AI 2030: What to expect, and five areas for investment, from our head of AI
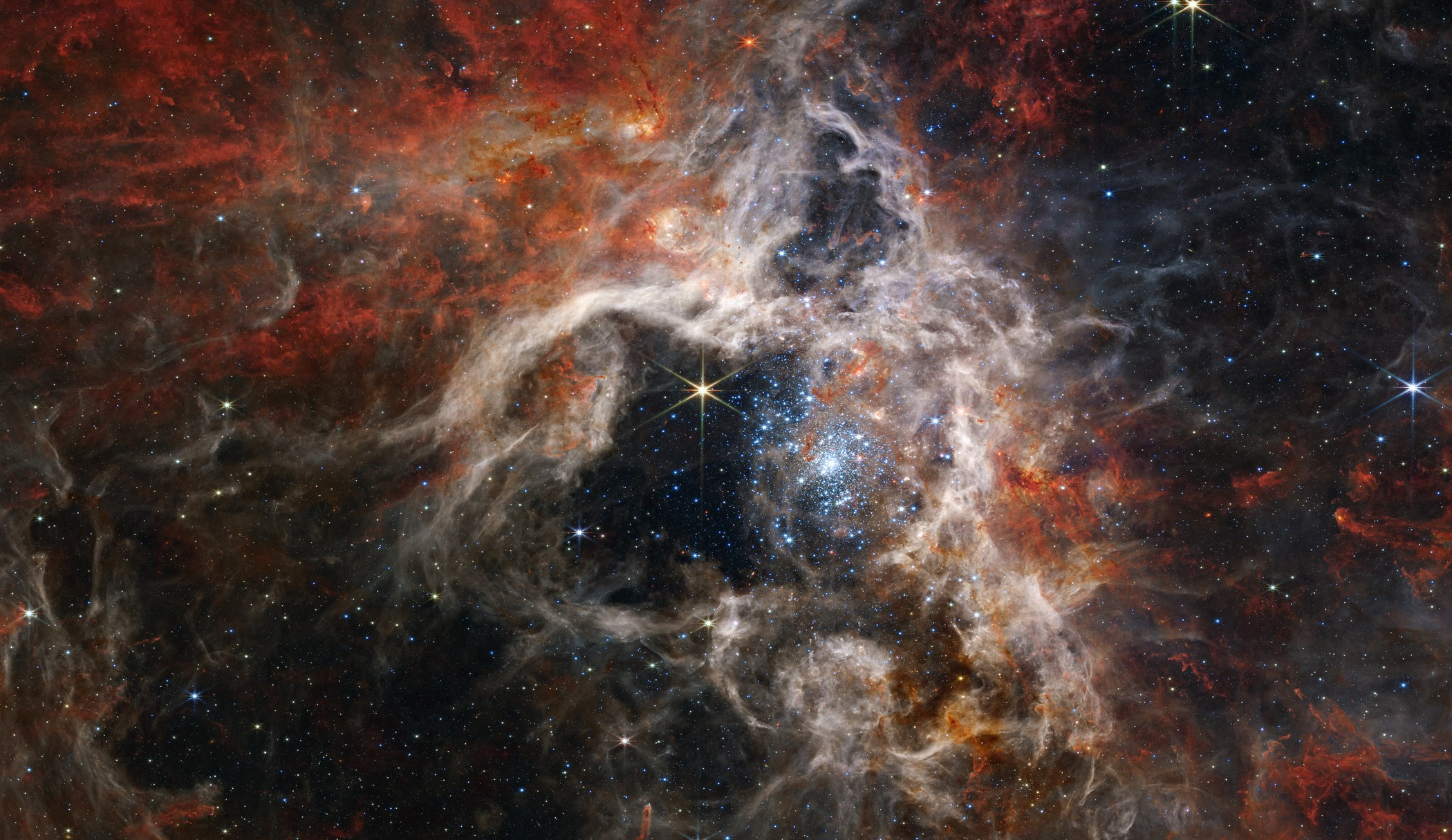
Andrew Moore
Vice President & General Manager: Cloud AI & Industry Solutions
Andrew Moore is the head of AI at Google Cloud and the former dean of the Carnegie Mellon School of Engineering in Pittsburgh, where he has been at work on the big questions of AI for more than 20 years. Here he shares his vision for some of what we can expect over the next 10.
When people ask me what AI is likely to look like in the near future, I often answer with the following theoretical example:
Suppose you’re about to leave for a business trip and decide at the last minute to bring your child for some sightseeing. Doing that today would likely involve so many logistical tasks that you’d practically need a personal assistant to pull it all together.
But soon, you may be able to have the following conversation with a nearby device:
“Hey, Personal Assistant, please connect me with Sunflower Airlines.”
“Sure, Andrew”
“Hi, this is the Sunflower AI. Nice to talk to you, Andrew. Is this about your flight to Bournemouth tomorrow?”
“Yep, I’d like to bring Joey with me”
“Nice. I’ll see how to make that happen. Do I have permission to consult with your hotel and your rental car’s AIs?”
“Sure.”
(Nine seconds pass.)
“OK, we have a plan. It will be $340 extra all told, and we have you sitting together on all the flights, and we changed the hotel room. Your car doesn’t need to change, but we’ll make sure there’s a toddler seat. Does that make sense?”
“Yes, thanks!”
“No problem. Shall we discuss sightseeing options later in the taxi?”
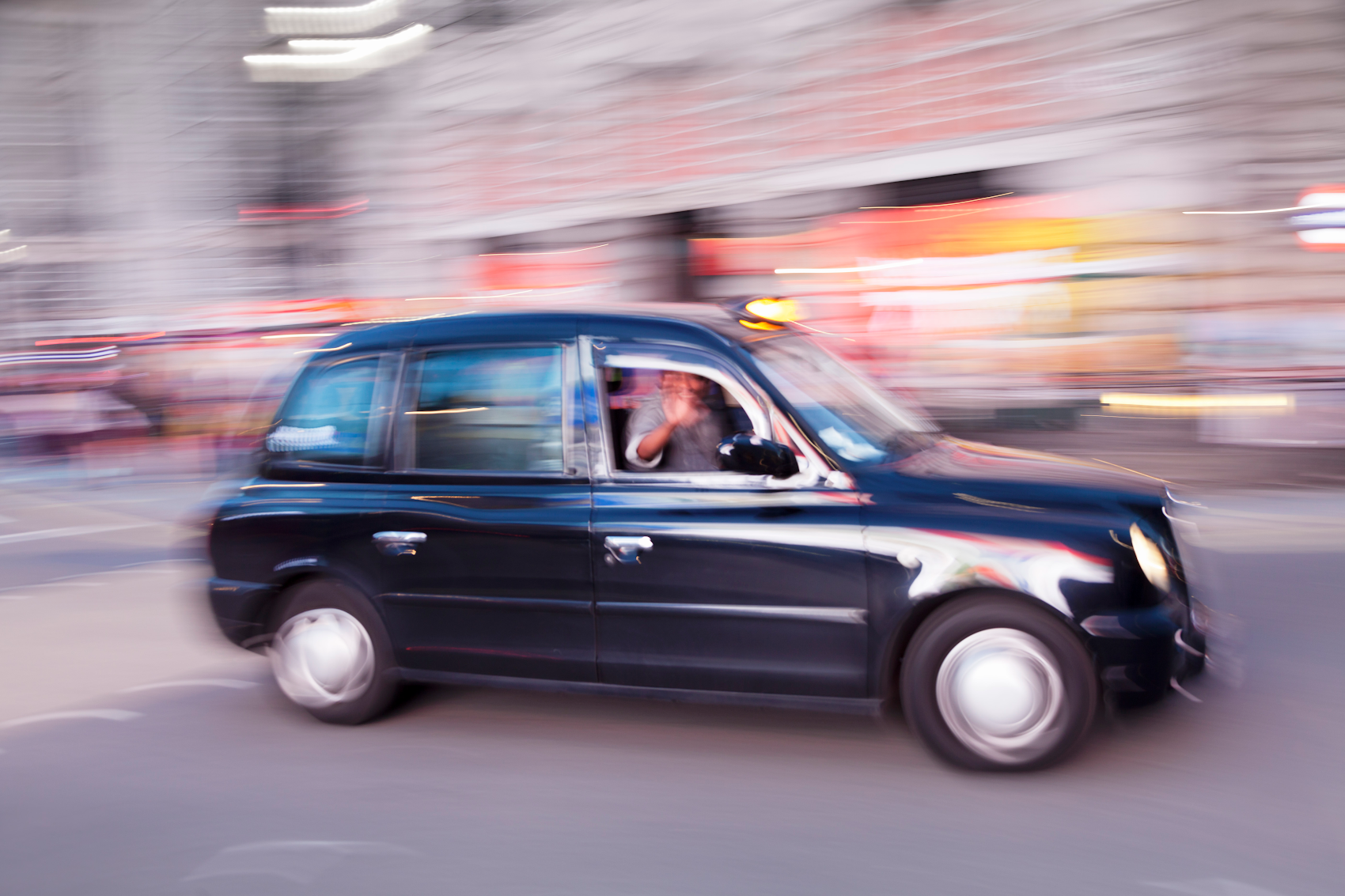
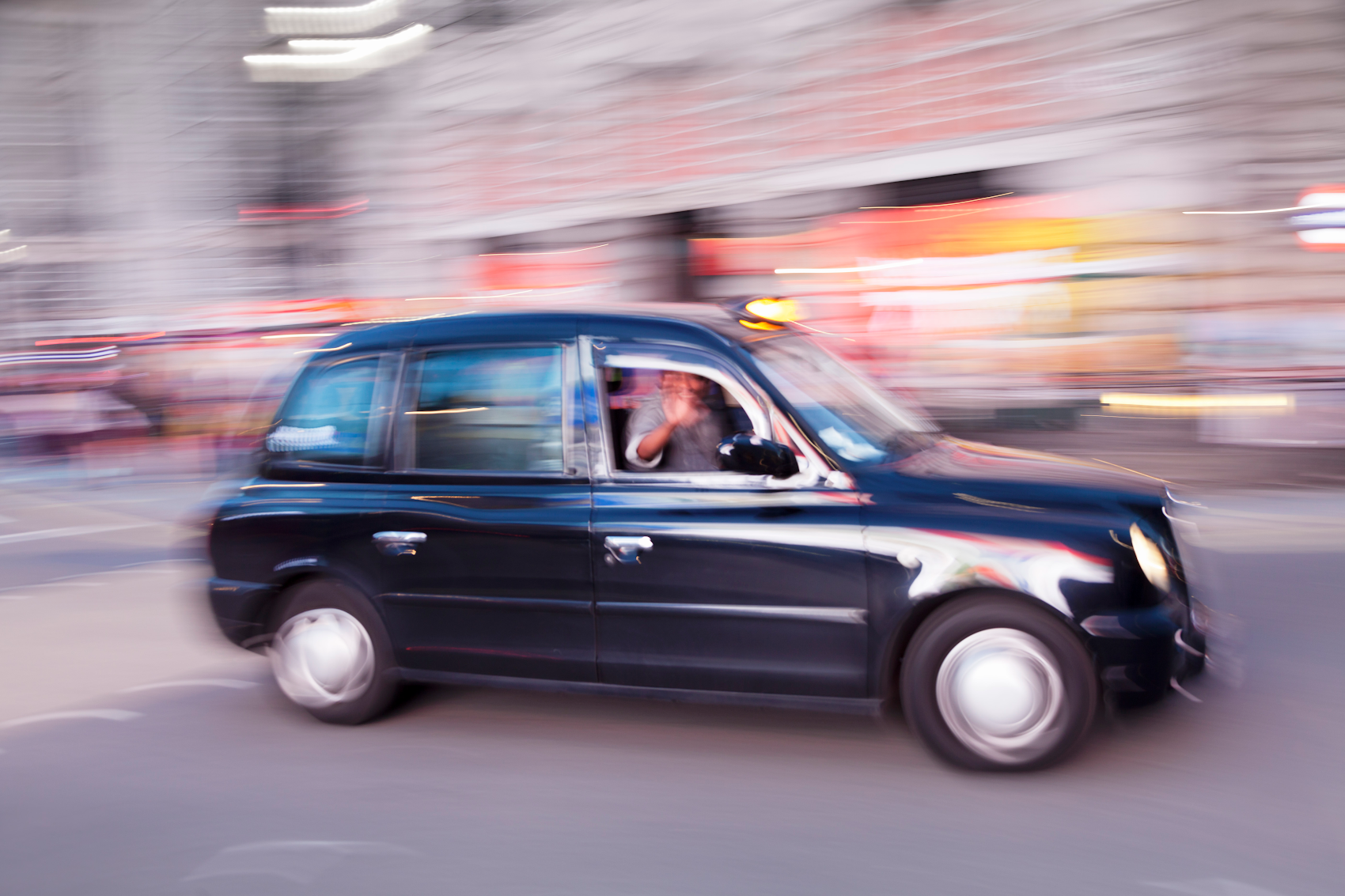
To be clear, this use case is theoretical, so don’t interpret it as a product roadmap for Google or any other provider. But it is a reasonable extrapolation of where AI technologies are headed and what kind of broad experiences may soon be possible, with virtually anyone able to enjoy concierge-like services available today to only a few.
To prepare for a likely future of ubiquitous and helpful AI, enterprise leaders should visualize progressions like the above. Leaders should consider what these progressions imply about experiences that keep pace with customer expectations. They should think about how AI use cases that seem discrete today may converge, putting increasingly intricate blends of AI behind simple user interfaces. Perhaps most of all, they should imagine the IT agility this future (or most any future of continued technological disruption) may require.
Something I really want to stress is that the dialog management — or machines being able to understand requests and respond accordingly — is not the hard part. We do most of that today with services like Contact Center AI. The important part is the decision-making and action-taking smarts an AI will require to near-instantly 1) understand the context of a request; 2) nimbly make changes to plans, and; 3) provide the convenience with minimal cost and carbon impacts. We’ll summarize this AI role as problem solving.
Why AI uses cases increasingly converge around problem solving
Managing schedules and reservations is just the tip of the iceberg. To see the broader range of possibilities, we need to recognize that in our initial example, a lot of AIs are involved, but they’re structured around the central theme of understanding human end-user needs and brokering those needs with backend transactional systems.
The notion of using AI to turn audio into text is not new, but conversational AI goes much further by not only accurately transcribing spoken words but also interpreting their meanings. One familiar comparison — outside of speech technologies but still broadly within the realm of conversational AI — is search engines: older approaches matched words in queries with words in web pages but newer models are much better at both understanding the intent of a query and either diagnosing what the end user really needs or, if not confident about what that need is, composing a follow-up question.
Accurate transcription from speech to problem statement enables a low-friction, intuitive interaction model in which people don’t need to learn a series of steps or use pre-defined commands to get to their desired outcomes. These technologies also abstract the increasing complexity on the backend, where spoken statements are invisibly handed off to other AIs (invisibly, as far as the user is concerned) to address a task or problem.
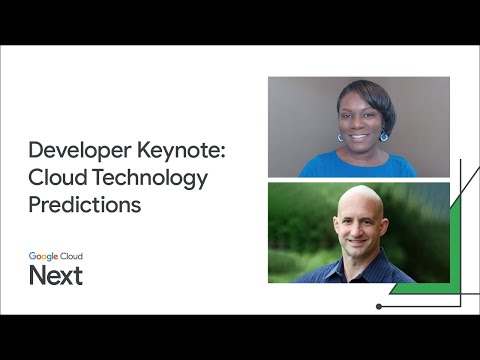
Google Cloud's Top 10 Cloud Predictions from Next '22
For example, if someone makes a simple ask of a digital assistant — “Will I be able to swing by the pharmacy to get my medicine after work?” — the backend AIs would need to undertake a series of near-instantaneous tasks. It would start by securely relating spoken words or phrases to a Knowledge Graph of real-world objects and pieces of information: which specific pharmacy the user wants, what the pharmacy’s hours are, how long it will take to get there if the user leaves work at the usual time, and so on. Similarly, if someone asks, “What’s that suspense movie set in San Francisco in which a main character wears a green dress?” then the frontend AI might pass the baton to a problem solving AI that assesses movie descriptions and reviews, or that leverages vision AI to analyze images in films.
In all these scenarios, the “problem solver” is foundational AI technology to which other AIs are attached, keeping the user experience straightforward even as use cases become exponentially more elaborate and wide-reaching. It is not hard to imagine AI assistants that not only serve as concierges, as in the examples posed here, but even guardians (such as first responders using AI to coordinate during an emergency) or meaning makers (such as researchers trying to wrangle large datasets involving both structured and unstructured information).
Investing for an AI-driven future
The field of problem solving AI is maturing fast, as are the backend technologies that make conversational interfaces such a potentially profound evolution in human-computer interaction. The companies best positioned for an AI-fueled future will be those with the foresight to act before the tides turn, not when the wave finally crashes upon them.
As this article suggests, competency in conversational AI and connections to your backends are rapidly becoming foundational and should be a top consideration within your company’s vision — but that vision should also include capabilities to turn this foundation into diverse and helpful use cases.
To prepare your business, I recommend prioritizing the following:
Use Case-Driven AI Research
Research is by far the largest expense in the development of useful, sustainable AI models. It involves not just the cost of designing and building infrastructure but also the demands of refining models for accuracy or to avoid bias, and the process of translating abstract technology experiments into enterprise-grade services.
Pure research, without an eye toward real-world use and productization, can be akin to burning money, while product-focused development that isn’t vetted through research is unlikely to produce sharp, accurate models that are useful over time. When you can achieve research directed to the most common problems for your customers, such investments of not only resources but also time become incredibly valuable.
As organizations continue to adopt AI as a core part of how they do business, they must prepare for these massive investments themselves or carefully select technology providers whose robust research practices can keep their organizations at the forefront.
Data harmony and interoperability
Even if AIs can understand language and relate it to the real world, insights and solutions often come from data that can be hidden away in sources and silos. This means the ability to unify and clean datasets is a prerequisite to applying AI.
In fact, one of the virtues of problem-solving AI is that disparate data can produce richer insights, since the people who siloed off that information in the first place weren’t considering potential relationships. Machine learning suffers from no such pre-existing biases. A company can build a data management foundation without building any AIs, but no one can build a useful AI without investing in their data substrate.
Responsible and explainable AI
If your AIs are useful, they are allowing you to improve your users’ experiences in an economically sustainable way. But this improvement of experience comes with a serious risk: There are dozens of ways that the improvements might disproportionately affect different populations of customers. No one wants to launch an AI that damages vulnerable populations, and, in addition to the moral issue, there is a growing body of regulation that such systems must be tested for such vulnerability.
This is why the ability to diagnose the behavior of an AI has to happen during design stages, not as an afterthought. I am finding that in an increasing number of AI applications involving decisions on behalf of end users, I and my engineers are abandoning so-called ”black box” algorithms such as neural networks in favor of the new generations of predictive models that can be much more easily interpreted by trained human ML engineers.
Sustainable AI practices involve virtuous, perpetual cycles: Data is required to create AI models, those models create data of their own that should be harnessed for future research and model refinement, new data sources may replace old ones due to various disruptions (such as the macro-economic shifts during COVID-19 that rendered most pre-pandemic data useless for projections), and so on. Without insight into how the models work and are iterated over time, and without a framework for acting on those insights and securing underlying data, organizations will struggle to turn their AI investments into business assets that are viable over the long term.
Democratization
The ability to inject AI into workflows or understand AI-based tools cannot be confined to those with data science and programming skills — it needs to be accessible to everyone. Some of this journey involves tools that use simpler interfaces to abstract the complexity of building AI-based apps. No-code platforms and APIs such as the Google Cloud Speech API facilitate some of this, but some of it is also an extension of creating AI research practices and investing in responsible, explainable AI. Without the cultural socialization within a company, democratized tools help only so many and only so much.
Multicloud
AI becomes scalable when it can be replicated without restriction across clouds or combined with technologies across cloud providers and your company’s proprietary internal projects.
If your business is tied to a single provider, all the intellectual property your organization develops (the AI or ML models, analytics, the processes, the rules, the applications, and even the database) are locked in and dependent on your cloud provider’s infrastructure and products. This makes it extremely difficult to migrate your intellectual property from one service provider to another. Starting with a hybrid or multicloud approach on day one can be vital to the long-term success of your AI strategy and investments.
The future of AI is being built now
This moment in AI is one of the most exciting I’ve experienced in my three decades in the field — not just for how we engage with machines but also the tasks we’re increasingly able and comfortable entrusting to them.
This evolution is possible because so many AI technologies are converging behind single points of entry, like conversational AI, to perform progressively more sophisticated and elaborate tasks based on increasingly natural and intuitive user inputs.
Opening image courtesy NASA, ESA, CSA, STScI, Webb ERO Production Team, via Creative Commons and Flickr.