How one bank is making fixed-income trading the new frontier in financial services innovation
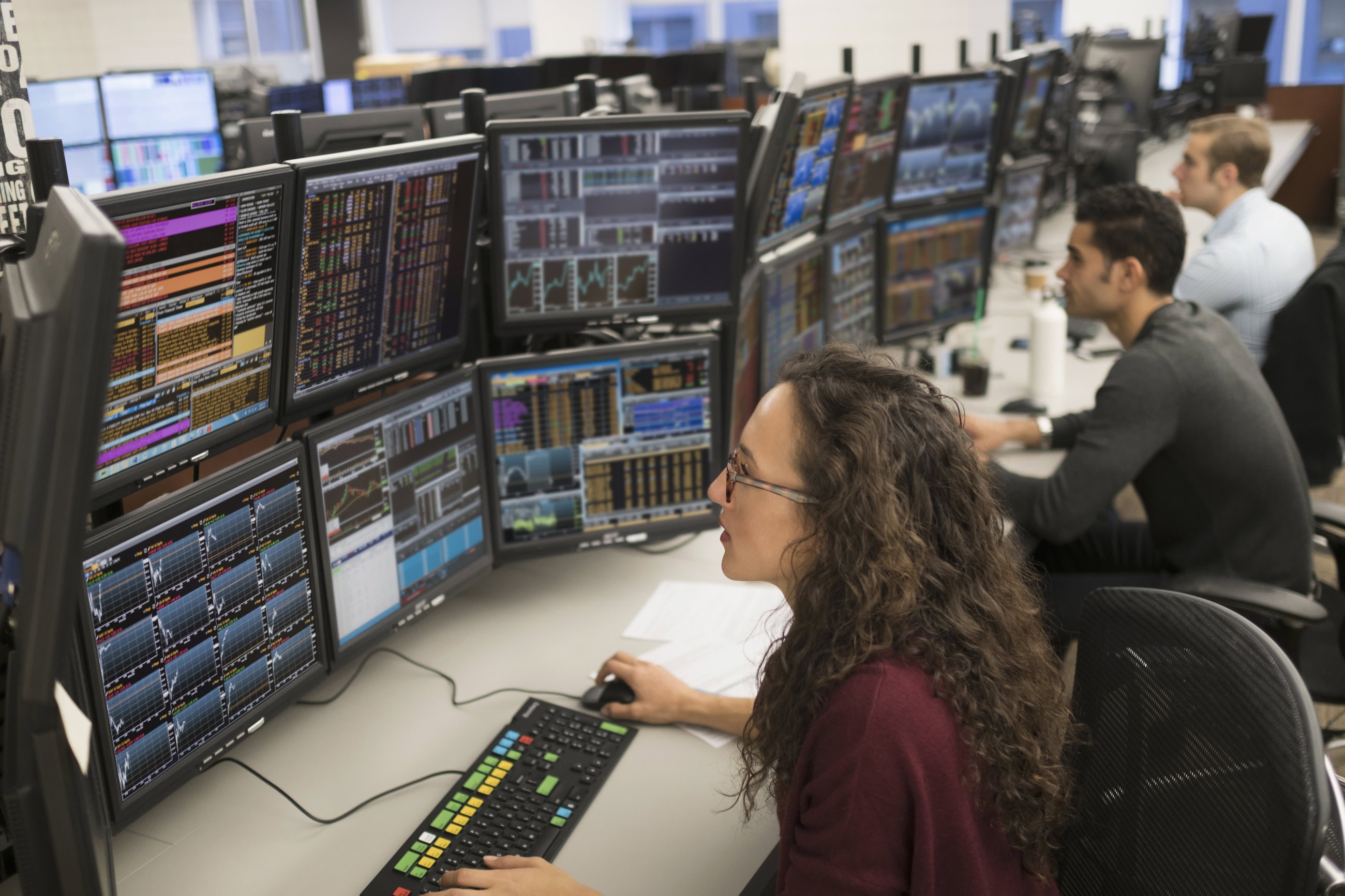
Matthew Schrager
Managing Director and Head of Automated Trading, TD Securities
Fixed-income trading has long resisted automation because of the complexity of investing in bonds. TDSAT is using cloud computing to change that equation.
Everyone knows about stocks. Automated, efficient – the domain of “flash boys” and hedge funds. In the world of financial markets, stocks were early to embrace automation and quantitative modeling, creating a hyper-efficient environment where opportunities flicker in and out of existence in much less than the blink of an eye. The smartest people in the world have spent decades building trading systems to squeeze every last molecule of efficiency out of the stock market.
That is to say: nowadays, stocks are boring.
Admittedly, I am biased. At TD Securities Automated Trading (TDSAT), we are devoted to a very different space, with very different challenges and opportunities.
At TDSAT, we trade fixed income.
For those unfamiliar with the term, when you hear fixed income, think of bonds. Yes, the boring certificates your grandparents probably bought for you when you were born. Except nowadays, they’re not so boring. In fact, fixed income in 2024 is one of the hottest spaces in all of finance.
If stocks were early adopters of technology, fixed income markets are late followers. Bonds have historically traded old school – over phones and chats, with humans on both sides of the transaction. This stems from inherent complexities that make these markets more difficult to automate than simpler instruments like stocks.
But that is changing rapidly. Fixed income is becoming the new frontier for automation in financial markets. And at TDSAT, with the help of Google Cloud, we’ve positioned ourselves at the forefront of that revolution.
TDSAT: Who are we, and how does Google Cloud fit in?
TD Securities Automated Trading is a subsidiary of TD Securities, based in Chicago. Our group got its start in 2013, at Headlands Technologies, a leading low-latency trading firm founded by former Citadel executives; we began with the goal of delivering world-class technology and quantitative modeling to fixed income markets. Our group joined TD Securities in 2021 as part of an acquisition to enhance the company’s fixed income business.
Since then, we have become one of the most active liquidity providers in the municipal bond market, with a growing presence in corporate bonds and plans to extend to many fixed income asset classes over time. We’ve achieved this success with a relentless dedication to technology, automation, and quantitative modeling.
Our partnership with Google Cloud has been instrumental in that success. Google Cloud’s offering has enabled us to construct a research platform that is one-of-a-kind in this space, with enough horsepower to drive the massive research workloads associated with our data-heavy approach.
At the same time, because of the scalability of the cloud compared to on-prem servers that are ours alone, we have the flexibility to pay for that high-performance infrastructure only when we need it.
Fixed income: unique challenges, unique solutions
As mentioned earlier, bond markets are complicated in ways that have historically made automation difficult. Many of these follow from the fact that there are simply far more fixed income instruments than stocks.
While a company generally has one associated stock, companies can issue hundreds of bonds, each unique. In extreme cases, like the municipal bond market, there are millions of unique securities, compared with just a few thousand liquid stocks.
Due to this fragmented structure, an individual bond has far less trading information than a typical stock. Associated datasets, therefore, are comparatively more fragmented and sparse. While an active stock may trade millions of times per day, a typical municipal bond trades only once every few weeks. Modeling techniques that work in the former environment simply do not apply in the latter. These differences have contributed to fixed income markets’ resistance to automation.
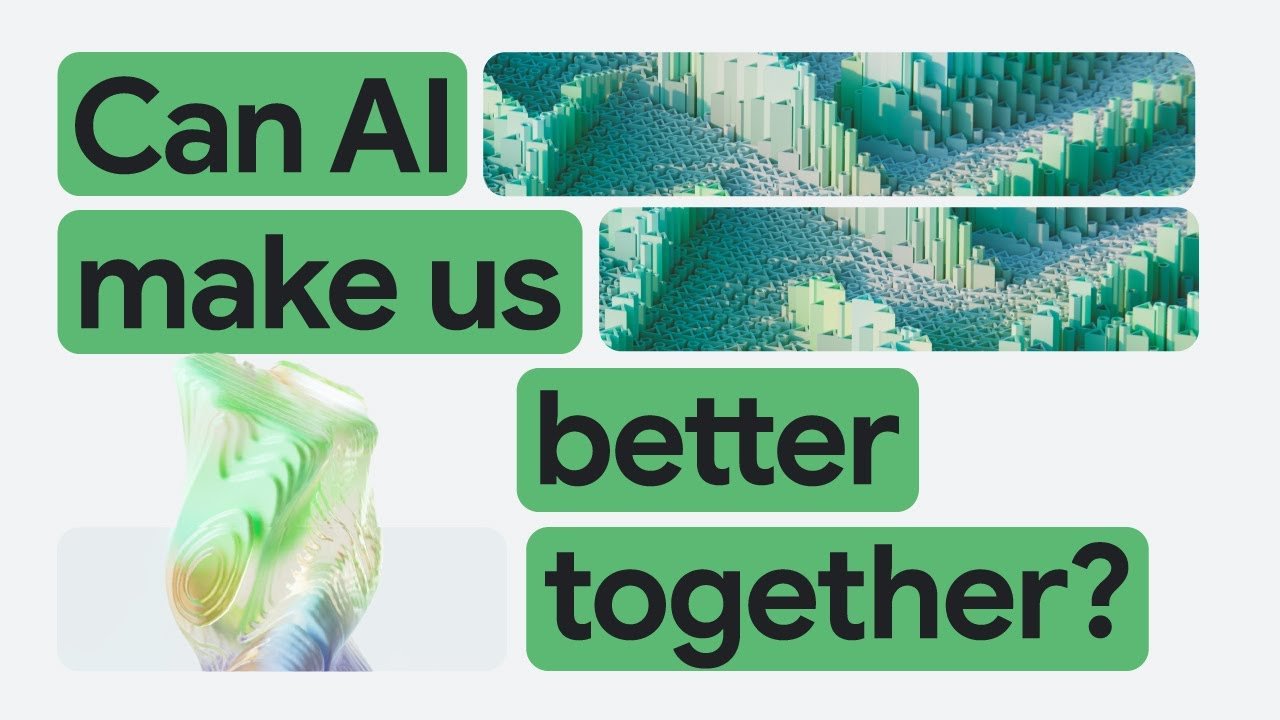
For example, in equities, trading strategies often exist on a per-instrument basis – one strategy for a big tech stock, a separate strategy for a large auto manufacturer or bioscience start-up, and so on. Computationally speaking, each strategy can be treated independently, increasing the parallelism of the problem space.
By contrast, due to the aforementioned dynamics, fixed income instruments generally lack sufficient data to support such an approach. Instead, fixed income strategies often involve learning over all instruments simultaneously. This reduces the degree to which the problem can be parallelized by several orders of magnitude, which has significant implications for algorithm selection, compute infrastructure, data organization, and more.
At TDSAT, our business was founded on the belief that these problems were tractable. But solving them would require addressing novel technical and quantitative challenges, with no map to guide us.
What it takes to automate fixed-income analysis
Our approach draws structurally from best-in-class techniques in low-latency trading, but with all the guts torn out and reinvented. It is highly quantitative and data-driven, using an array of statistical and machine learning techniques overlayed on a Google Cloud infrastructure, leveraging the horsepower to drive hundreds of thousands of concurrent research jobs at all hours of the day and night.
To understand why Google Cloud is such a powerful business enabler, let’s first outline the basics of our approach from a computational perspective. At the highest level of abstraction, our challenges include the following:
- Quantitative researchers run dozens of experiments per day.
- Each experiment is compute-intensive and requires thousands of concurrent cores.
- The overall workload is bursty and unpredictable.
- All experiments require fast access to large datasets.
This leads to a specific set of business requirements:
- Experiments are in the hot path of our researchers’ iteration cycle, so minimizing wall clock time of a single experiment is critical.
- Since we run many experiments simultaneously, and each experiment uses many cores, we need scalability to hundreds of thousands of cores, all with fast read access to large datasets.
- Since the overall workload is bursty, we need flexibility to scale the infrastructure up and down as required.
As we considered how best to build a platform to support these requirements, we were faced with the typical question: on-prem or cloud? We had to determine which approach best balanced the business requirements above against cost and time to market.
After analyzing Google Cloud’s technology, the choice was clear.
To begin with, an on-prem compute farm of sufficient scale would require an initial outlay of tens of millions of dollars, plus hundreds of thousands of dollars in annual support. It would also take time to build – we’d have to find a data center to house the servers, procure the hardware, hire ops staff, wire it all up, etc. Suffice it to say, the upfront costs of the on-prem approach were high.
By contrast, a Google Cloud-based infrastructure allowed us to quickly build a most-viable product at minimal expense, and then seamlessly scale it as the business grew. We were able to focus on the application layer on day 1, knowing the hardware resources were available whenever we needed them. This dramatically reduced time-to-market – essential in a fast-moving industry like trading.
Many specific Google Cloud features made the platform well-suited to our task. The massive internal bandwidth between Google Cloud Storage and Google Cloud Virtual Machines allow us to access large datasets quickly across hundreds of thousands of cores. Flexible VM types let us optimize for our compute-heavy workload, for example by using Compute Engine C2 instances when available. Preemptible instances allow us to optimize for cost in cases where the workload can sustain a loss of subsets of VMs. Google Kubernetes Engine lets us orchestrate this substantial infrastructure with unparalleled stability at scale.
And perhaps most importantly, Google Cloud offered true collaboration instead of just treating us like another revenue stream, offering copious resources and educational materials to make our integration as seamless as possible.
Changing the game
The results of our investment in this platform have been remarkable. Since its completion, our business has grown tremendously in terms of trading volumes, portfolio size, and profitability. Most importantly, the platform allows us to optimally serve our clients by providing competitive pricing on hundreds of thousands of fixed income securities, day in and day out.
Today, TDSAT is one of the most active algorithmic bond trading groups in the world. And we owe part of that success to our partnership with Google Cloud.
The future of fixed income is bright. Over time, more firms will embrace automation, leading to greater market efficiency and better outcomes for investors. At TDSAT, we are excited to be part of that journey, and thankful for Google Cloud’s help in becoming an important figure in the story.