The Prompt: What is long context — and why does it matter for your AI?
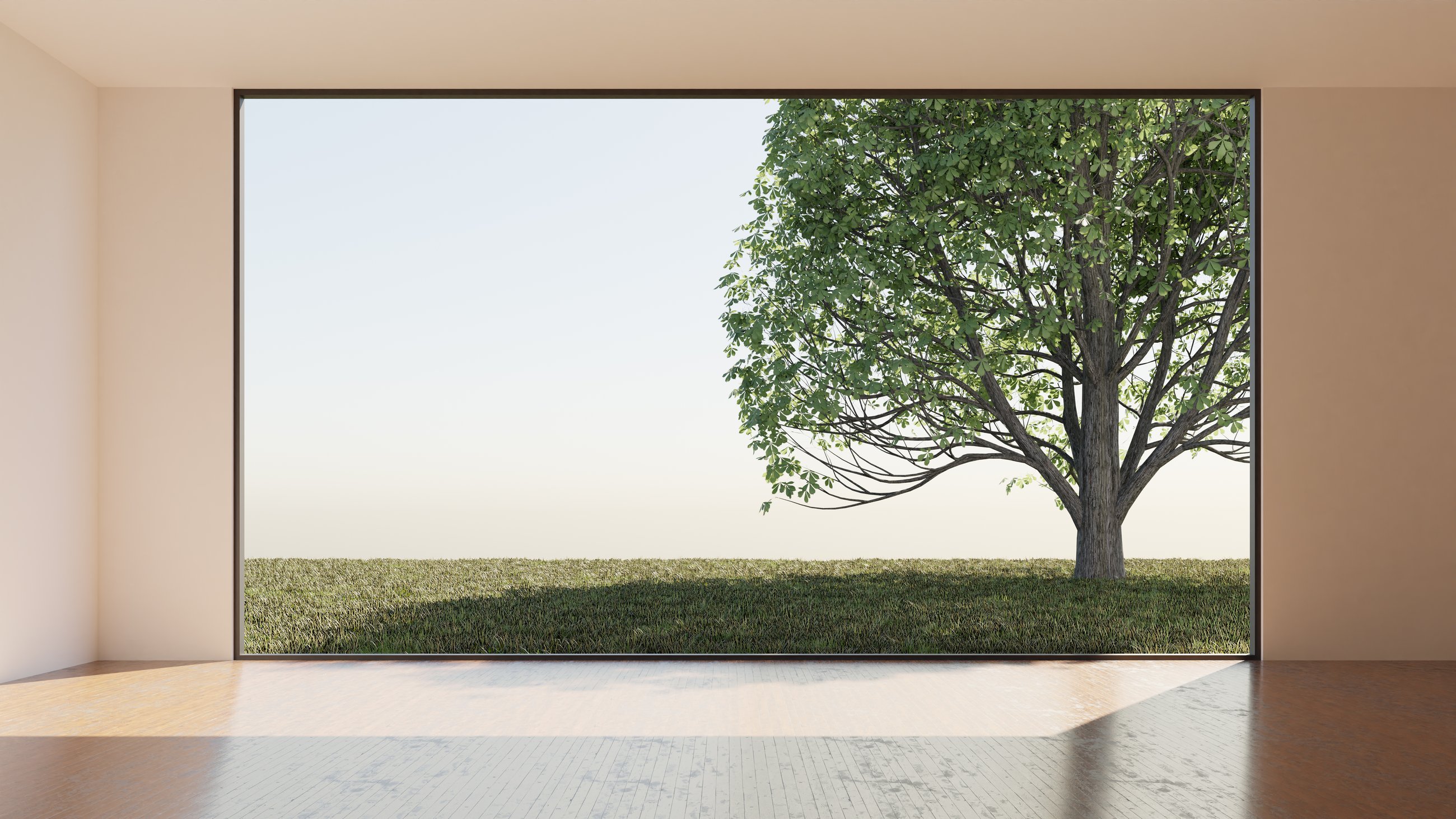
Warren Barkley
Sr. Director of Product Management
Expanded context windows are driving a new wave of capabilities in generative AI, enabling more accurate and sophisticated interactions.
Business leaders are buzzing about generative AI. To help you keep up with this fast-moving, transformative topic, our regular column “The Prompt” brings you observations from the field, where Google Cloud leaders are working closely with customers and partners to define the future of AI. In this edition, Warren Barkley, Vertex AI product leader, explores the use cases that benefit from long context windows and key considerations when using them.
No matter where your organization is in its generative AI journey, keeping track of what’s coming next is important as new advancements and innovations emerge at record speed. In the last year, one of the most significant developments that deserves attention is the explosive growth in context window size.
Context windows are the amount of information a gen AI model can recall during a session, measured in tokens — the smallest building blocks a model can process, such as a part of word, image, or video. The longer the context window, the more data a model can process and use. For example, Gemini 1.5 Pro model features our industry-leading context window of up to 2 million tokens. In more practical terms, that means it can process about 1.5 million words at once, roughly the equivalent of 5,000 pages of text (or, all the text messages you sent in the last 10 years).
This breakthrough marks a huge step forward towards making AI more helpful for everyone, yet we often find that organizations are not fully utilizing long-context capabilities. It’s not uncommon for someone to ask me, “Is long context really useful? What can you actually do with it?” In many cases, leaders and executives need more guidance about the practical advantages of long context and, more crucially, why it matters when it comes to scaling gen AI.
In this column, I want to take a closer look at the value of long context and the top use cases it enables, along with some key things to consider when putting it to work in your own organization.
Unlocking more gen AI value with long context
Think of a context window like a model’s short-term memory; it can only remember a certain amount of new information at one time before it starts to “forget.” When the context window reaches its limit, a model will stop considering the earliest tokens to make room for new ones, which can impact the quality and accuracy of its responses.
This constraint is particularly significant for use cases that require working with enterprise datasets and documents or enabling lengthy, complex interactions. When a model isn’t able to consider an entire dataset or “remember” an entire conversation, it can lose critical context, leading to misinterpretations or gaps in reasoning. This can cause models to overlook important details or even hallucinate when generating a response. Imagine how difficult it would be to summarize an annual financial report from a publicly-traded company without the first 30 pages.
Organizations have adopted several strategies to handle these limitations, including techniques like retrieval augmented generation (RAG) to move data out of the context window. RAG is also commonly used to help ground models and add real-world context for more accurate and reliable responses. While these approaches are still relevant in certain cases, longer context windows can change how we leverage and interact with gen AI models due to some unique capabilities, including:
- Improved understanding and factual accuracy: Long context models can consider longer, more detailed and context-heavy inputs. This broader context provides them with a comprehensive understanding of the nuances and intricate relationships in complex topics, leading to more accurate and relevant responses. In addition, by considering and processing more information, these models are much less likely to generate factually incorrect information or hallucinations.
- Advanced in-context learning: Long context windows enable “many-shot” in-context learning, where a model can learn from hundreds or even thousands of training examples provided directly in the prompt. A many-shot approach can help adapt models to new tasks, such as translation or summarization, without the need for fine-tuning.
- Enhanced summarization and information retrieval: Long context models excel at synthesizing information, allowing them to analyze and summarize large corpuses of text. They are also able to efficiently locate and retrieve information from vast datasets, similar to RAG techniques, making them especially effective at question-answering tasks. Gemini 1.5 Pro, for instance, demonstrates near-perfect recall of finding specific information within context of up to one million tokens across text according to the “Needle in the Haystack” test.
Sophisticated workflows: Long context windows can also help to power more advanced and complex workflows that require deep understanding, reasoning, and longer memory. This is particularly significant for enabling AI agents and assistants, which need to maintain context and coherence over extended periods of time and multiple interactions.
Long context use cases
Long-context capabilities unlock a whole new set of use cases for organizations that were previously limited by smaller context windows.
The ability to handle large amounts of textual input has also enabled organizations to implement personalized AI assistants and agents that can maintain context over multiple, extended conversations, creating new opportunities for more personalized, relevant experiences for customers and employees.
We’ve seen customers using long context windows to generate personalized diet plans based on an individual patient’s entire medical history or analyzing thousands of social media videos and comments to extract the most common customer pain points. Long context windows also enable financial institutions to process lengthy, intricate documents like loan agreements, regulatory filings, and market research reports, leading to more accurate analysis and decision-making.
In addition, Gemini 1.5 models enable a number of multimodal use cases for long context, which all benefit from the ability to process text, video, audio, images, and code together at the same time. For example, these models can be used to gain more value and utility from multimodal inputs, enabling tasks like question and answering on video or podcast content, real-time transcription and translation, video captioning, and video customization.
A multimodal model with long context could scan an entire video library and identify relevant footage to create marketing or e-learning videos for a target audience. This is particularly important for manufacturers because Gemini models can analyze real-time sensor data to predict equipment failures before they occur, which can achieve new levels of efficiency, productivity, and innovation.
Already, we’ve heard some amazing stories from our customers, partners, and the Google community of how they’re using Gemini 1.5 Pro:
- A university professor leveraged it to extract data accurately from a three-thousand page document — in one shot.
- A financial services company is utilizing it for searching and analyzing all of its merge and acquisition documentation.
- A sports technology company is enabling its users to ask questions about videos captured with its AI-enabled cameras to evaluate plays or quickly pinpoint moments of interest.
- A startup founder used it to understand an entire codebase, identify the most urgent issue — and implement a fix.
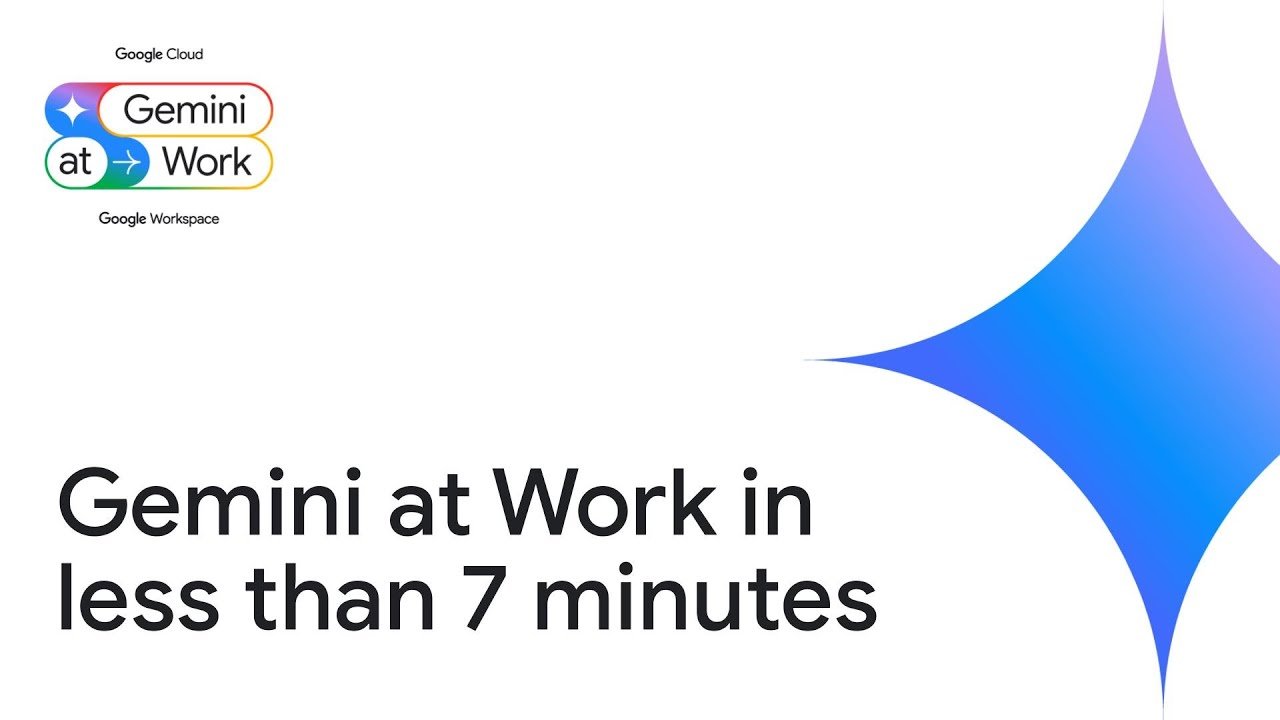
These are just a few prime examples of ways you can start thinking about using long context and what’s possible when you combine it with the multimodal reasoning and powerful performance of Gemini.
Key considerations for long context
Despite the exceptional capabilities enabled by long context, it’s not the ideal solution for all use cases. In scenarios where data gets frequently updated or requires pulling many different pieces of information from multiple sources of information, connecting a RAG system directly to gen AI models may still be more effective for retrieving accurate, factual information to generate responses.
Long-context queries also typically increase data processing times and demand more computational resources, which can potentially result in higher costs if not optimized effectively. Here, context caching is the primary optimization we recommend when working with long context to help reduce the latency and cost of requests, particularly with use cases that require passing the same information again and again to a model. Say, users are asking questions about the same information, such as a large amount of text, an audio file, or a video file. You could pass these items once and refer to the cached information to generate responses for subsequent questions.
In summary, it's clear that long context is reshaping the boundaries of what's possible with gen AI. While it's essential to consider factors like cost optimization and data freshness, the potential benefits of long context are undeniable. As you navigate your organization's generative AI journey, embracing this advancement will be important to unlocking its full potential and driving innovation.