Five questions every executive should ask themselves about gen AI

Donna Schut
Head of Technical Solutions, Applied AI Engineering
Hamidou Dia
Vice President, Applied AI Engineering
Generative AI has proven its value in the past year. However, the rapid evolution of this technology requires executives to strategically plan for the future, and we've identified five key questions to guide that process.
Over the past year, the value of generative AI has become evident. Organizations have adopted gen AI to improve customer experiences and for a wide range of internal productivity use cases, such as internal helpdesk, developer efficiency, procurement, contract management, sales and marketing. These are great use cases to drive immediate impact, yet in a quickly evolving space, it’s difficult to stay on top of what is next.
In our roles, we’re fortunate to speak with executives around the world about their gen AI strategy and work with their teams to deploy gen AI applications. Based on our engagements, our own internal development, and seeing what has worked – and what hasn’t – we’ve distilled five key questions that every executive should ask as they’re formulating their strategy for gen AI next year.
- How do we select and evaluate gen AI projects?
The first question leaders embarking on their journey will ask is how to identify and select gen AI projects. We’ve seen most organizations start with internal, low-risk use cases that address pain points in lines of businesses – starting with a small group of users for feedback and iteration, before expanding more broadly.
Critical in bringing gen AI projects to success, is the ability to measure impact. Ultimately, it comes down to understanding the business impact of gen AI projects – and setting the wrong metric can do more harm than good. While this may take longer to set up, we encourage customers to do this as the foundation of gen AI application and product success.
For example, to measure the impact of gen AI for customer service, one may think time-to- resolution is a good metric. However, in this scenario, optimizing for time to resolution may mean that the lots of returns are approved, resulting in cases being closed much more quickly, but ultimately, driving losses for the business. Therefore, a better metric may be customer lifetime value, which accounts for customer satisfaction and sales.
Therefore, we recommend teams define metrics that align with the business goals, thinking through how they can be measured and, how they can potentially result in harmful behaviors or wrong prioritization. In cases where measuring the long-term impact isn’t possible, teams will need to define surrogate metrics as a proxy for this. In addition to these metrics, it’s important to think of evaluation along the development lifecycle and at different levels of the technical stack – from models to systems.
2. What technical capabilities should we invest in?
While gen AI can provide intuitive experiences, create new content, and aid in solving tasks, it’s worth considering which problems these technologies are best suited for. For example, deterministic problems may be better solved by rules-based systems. Similarly, agentic workflows are well suited for tasks that require planning, reasoning and tool use, but not a recommendation engine, which can be solved by classical machine learning methods. Determining the right techniques for each business use case will yield a better return on investment.
As organizations move from experimentation and evaluation to production deployments of gen AI applications, there will be a need for more advanced system design. This entails building systems that orchestrate multiple prompts and agents with various frameworks, how to do evaluation and observability for them, and how to put these multi-agent systems into production.
To productionize and operationalize gen AI applications at scale in an organization, it’s critical to invest in MLOps capabilities, and accompanying skills for gen AI. These include: data curation, artifact management, model adaptation and optimization, chaining and reasoning with tool use, grounding, guardrails, observability and monitoring. As this is a fast- moving field, investment in technical capabilities is ongoing and we look forward to working with customers to explore new model adaptation methods and the ML engineering challenges as this field evolves.
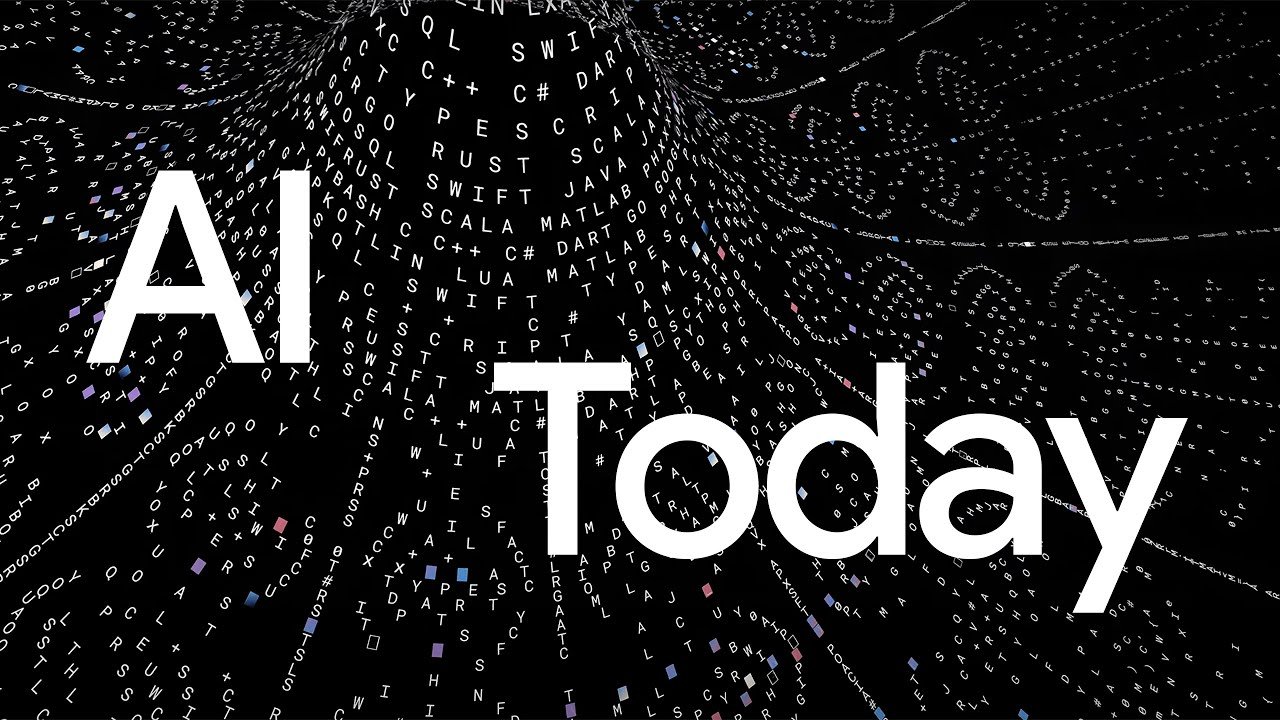
3. How do we empower teams to be successful with gen AI?
One of the blockers of gen AI adoption is the lack of skill set. Organizations we’ve worked with that have successfully driven change, identified a team of pioneers that were willing to experiment with new technologies and spearhead new initiatives. Based on their results, others followed suit.
While hiring gen AI experts may be challenging, working with partners is another way to develop hands-on skills by working side by side. This not only accelerates the time to market, but also allows for practical training of employees that are motivated to learn, while benefiting from partner domain knowledge on the nuances of gen AI application development.
To successfully upskill teams in generative AI, organizations should adopt a multi-faceted approach. This involves providing a combination of formal training programs, hands-on workshops, and access to AI experts or mentors. To support all of these efforts, having a culture with psychological safety and culture of experimentation is critical. Account for more time to experiment with gen AI to find the right solution than with traditional technology; don’t commit to an approach too early and instead, aim to fail fast and get a proof point early.
Organizations we’ve worked with that have successfully driven change with gen AI, identified a team of pioneers that were willing to experiment with new technologies and spearhead new initiatives. Based on their results, others followed suit.
Hamidou Dia, Vice President of Applied AI Engineering, Google Cloud
4. How do we manage risk?
In addition to the legal, regulatory and ethical compliance aspects of gen AI, organizations also need to think through responsible AI use internally. Whereas previously AI was confined to a central team of Data Scientists, gen AI is now more accessible to many teams across lines of businesses. While this generates more value, it also poses more risk, and with any new technology, evolving capabilities can create potential risks and complexities such as toxicity, hallucination, and new security threats.
This has given rise to new questions around the best way to build fairness, interpretability, privacy, and safety into these systems. While the general software engineering practices still apply, there are a number of unique considerations for AI systems such as using a human-centered design approach, understanding limitations of your data, model and system, analyzing performance and continuous monitoring.
Additionally, data governance is critical for building successful gen AI applications because it ensures data quality, reliability, and ethical use. By establishing clear policies, procedures, and accountability for data management, organizations can help mitigate risks associated with inaccurate, biased, or sensitive information. This foundation of trust in data enables gen AI applications to produce more accurate, relevant, and responsible outputs by grounding them. We also see customers investing in their own compliance and policy checks using LLMs.
5. What is the long-term transformation for my industry?
Looking further out beyond the initial use cases that deliver business value, we need to consider the long-term impact on industries. We envision a world where agents work, with other agents, in an assistive capacity with, and on behalf of, employees. To power this new future, leaders will need to think through management structures that can be translated into agentic workflows, defining task-level performance. We’ve seen the biggest step change in rethinking end-to-end workflows, rather than focusing on narrow use cases. As a result, business models and industries will evolve, and we look forward to working with organizations on their transformations with new and advanced technologies as they do.
Learn more about gen AI use cases and solutions from our team, here.