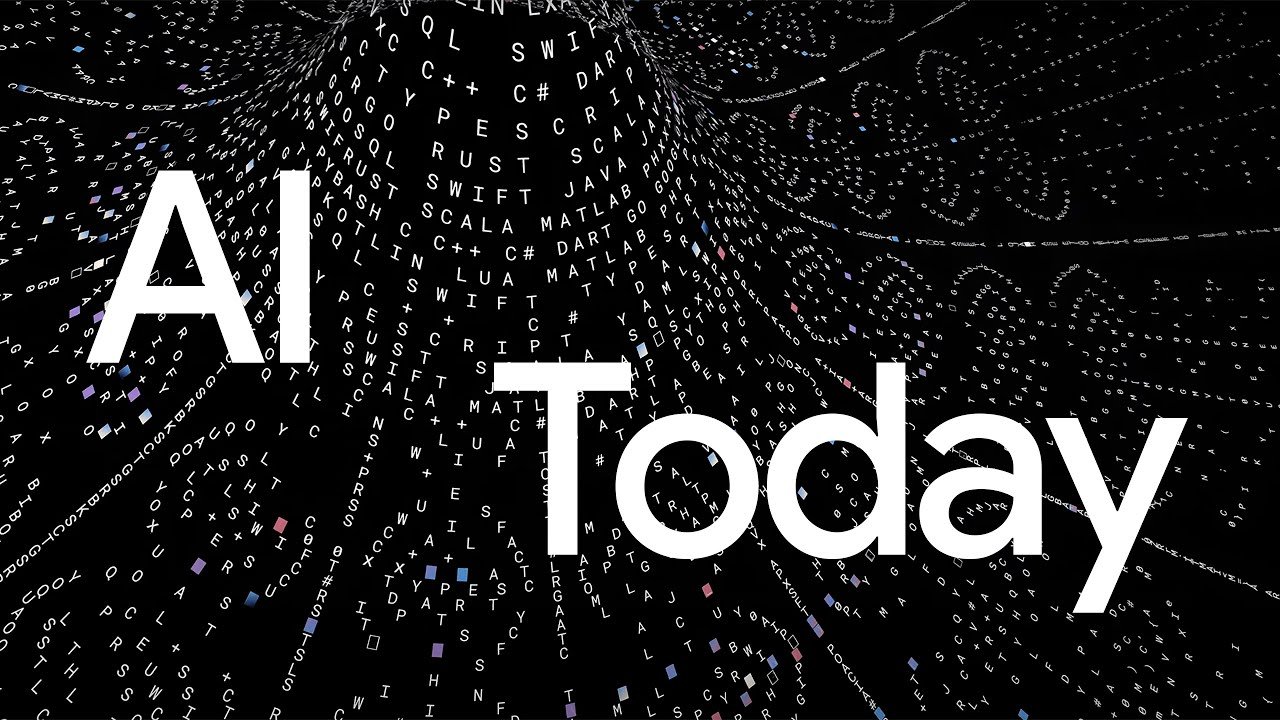
In general, the most common challenges that come up relate to data quality and management. Like any data or AI system, the success of RAG relies heavily on how well your data is organized and maintained, and how well you understand what it is and how it relates to your overarching objectives and goals.
In particular, many companies overlook the importance of data hygiene when they start implementing RAG components. The old computer science adage of “garbage in, garbage out” still holds true in the gen AI era; if your data is incomplete, inconsistent, or inaccurate, the information RAG retrieves will be too. For instance, if you have two pieces of data in your dataset that contain varied information for the same query, you might end up getting a different answer every time that question is asked.
In addition, embedding models come with their own set of considerations and constraints:
- How rich is the data you’re trying to convert into vectors?
- How diverse is the data?
- How will you determine the relevance for each vector based on its similarity to queries?
- What mechanisms can help rank their importance?
These questions only become more complex as you introduce multimodal models and data, which requires identifying the relationships and connections across many different types of data, including text, images, video, audio, and even code.
Anecdotally, we have seen that as much as 80% of RAG efforts are spent trying to get data foundations in order. And given how fast gen AI models are evolving and emerging, teams are already starting to accumulate technical debt before they’ve managed to develop solutions that can deliver reliable enough answers to support their use cases.
Grounding gen AI needs to be easy
At Google Cloud, we believe that grounding responses in enterprise truth is the key to adopting gen AI at full speed. Organizations not only need amazing foundation models, they need capabilities that empower them to augment their training data with RAG from a wide variety of reliable fresh data and information – whether public, private, or multimodal. And they need it to be easy to build and implement, too.
Much of our recent and ongoing, work is focused on making RAG an attainable reality for everyone.
Vertex AI comes with grounding capabilities for a variety of needs and requirements, including building custom RAG workflows for retrieval, ranking, and document processing to make it easier to ground gen AI in your own private data. For more factual responses in highly-regulated or data-intensive industries like healthcare, financial services, or insurance, there is high-fidelity mode, which only sources information for response generation from the context you provide, not the model’s world knowledge, to accelerate response time and ensure higher levels of factuality.
At Google Cloud, we believe that grounding responses in enterprise truth is the key to adopting gen AI at full speed.
We also recently announced the ability to ground gen AI models in Google Search — one of the world’s most trusted sources of fresh, factual, and high-quality information — and we’re currently working with specialized providers, such as Moody’s, MSCI, Thomson Reuters, and Zoominfo to enable incorporating even more, reliable external datasets.
We’re entering the era of enterprise-ready gen AI, where accuracy, timeliness, and contextualization are now becoming non-negotiable requirements to fuel gen AI innovation. With an increasingly robust ecosystem of products and integration available, incorporating RAG into gen AI systems will further expand the opportunities to tackle use cases that demand domain-specific knowledge and deep contextual awareness.
Interested in learning more? Download our comprehensive guide to find out how to ground your gen AI models in enterprise truth and gain a competitive edge.
To keep up with the very latest in GenAI on Vertex, check out our guide.