Research’s next revolution: Getting fast answers from the life science data universe
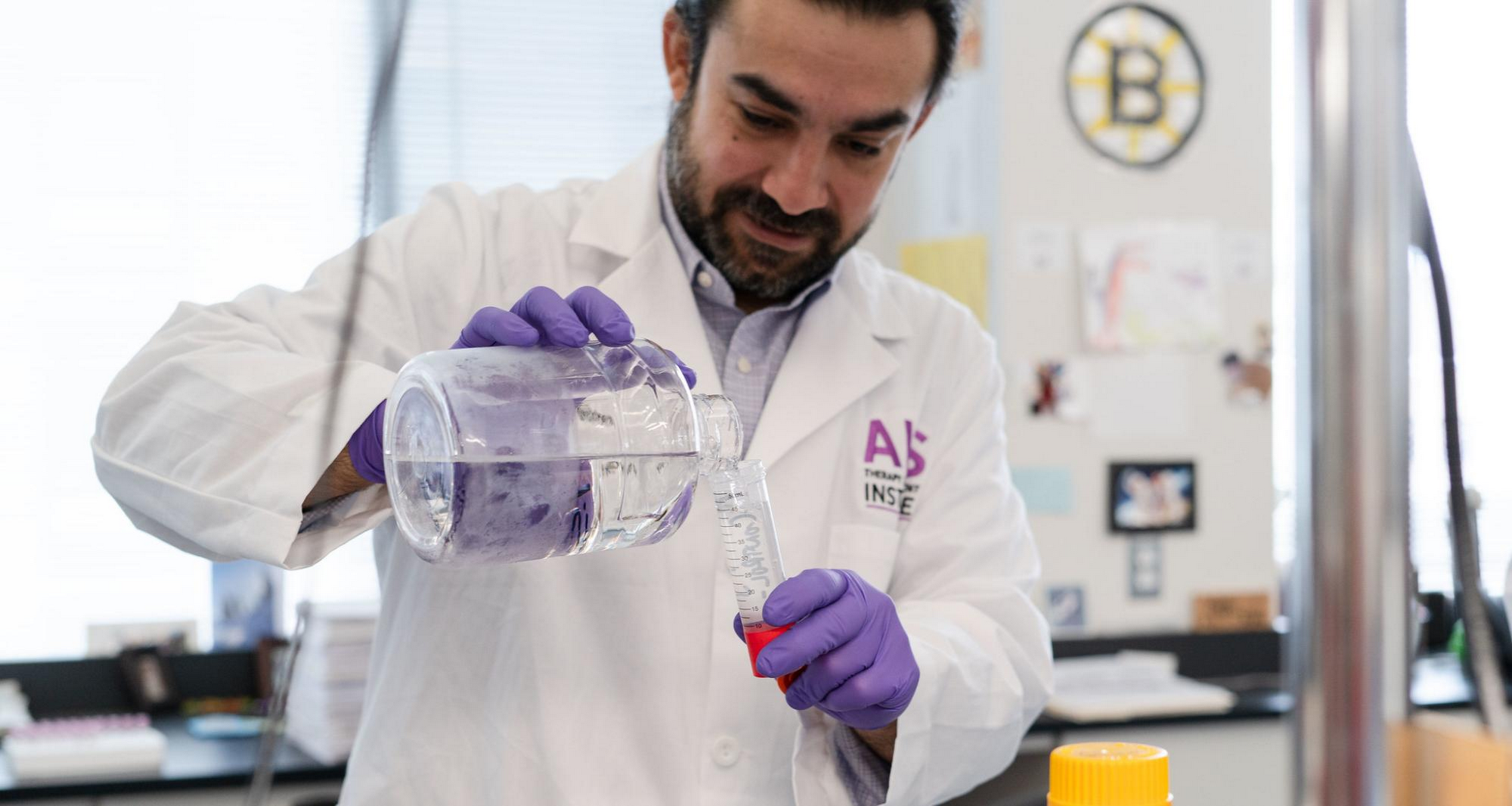
Shweta Maniar
Director of Industry Solutions for Healthcare & Life Sciences, Google Cloud
Fernando Vieira
CEO and Chief Scientific Officer, ALS TDI
Cloud computing is helping researchers unlock the mysteries of ALS and other neurodegenerative diseases
When was the last time you assembled a thousand-piece jigsaw puzzle? Did you feel tantalizing anticipation as the puzzle image started to reveal itself and exhilaration as you snapped the final piece into place? Now imagine thousands of puzzles, each with a thousand pieces. Someone has dumped all the pieces into a bin and thrown the box tops away — and one of the puzzles contains the key to saving lives.
This is the unfathomable dilemma facing healthcare and life sciences researchers. While there’s certainly no shortage of healthcare data or ways to collect it, organizing all that data and deriving answers from it — fast enough to make a difference — is an incredible challenge.
At Google Cloud, we know firsthand how challenging it is to bring massive amounts of data together, make sense of it, and unlock its value in a secure manner. So, we’re combining our experience and expertise in cloud computing, data, and healthcare to help organizations solve some of the toughest problems in healthcare while prioritizing data privacy. Every day, we embrace the prospect of healthcare data revealing life-changing information, and we share the exhilaration of our customers when disparate data sources come together to deliver answers that save lives.
In this spirit, we’ve invited Fernando Vieira, CEO and chief scientific officer of the ALS Therapy Development Institute (ALS TDI), to share his organization’s story. ALS TDI is a unique nonprofit research organization dedicated to discovering and developing effective treatments for amyotrophic lateral sclerosis (ALS). Their goal is to significantly accelerate the understanding of this terribly complex, invariably fatal disease and to discover and invent ALS treatments that commercial partners can advance through clinical trials. ALS TDI has already invented one drug, tegoprubart, which has completed a phase 2a trial in ALS, and they plan to advance many more.—Shweta Maniar, director of life science industry solutions, Google Cloud
Amyotrophic lateral sclerosis (ALS), also known as Motor Neuron Disease (MND), Lou Gehrig's Disease, and Charcot's Disease, robs people of their ability to move.
The effects of ALS are common to most patients: The upper motor neurons in your brain and the lower motor neurons in your spinal cord degenerate and die, so your muscles no longer know how to move, and you gradually become paralyzed. The progression of the disease, however, can vary wildly. For example, I lost my best friend to the disease nine months post-diagnosis, whereas the chairman of ALS TDI’s board of directors has been living with ALS for more than 17 years.
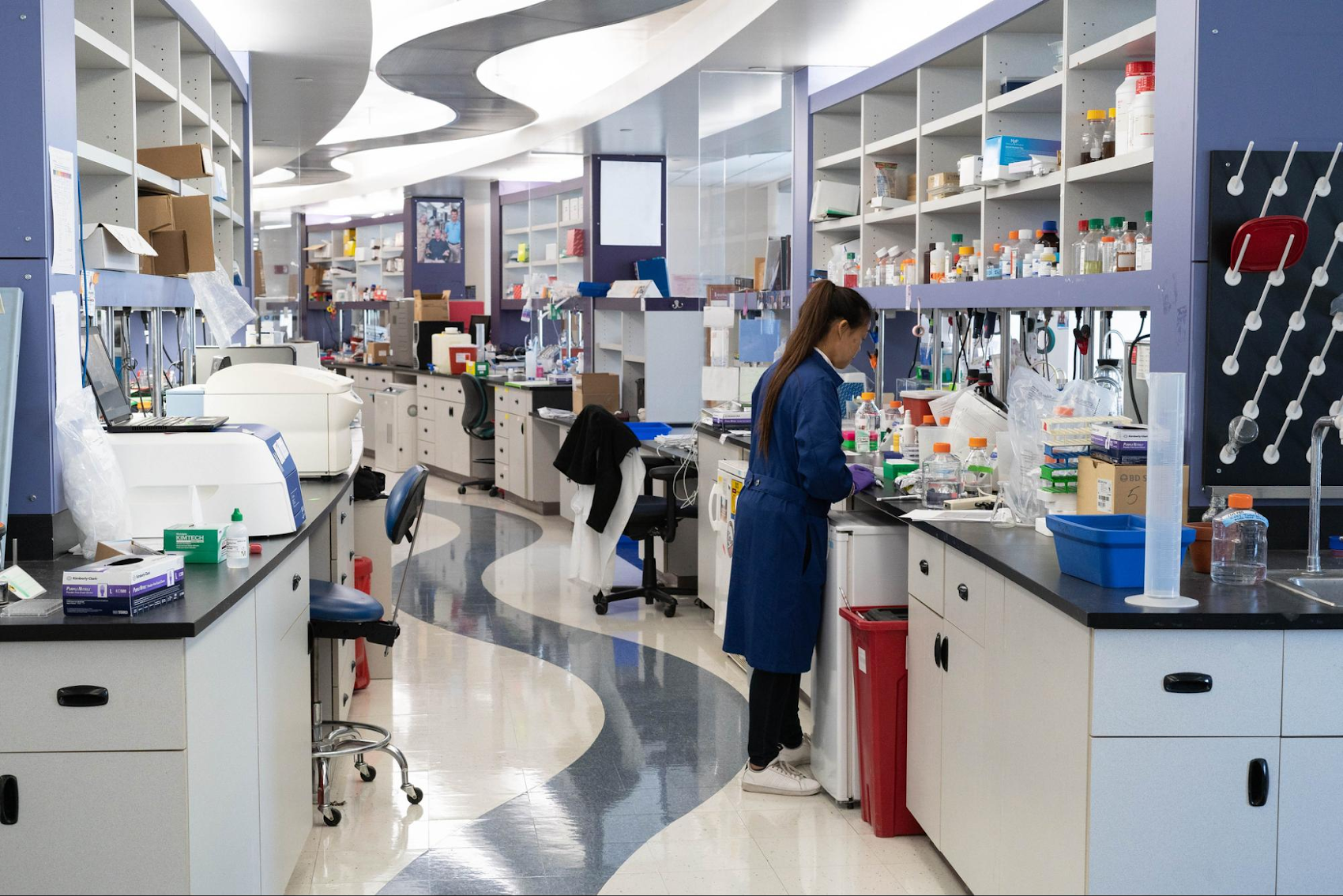
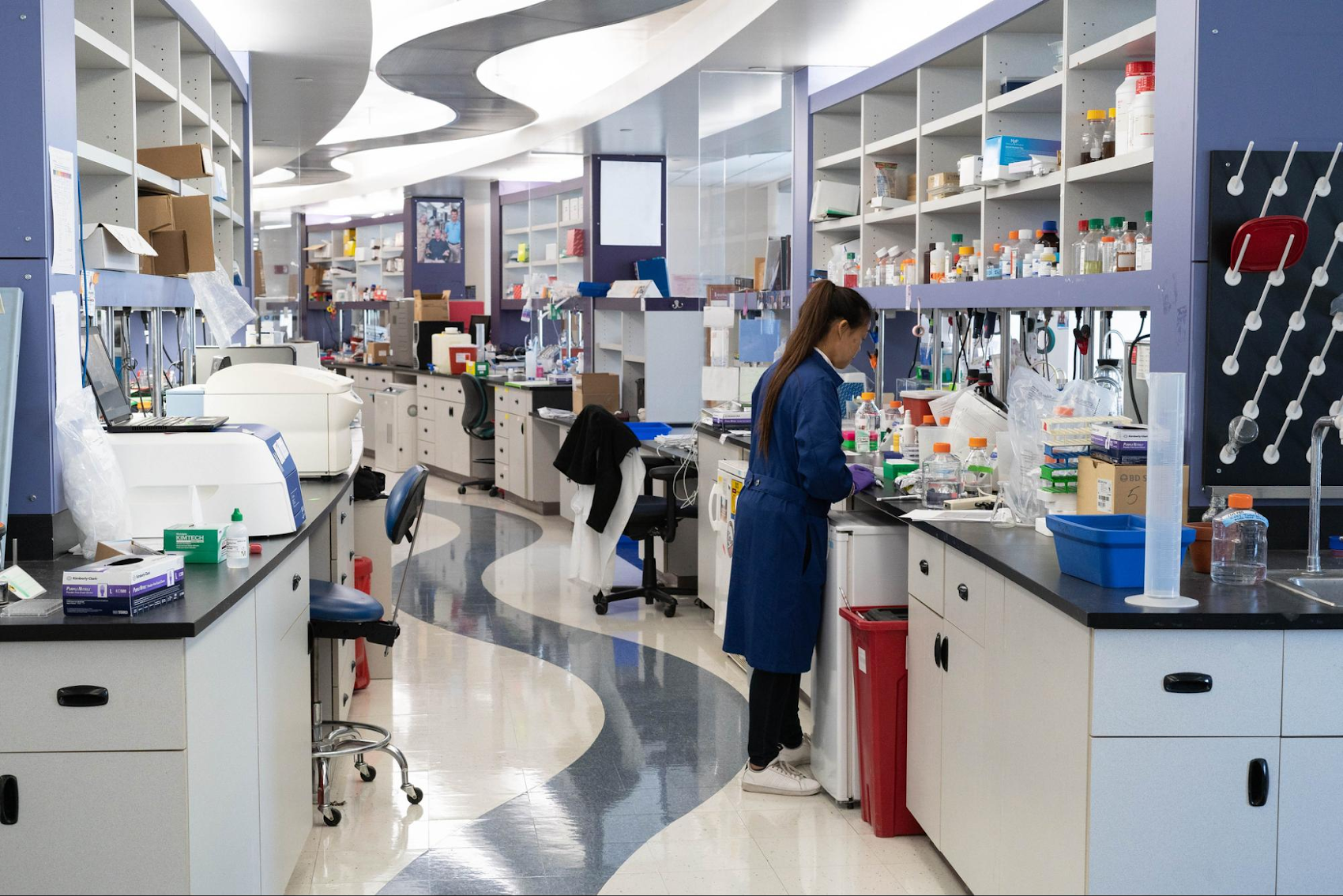
Because ALS is incredibly heterogeneous in underlying biology and presentation, it’s hard to understand what causes it and to measure, at a population level, how interventions affect disease progression. While the ALS field has identified a number of genes relevant to the 10% of cases that are inherited, what those genes do differs greatly, which tells us there are many paths to motor neuron degeneration.
In fact, ALS probably isn't a single disease, but a collection of related diseases. Thus, it’s likely that no single therapeutic measure will address everybody's needs completely — some may help everybody a bit and others may help a small subset a lot. For this reason, it’s extremely important not only to find effective treatments but also to identify the patient subsets that can benefit most from them.
Learning about ALS from people with ALS
To make progress against such a complex disease, the ALS field, including ALS TDI, realized that we had to learn about the disease by collecting as much data as we possibly could from people who have ALS.
Many programs were doing whole genome sequencing, but they weren't collecting information about ALS progression. Cell biology studies (using induced pluripotent stem cells collected from people with ALS) weren't relating findings back to whole genome sequencing or to clinical progression. Other studies were asking about risk factors—where you’ve lived, your occupation, how you eat and exercise—but weren't connecting those factors to the type of ALS that patients ultimately developed.
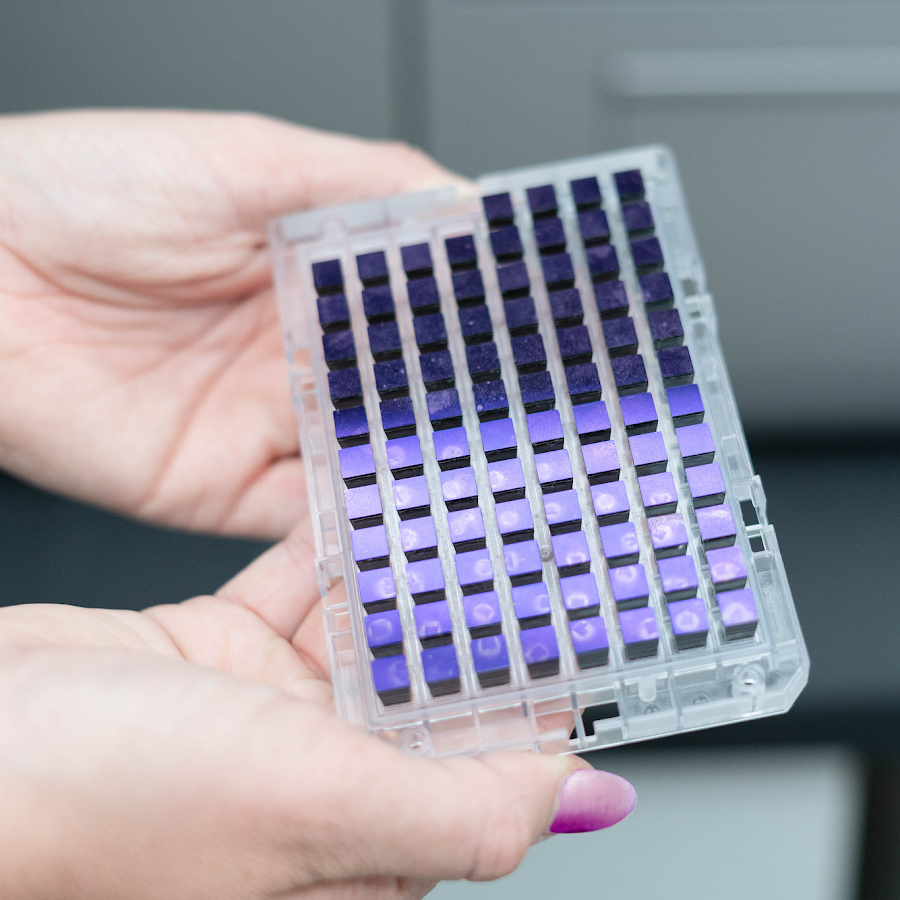
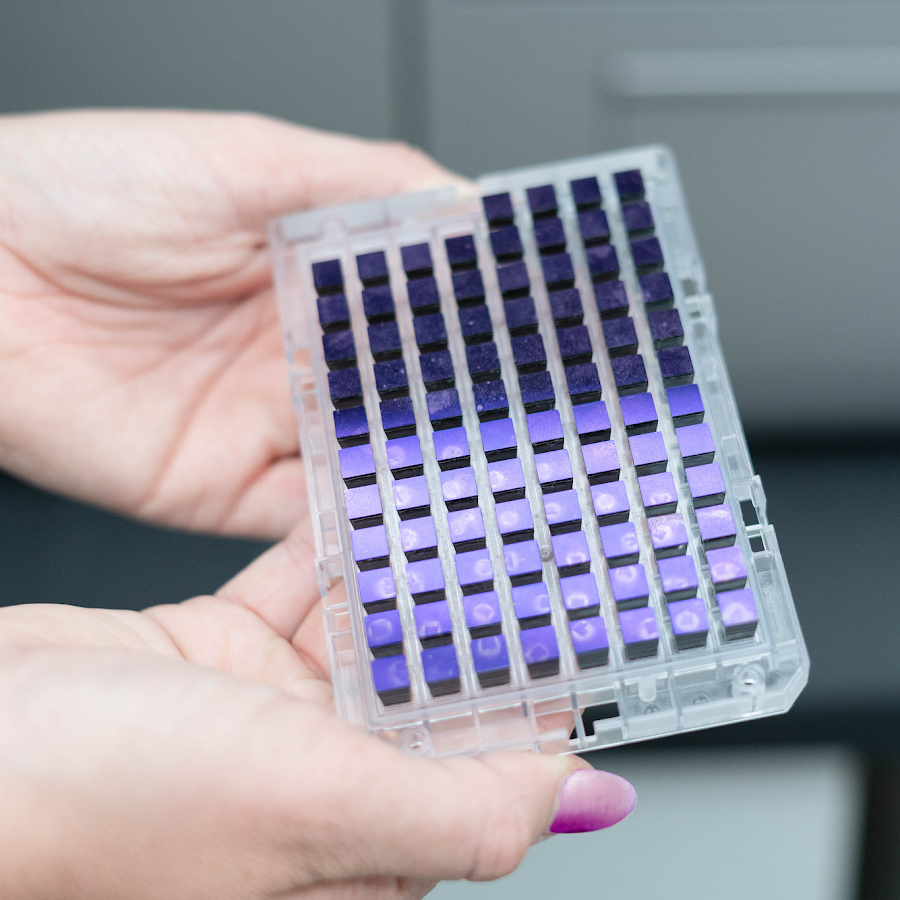
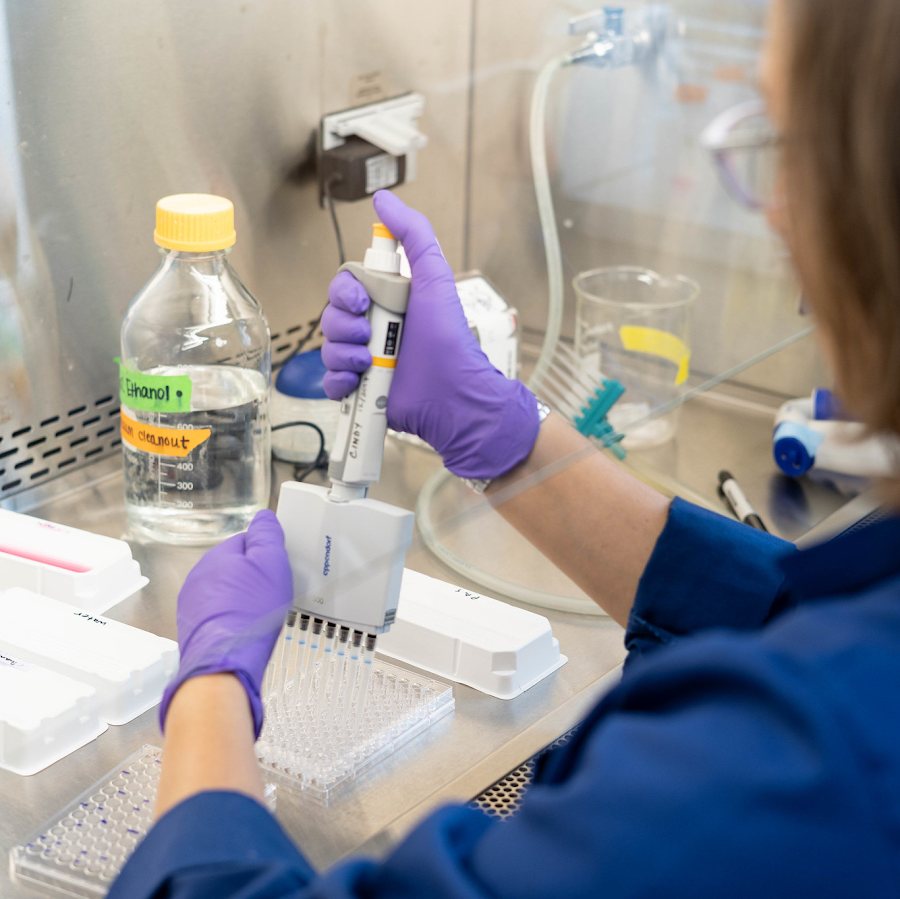
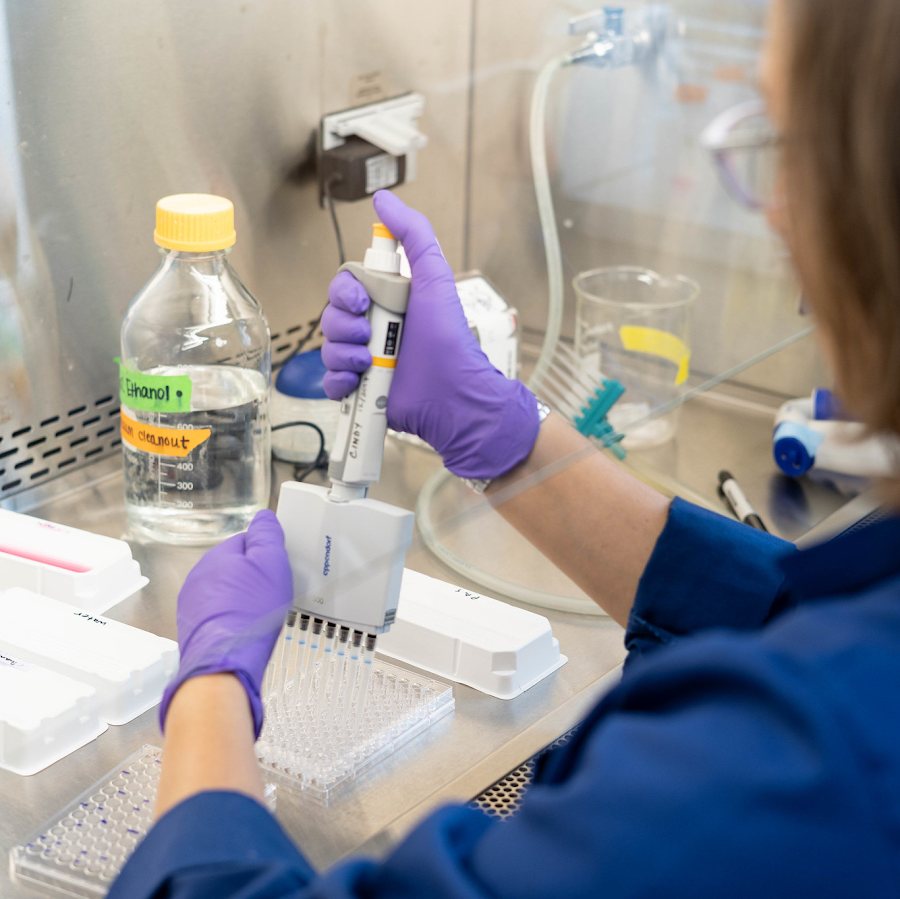
In 2014, with the goal of breaking down these data silos, ALS TDI initiated a “direct-to-patient” program called the Precision Medicine Program (PMP) that implemented innovative ways to capture data directly from people with ALS, such as accelerometer measurements and voice recordings.
Our program is now the longest running and most comprehensive of its kind. In the past eight years, we’ve collected more than 30 terabytes of carefully curated data from almost 850 people with ALS.
The challenge of analyzing big data
Since our participants’ time and motivation to contribute is so precious, the most important goal of our initial solution was to get the data in any way we could. We’d worry about how to analyze it later.
Once we had amassed a large pool of data, we wanted to see what questions it could help us answer.
Our original system was good, but not great. Whenever I had a question about our data, I'd have to describe it to our web development or IT teams, who would then build queries for me. Unfortunately, because I don't speak tech and they don't speak biology, I’d get answers that didn’t quite address my question. Overall, the process was slow and cumbersome.
Moreover, we couldn’t ask all the questions that we wanted. For example, ALS researchers consult PubMed almost every day to find new papers (published at a rate of five to seven per day) implicating a new protein, gene, or biomarker or uncovering correlations between certain blood characteristics and ALS progression.
Untapped potential was sitting in our on-site servers, and we couldn't quite get to it. We needed to make it easy to ask questions of the dataset, not only for our internal scientists but also for the larger research community.
At ALS TDI, we wanted to ask whether our dataset supported the conclusions of such papers. And if it did, we wanted to ask follow-on questions to determine what next step might identify a new therapeutic target or intervention point that could lead to a new drug for a specific subset of people. For example, we wanted to ask, “Is the protein this paper identified altered in any of our Precision Medicine Program (PMP) participants, and if so, what is their disease like?”
That was the dream, but it wasn't possible with the system we had.
Untapped potential was sitting in our on-site servers, and we couldn't quite get to it. We needed to make it easy to ask questions of the dataset, not only for our internal scientists but also for the larger research community, because we know that we don't have a monopoly on good ideas or brainpower at ALS TDI.
Using cloud computing to unlock data
We determined that cloud computing provided the best way to secure the data, scale our system, and make it easily accessible—nationwide and worldwide. And so, it made sense to connect with the Google Cloud team. Instead of selling us the technology and leaving us to do the rest, they said, “Here are the tools, we'll help you figure it out, and we'll connect you with other helpful people we trust.”
Our first step was to create a data lake, a central place to store all the data. Next, we created what I call “bronze” and “silver” layers to format the data so we could easily connect them to visualization tools. The ability to create interactive dashboards was the final selling point for us.
We built a presentation layer using Looker, which made it possible for researchers to ask questions of the data without relying on a developer to build queries for them. We could initiate queries on our own and view the results graphically in moments instead of waiting hours or days.
Our developers at ALS TDI worked with Google Cloud and their partner Quantiphi to sketch an initial framework of a data analytics platform and teach our own developers and scientists how to build out the rest of the tools ourselves. We’re now excited to share with the public our new platform, which we’re calling the ALS Research Collaborative Data Commons, or the ARC Data Commons.
Getting big results in record time
Although today we’re only in beta testing, the solution already works for our internal scientists exactly the way we dreamed. They’re simply amazed at what can now be done so quickly.
Potential partners in academic, nonprofit, and for-profit spaces marvel that so many people with ALS have contributed and how a biologist can query this massive database without writing code in Python or JSON. They can quickly test their hypotheses against the real-life data and gain enough confidence from the results to design their next study.
For example, a potential pharmaceutical partner recently reached out and said they had a drug that can target a gene implicated in ALS that is so rare, nobody had really thought about it yet. Within five minutes of getting the email, I opened up the ARC Data Commons genomic dashboard and found the gene, along with all program participants who harbor polymorphisms or mutations in that gene.
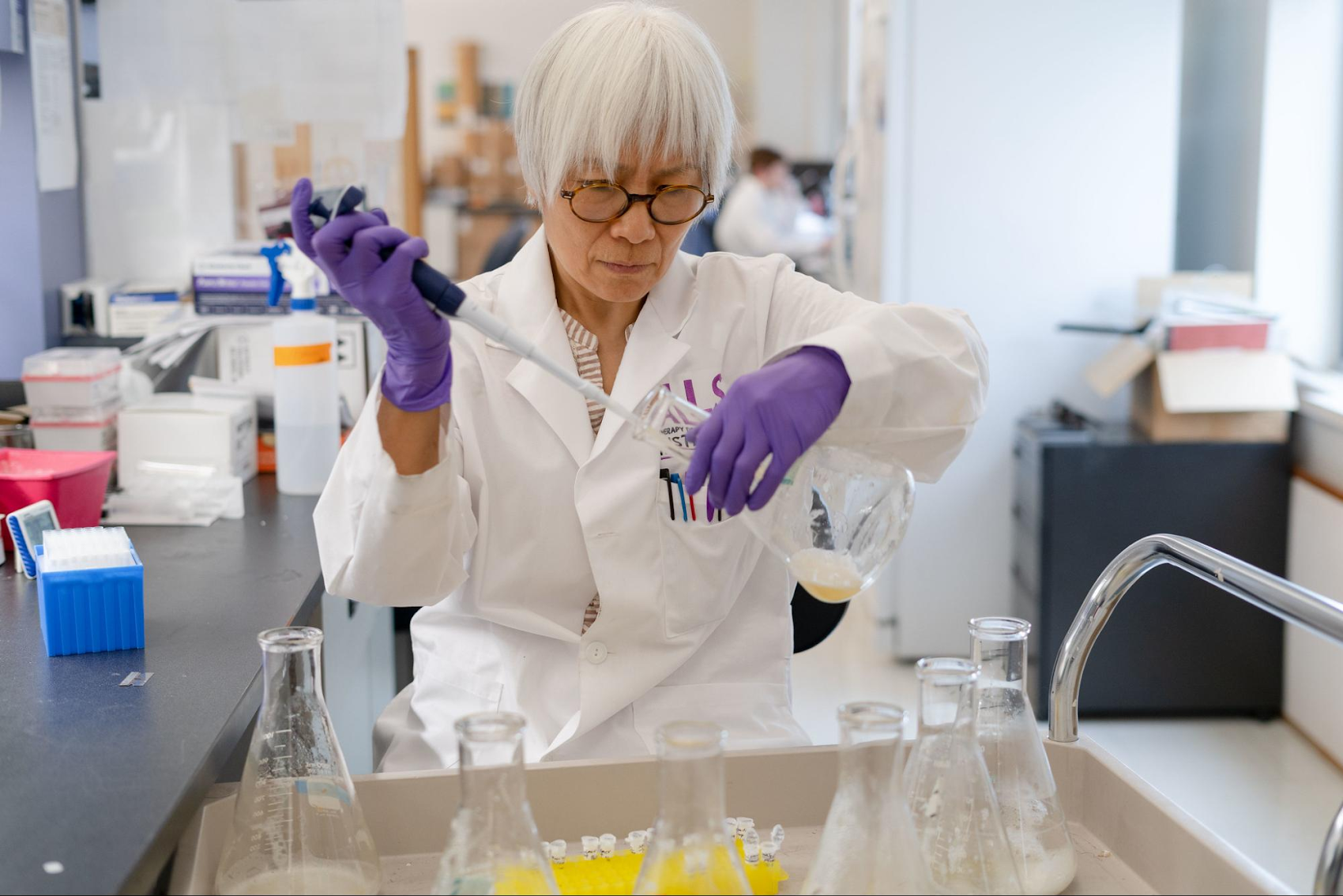
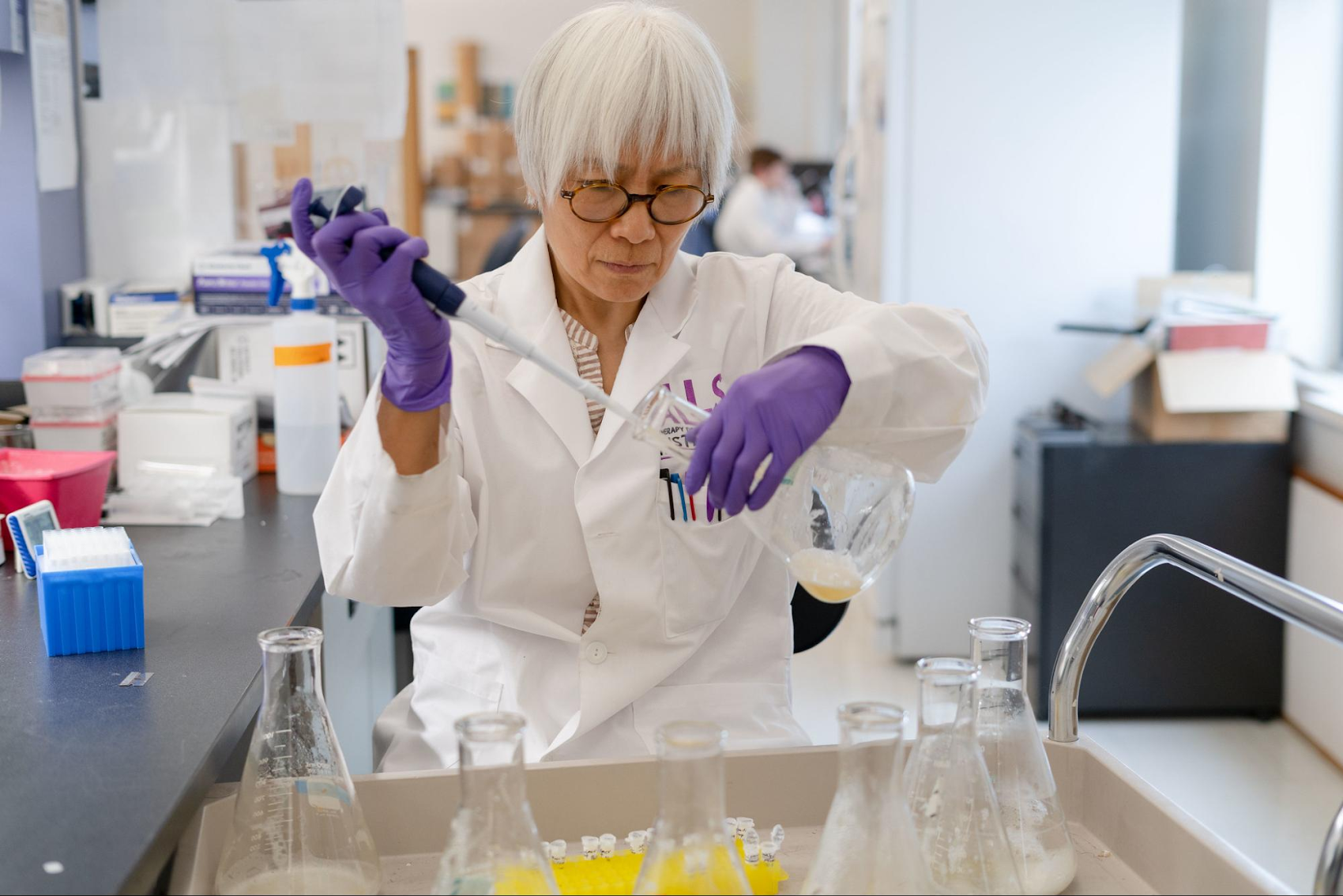
Using the platform, I was able to plot their progression types and then ask the database, "From whom do we have cells?" When I learned we had fibroblasts or induced pluripotent stem cells from two participants for use in lab experiments, I wrote back saying, "Here's how we can help." This whole process took 15 minutes. A year and a half ago, I wouldn't even have tried.
A key hallmark of our research program is treating participants as partners. People with ALS may also have their own gene of interest, whether they carry a mutation or heard about it in the news. In time, we hope to make these data available to PMP participants as well, but I’ve had the ability to demo it with a few while it’s in beta. It’s been really satisfying — for me and for them — to show how quickly we can answer their questions about a patient subpopulation that harbors a specific gene mutation.
A groundbreaking tool to advance ALS research
At the time of writing, the ARC Data Commons is still in the iteration stage. Whenever I uncover a new use case, I send it to ALS TDI’s web dev team, who uses the time they used to spend pulling data for me to instead build a new button or filter I can use forever.
Our new tool also helps our clinical operations team save time. For example, it simplifies the logistics of sending phlebotomists to people's homes so they can contribute samples. And because the solution scales quickly and cost-effectively, we plan to integrate new data types from those samples — such as measurements of 7,000 different proteins from large proteomics studies and 30,000 RNA molecules from large transcriptomic studies — then connect them to patient metadata and our knowledge of biological pathways.
This whole process took 15 minutes. A year and a half ago, I wouldn't even have tried.
Our goal for 2023 is to start getting the ARC Data Commons out there by inviting researchers, academics, and industry partners to access the data. The next step, with Google Cloud's help, is to generate new insights by applying artificial intelligence and machine learning models to sift through the data in ways that the human mind and eye can't.
Scaling up: more data will give us more insights
Cross-analyzing our data with additional datasets could yield even greater insights. ALS is so heterogeneous that it bleeds into other neurodegenerative conditions. In fact, the Venn diagram of neurodegenerative conditions contains a lot of overlap. For example, in our study of familial cases of ALS, we’ve seen that the same genes with the same mutations in the same family sometimes manifest as frontotemporal degeneration, damaged neurons in the front of the brain that can behave a lot like Alzheimer's disease.
In an ideal world, we’d connect numerous data lakes because it’s highly likely that there’s as much, if not more, to learn from comparing ALS to diseases such as frontotemporal dementia, Alzheimer's, Parkinson's, and Huntington's. We may find therapeutics that work across diseases or across subsets of people with those conditions.
The idea of our Precision Medicine Program was "if you build it, they will come." That is, if we build this solution, people will come and share data. Now that we're able to share data, we hope to create a virtuous cycle of more people coming to share more data with us so that we can share more data with more researchers, leading to more patients sharing, and so on.
We’ve already seen how quickly we can find answers to questions we could never ask before — and we’re just getting started. We’re determined to keep going until all people with ALS can receive effective treatments.