Turning chicken scratch into data riches: The secret ingredients in Tyson Foods' smart factories
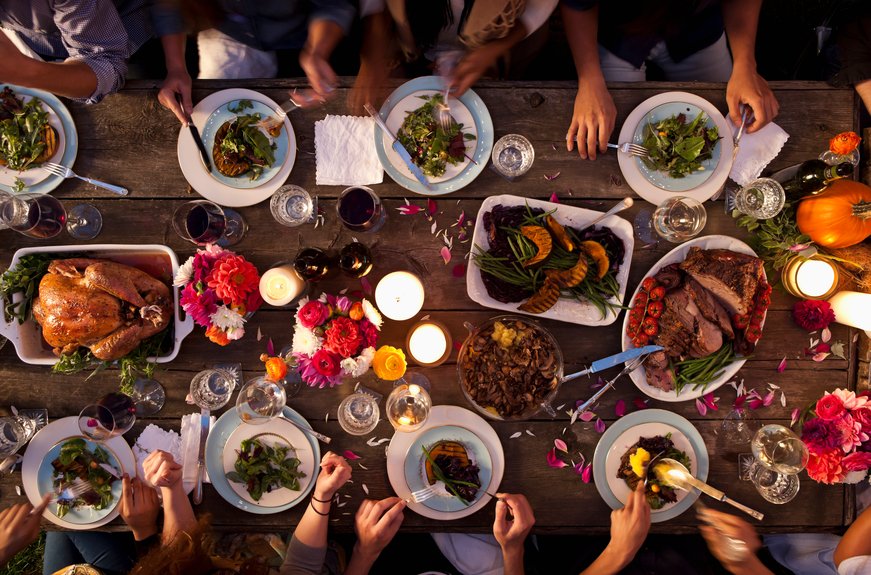
Barret Miller
Senior Manager of Emerging Tech, Tyson Foods
Nate Marks
Senior Staff Solutions Architect, Tyson Foods
One of the world's largest meat producers is using data, analytics, and AI to more efficiently feed a growing world.
A few years ago, at Google Cloud NEXT, our team was in line for a panel, and another guest turned to us and asked which company we were from. When we said Tyson Foods, he asked, “What’s a chicken company doing here?”
Yes, we are a food manufacturing company, and we’re one of the largest meat production companies in the world. We also have ambitious goals for what we believe cloud computing technology can do to accelerate the consumer packaged goods industry.
Our emerging technologies team has become a core function of the company because Tyson Foods is committed to finding technology that is use-case driven and fit for specific business purposes. Our work with Google Cloud is just one example of how we’re rethinking technology to make a meaningful impact across our operations.
With global operations aimed at feeding a growing world, we needed to centrally manage and process data for hundreds of factories and warehouses across the planet.
As we looked to the future of our relationship with our data, we started to reflect on what capabilities we would need to do that. Cloud and edge computing were immediate choices.
Bringing the power of data to the factory floor
Connecting our hundreds of locations, in addition to our hatcheries and trucking fleet, would require us to process millions of data points from hundreds of thousands of sensors. The data sent from those sensors could be joined upstream with our other operations data to help us make better process decisions, so we needed a way to collect Internet of Things (IoT) data at scale.
We also needed to be able to get our enterprise data down to the factory floor. Factory teams need accurate and reliable manufacturing and standards data to monitor efficiencies on their machines and orders, identify any issues, and address them quickly.
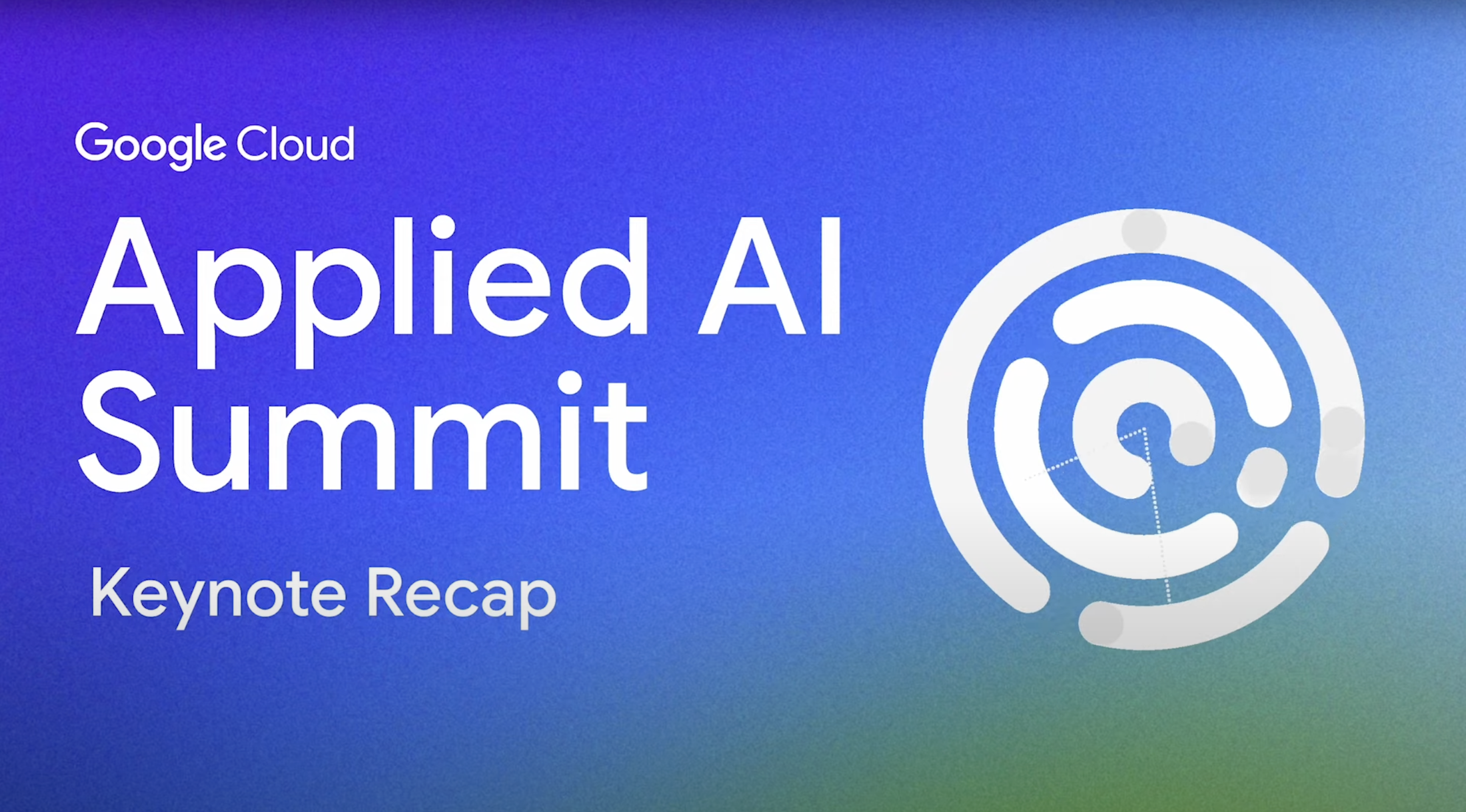
The process for sharing data varied from location to location, which meant that some factories could go long periods without updated data. For years, if that location’s internet went down, the local team would have to resort to pen-and-paper tracking, which would then be entered manually into the system when it came back online.
We’d already used Google Cloud to manage and sync our enterprise data, so we knew we could trust it in the next stage of data journey.
Designing a data-first operations infrastructure
We started to explore ways to better make use of data to make our factory and enterprise teams more efficient. As part of that search, we also wanted a solution that would help us relieve some of our technical debt, including replacing unreliable legacy servers. We found that Manufacturing Data Engine could tackle both problems at once.
We discovered it was easy to move data back and forth between our data lake, BigQuery, and the factory floor by funneling data directly through our new data system which gives us a future-proof solution for advanced analytics with machine learning and computer vision models.
Our first factory deployment took a month and a half, and it’s only getting faster as we use our new data architecture to standardize rollouts for future locations. This process includes deploying the data engine, setting it up, configuring it, and building the data models that manage everything from orders and engineering standards to materials and throughput rates.
With this system in place, we have the ability to monitor and capture small changes in data.
For example, we can use the “time travel” feature to record precise time information about processes on the factory floor or in shipping processes by capturing default logging events that BigQuery syncs to Cloud Logging automatically. We also have the flexibility to filter, sample, and aggregate data in different ways. In one case, if we have a sensor that we read every 10 milliseconds, we may not want to send that data at the same rate, and we can adjust as needed.
In total, we’ve designed a system that is simple to manage with the fewest moving parts and the most reliable, near real-time data available.
Scaling smart factories for a new generation of consumer packaged goods
Having access to the information we can capture with Manufacturing Data Engine is adding an unprecedented amount of value to teams on the ground. One plant had spent years trying to manually extract and review operations data from our enterprise resource planning (ERP) system to improve its manufacturing processes. With our new data processing solutions in place, the factory can now access the data it needs in a very short period of time.
Because we created a replicable and scalable deployment process, we can continue to give our end-users the information they need efficiently across all our facilities. All we have to do is deploy the system in the factory’s environment, provision the resources, connect that new deployment to our system, and the data starts flowing.
Directly connecting operations data to IoT data points and vice versa has allowed us to modernize and future proof at the same time.
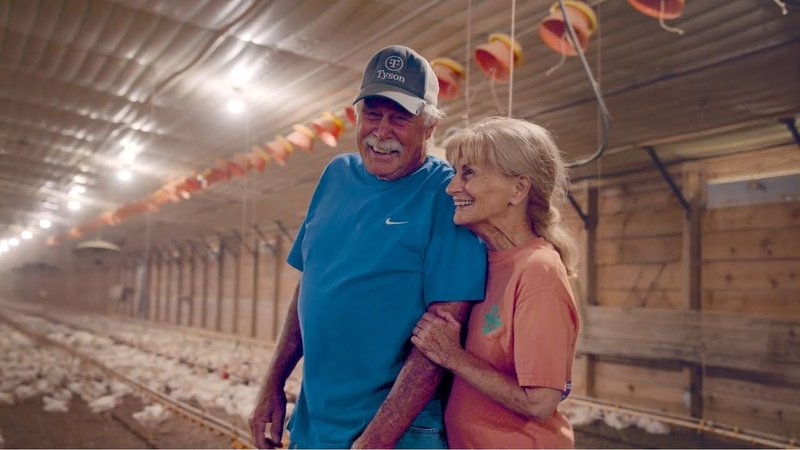
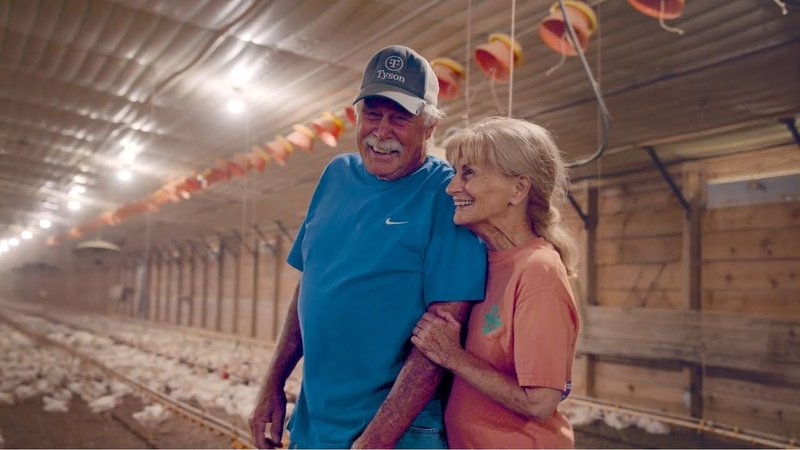
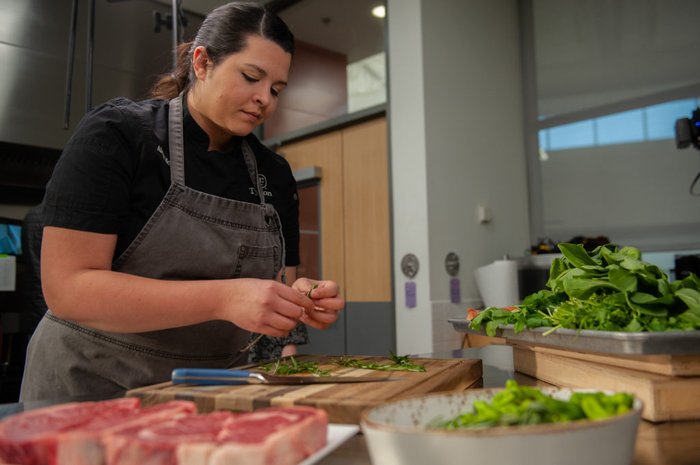
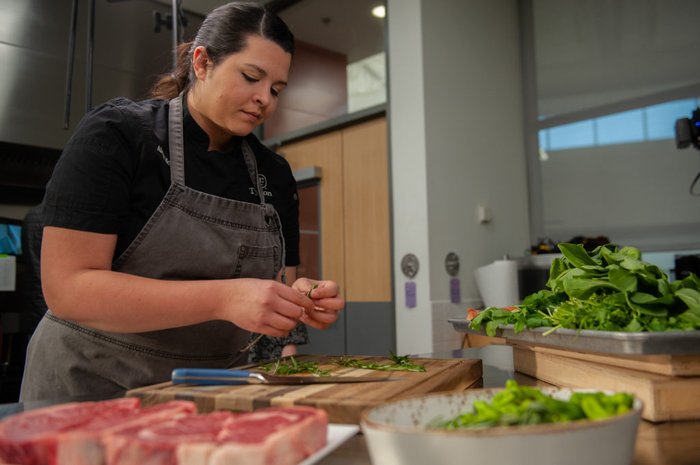
Data is helping manage supply chains from the farm all the way to the kitchen.
This evolution includes being able to give our factory teams the resources they need, when they need them. For years, work orders had to be handled from our ERP system, sent to a central office location on the plant floor, and the managers would have to write the order down or print it out to know what they needed to produce. Sometimes, this process would include keying information into other systems manually too. Being able to connect our systems together to streamline that will help us all move faster together.
With data flowing freely across systems and locations, our engineers can focus even more on the future of consumer packaged goods. This includes finding ways to integrate legacy protocols and systems to clean up even more technical debt, and evaluating new use cases for machine learning and AI.
Our team sees the next generation of smart factories using unstructured data, such as images or videos, to train computer vision models to monitor hardware or IoT-connected sensors to optimize patterns. The future of our industry is going beyond one-off solutions and relying on repeatable, scalable enterprise solutions.