How Isomorphic Labs uses AI models to explore new frontiers in drug discovery

Sergei Yakneen
Chief Technology Officer, Isomorphic Labs
Shweta Maniar
Global Director, Healthcare and Life Sciences, Google Cloud
Using AI to reduce the experimental burden and accelerate the identification of potential treatments paves the way for faster, more efficient development of new treatments and cures.
Even with the advanced pace of scientific discovery, there’s still so much we don’t understand about how human biology and chemistry work. That’s why drug discovery, which relies heavily on lab experimentation, takes so long, costs so much, and fails too often.
Isomorphic Labs, an Alphabet company and Google Cloud partner, is harnessing the modelling capabilities of machine learning to unravel the mysteries of biology and chemistry so that researchers can accelerate the discovery of new medicines. They’re building a platform based on the premise that biology is akin to an information processing system: an infrastructure that transmits information. And if that’s the case, then machine learning models should be able to decipher the complex principles of biology and thus identify promising molecules much faster than traditional experimentation.
Isomorphic Labs is developing cutting-edge computational techniques to tackle not only some of the hardest problems in drug discovery, but also some of the toughest challenges in biology, chemistry, and medicine. It's exciting that Google Cloud can support this vision, providing the computational power and flexible, scalable infrastructure that are enabling Isomorphic Labs to reimagine scientific research.
In this post, Sergei Yakneen, chief technology officer of Isomorphic Labs, shares his insights into the enormous potential of applying machine learning to life sciences, based on his two decades of experience in scientific research, engineering, and artificial intelligence. He explains how reducing the experimental burden and accelerating the identification of potential treatments pave the way for faster, more efficient development of new treatments and cures. - Shweta Maniar, global director, Google Cloud Healthcare and Life Sciences
Nearly all diseases are linked to some molecular mechanism gone awry.
An infectious agent may get into the body and disrupt or co-opt its machinery. Or in the case of cancer and other genetic diseases, certain mechanisms within our cells, such as DNA damage repair or programmed cell death, break down. Proteins are complex molecules that do much of the work inside our cells. Because their role is so important, identifying the right protein and finding molecules that interact with it to change or treat the disease condition are common goals of drug discovery.
There is an infinite number of possible molecules to consider. Even if one constrains the possibilities to molecules that are similar to other known drugs, the number is still astronomically large at 1060. This makes the search for the best molecule to solve your problem extremely complex, frustrating, long, and expensive.
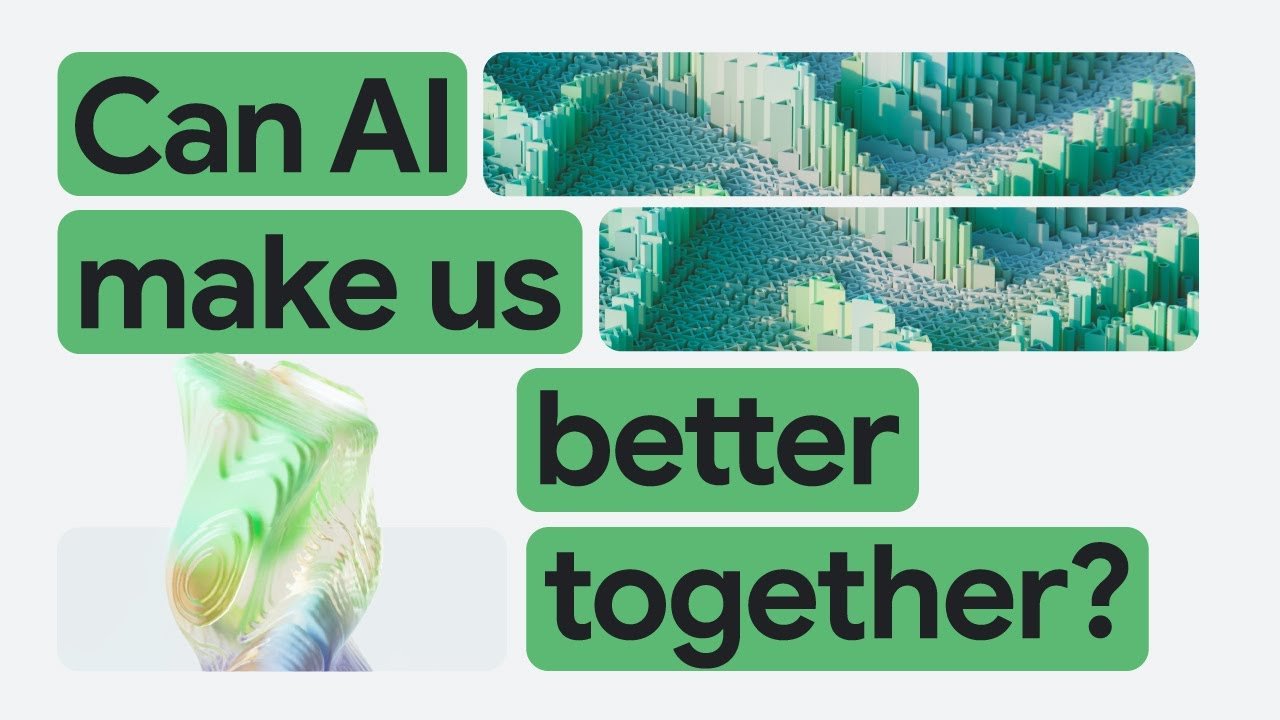
Hear from Google Cloud customers about the new way to cloud.
Humans generally struggle to accurately predict what properties a molecule will have just by looking at its formula. Instead, the drug discovery process relies heavily on lab experiments — which can take 10 to 13 years and USD 3 billion on average to bring a new drug to market.
The more we can narrow down the search to promising candidate molecules, the faster we can find ones that may be effective new medicines. This means narrowing the astronomic number of drug candidates down to only those that bind to a desired protein target, that are non-toxic, that get absorbed by the right tissue, are small enough to enter the intended cells, and get safely and timely flushed out of our bodies; identifying molecules that work is often not the problem, is mitigating these side effects and unintended consequences for the body.
AI is getting us to the right candidates in record time.
Research is doing something nobody's ever done before
Isomorphic is Greek for “same shape,” which speaks to the parallel between computational models and life itself. The premise of our company is to build machine learning models that accurately represent the fundamentals of biology and chemistry. With the assistance of these models, we'll be able to remove much of the experimental work from the equation of drug discovery and conduct the search for new medicines in-silco (through virtual computing environments) in a really rapid and efficient way.
The best-known example of such a machine learning system is AlphaFold3, co-developed by Google DeepMind and Isomorphic Labs, and recently published in the journal Nature. The latest in a series of AlphaFold protein models, it has learned how to very accurately predict the 3D structure, or shape, of proteins, of nucleic acids such as DNA and RNA, and of small molecules — only using their sequences as input.
AlphaFold is a great example of using a machine learning algorithm to understand a fundamental principle of biology and provides tremendous value on the road to designing better medicines. At the same time, there’s much left to solve, and we’re actively working on tackling many other problems so we can perform drug discovery in a dramatically improved way.
There are major questions we need to answer to succeed in drug discovery, including:
- Which protein should we target with our drug design program?
- Where on this target can a therapeutic compound bind to achieve the desired effect?
- Will a given molecule bind tightly enough?
- Will the molecule go where it’s supposed to go within the body?
- How long will it take the body to break the molecule down, and will it be able to do so in a safe manner?
When dealing with these questions, human experts often adhere to familiar patterns based on their own experience, design programs they’ve participated in, or already approved drug molecules. This is oftentimes helpful, but can also be limiting when exploring the vast possibilities of new molecules. Our computational models are free to explore the entirety of chemical space. Based on their learned representation of chemistry, they can efficiently suggest completely novel drug candidates that have all of the desired properties — ones that humans might never conjure up — while doing so in a small fraction of the time.
Can computing systems decipher the fabric of our health?
Modern scientific research, especially when it involves the development of machine-learning models, can be incredibly complex and compute intensive. One global research project I worked on from 2015 to 2019, the Pan-Cancer Analysis of Whole Genomes, needed more than three years to analyse the genomes of nearly 3,000 cancer patients using the technology available to us then.
Thankfully, more and more computing power and more advanced technology has become available each year to help us solve some of the toughest problems of biology and chemistry. AI and machine learning is at the heart of everything we do at Isomorphic Labs, and no one has embraced this field to the extent Google Cloud has. It was an obvious choice on which to develop our work.
AI is getting us to the right candidates in record time.
Isomorphic Labs has embraced Google Cloud’s planet-scale computational power to boost the development of our AI-driven drug discovery platform since the start. Cloud-based AI infrastructure can scale rapidly and readily to suit our needs both in research and production.
If we want to ingest and store a very large data set, perform various exploratory analyses, design a training and evaluation suite and feed it into our machine-learning algorithms, the cloud is there to meet all our needs. It’s no problem to implement highly scalable machine learning models using thousands of graphics accelerators. Google Cloud provides an infrastructure layer we can trust, so we can focus on the research and engineering behind our platform.
Laying the foundation for accelerated discoveries
At Isomorphic Labs, we’re already making massive improvements to drug discovery. Instead of developing narrow solutions for a specific disease, we’re working to understand core aspects of biology and chemistry, and have developed a general platform that we can apply across all disease areas. This type of scalability is what makes technology so powerful.
For example, we’ve collected and uploaded a large and varied dataset to Google Cloud, where our AI researchers continuously train a wide variety of cutting edge machine learning models. In production, our team of medicinal chemists uses a large fleet of Google Cloud graphics accelerators to run the models in inference mode. Our scientists can accurately screen massive compound libraries in-silico to identify potential hits, then run large-scale biophysics simulations on Google Cloud’s high-performance computing clusters, and visualise these results in our platform to further advance our drug discovery programs.
Over the past two and a half years, we’ve built an incredibly powerful AI platform that really moves the needle on drug discovery. As these models continue to improve, we’ll reduce the level of experimentation necessary to design new medicines.
Quality and safety are of utmost importance when it comes to health, and we'll continue to rely on experimental results as we take our molecules through clinical trials. But we’ve already made giant leaps in our ability to rationally and efficiently design novel medicines with the power of AI and the cloud.