Squawk bots: Can generative AI lead us to understanding animals?
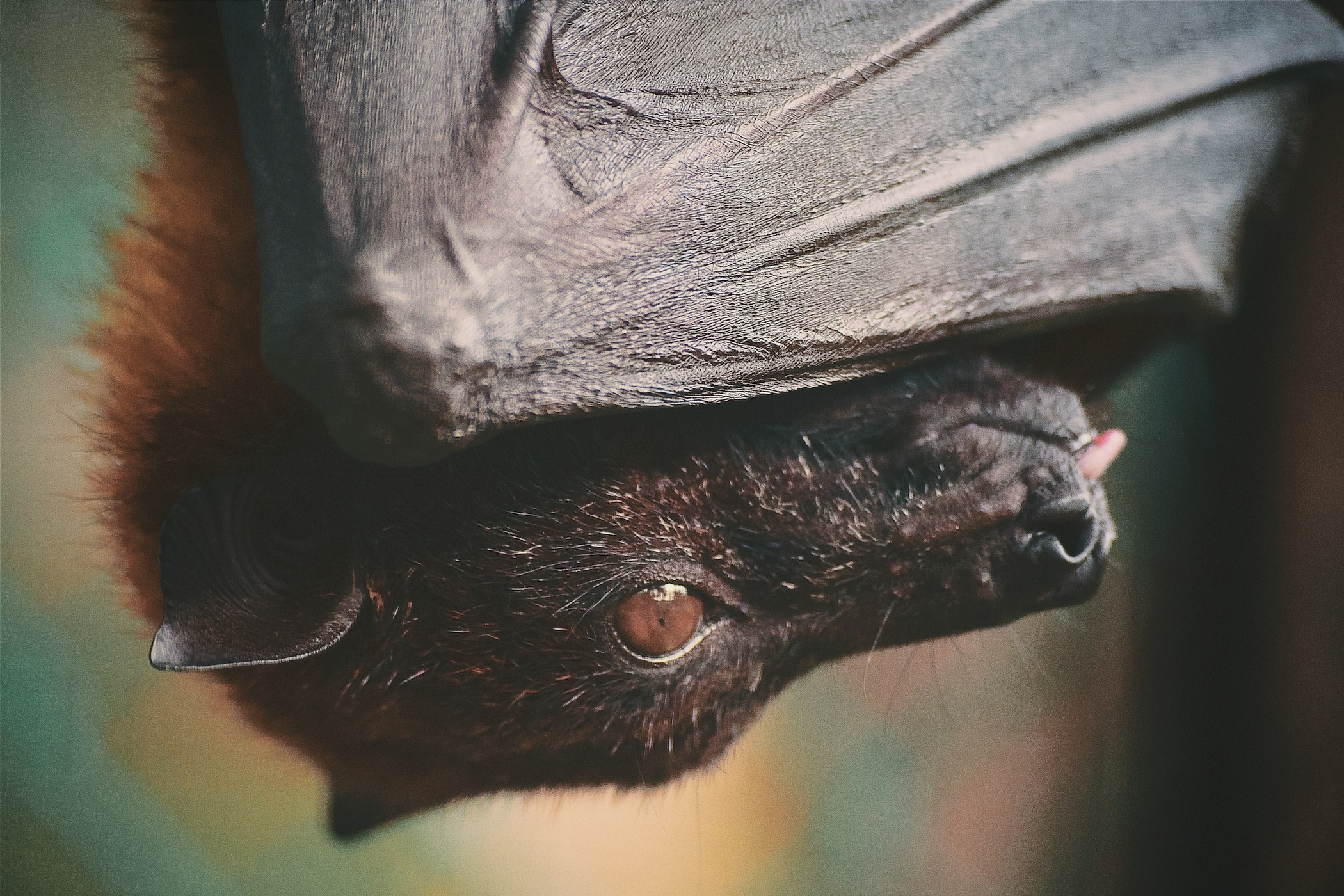
Michael Endler
AI Editor, Google Cloud
The Earth Species Project is using large language models just like Bard to develop tools to decipher animal communication. We might even squawk back.
The era of artificial intelligence is giving us a new species of advanced discovery tools. Earth’s complex systems have been full of mysteries to researchers and scientists for thousands of years. Now, with the ability to create and aggregate data on a massive scale and analyze it with AI and machine learning, new classes of mysteries can be unlocked.
One area we have long marveled at and been mystified by is the animal kingdom. What if using our increasingly human-like AI, we could increase our understanding of these animals.
The Earth Species Project was founded six years ago, with just such a goal in mind. It aims to use AI to better understand and communicate with the many species and cultures with which we share the planet. Along the way, the organization is building tools that will advance the work of animal behavior researchers and deliver better conservation outcomes.
“As human beings, our ability to understand is limited by our ability to perceive,” Aza Raskin, one of the cofounders of the Earth Species Project, said during a recent interview. “What AI does is that it throws open the aperture of what we can perceive.”
Just as the discovery by Western society of whale song in the 1970s led to a moratorium on deep-sea whaling and contributed to the birth of the Environmental Protection Agency, the team at the Earth Species Project hopes that the more we truly know about the creatures around us, the more we will know about ourselves — and the more we can do to protect the planet we share.
“I think about how humans have been speaking and communicating vocally, passing down culture, for 100,000 to 300,000 years,” Raskin said. “Whales and dolphins have communicated vocally, passing down culture and songs, for 34 million years. That which is oldest correlates with that which is wisest, so just imagine what we can learn by listening.”
To achieve this, Raskin and the team at Earth Species Project are including some of the most popular and consequential innovations of the moment as part of a suite of techniques to realize their project: generative AI and large language models. They believe the insights behind models, such as Google’s LaMDA and GPT-3, can be foundational in understanding of non-human communication.
Broadly speaking, the Earth Species Project is trying to solve an unstructured data problem through the use of machine learning and artificial intelligence. It’s just that, unlike most other problems solved with AI, understanding animal communication has no basic ground truth, no foundation from which to start.
The project is currently in the process of working with scientists and researchers across the world to create benchmarks and foundation models which include data from a range of species, including whales, seals, dolphins, crows, and many other kinds of birds; as well as working with partner animal labs on early communication experiments.
Imagine a LaMDA or Bard, but for animal communication. Just like you can build a text chatbot in a human language you don’t understand, ESP believes they can build an audio chatbot for animal communication that nobody yet understands.
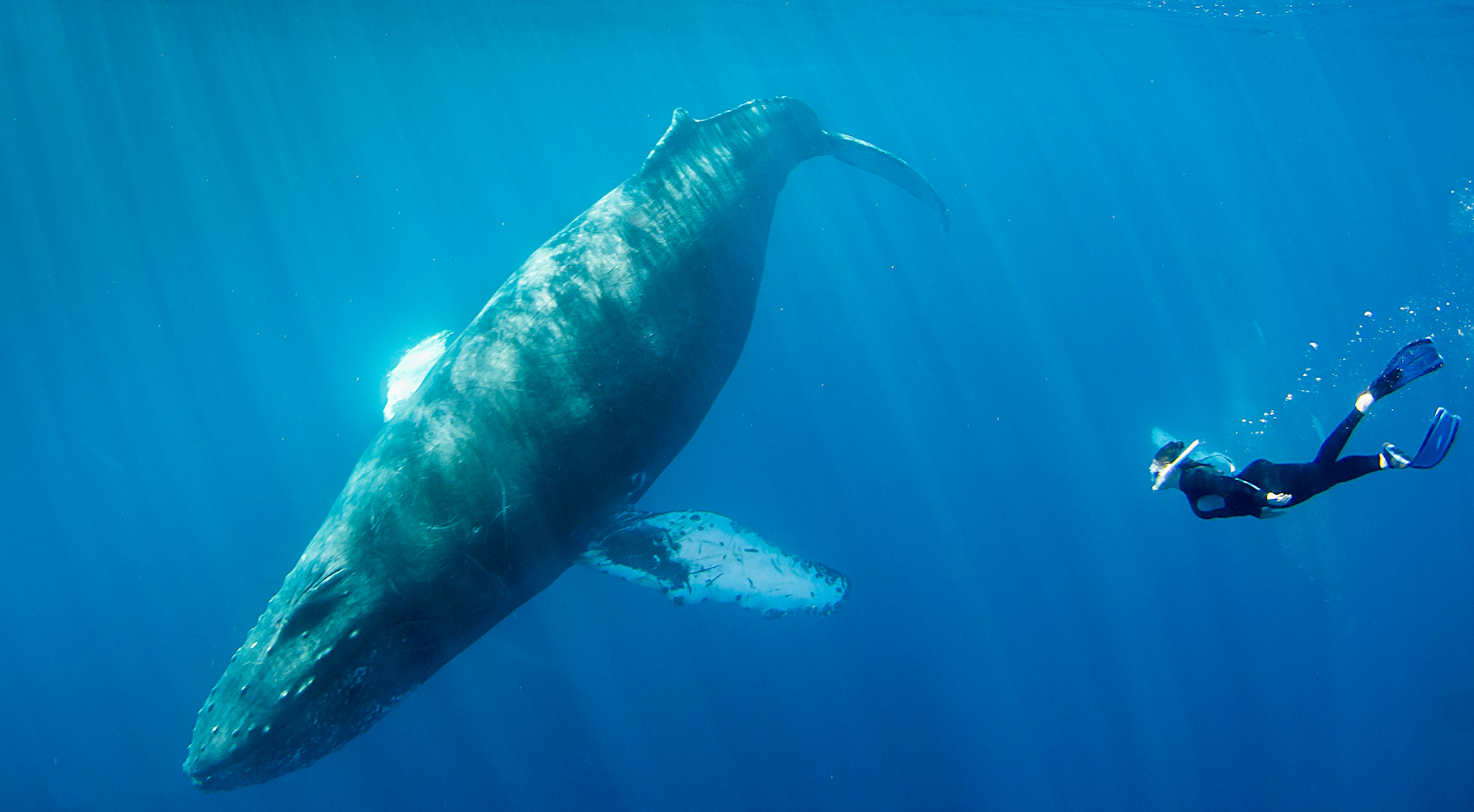
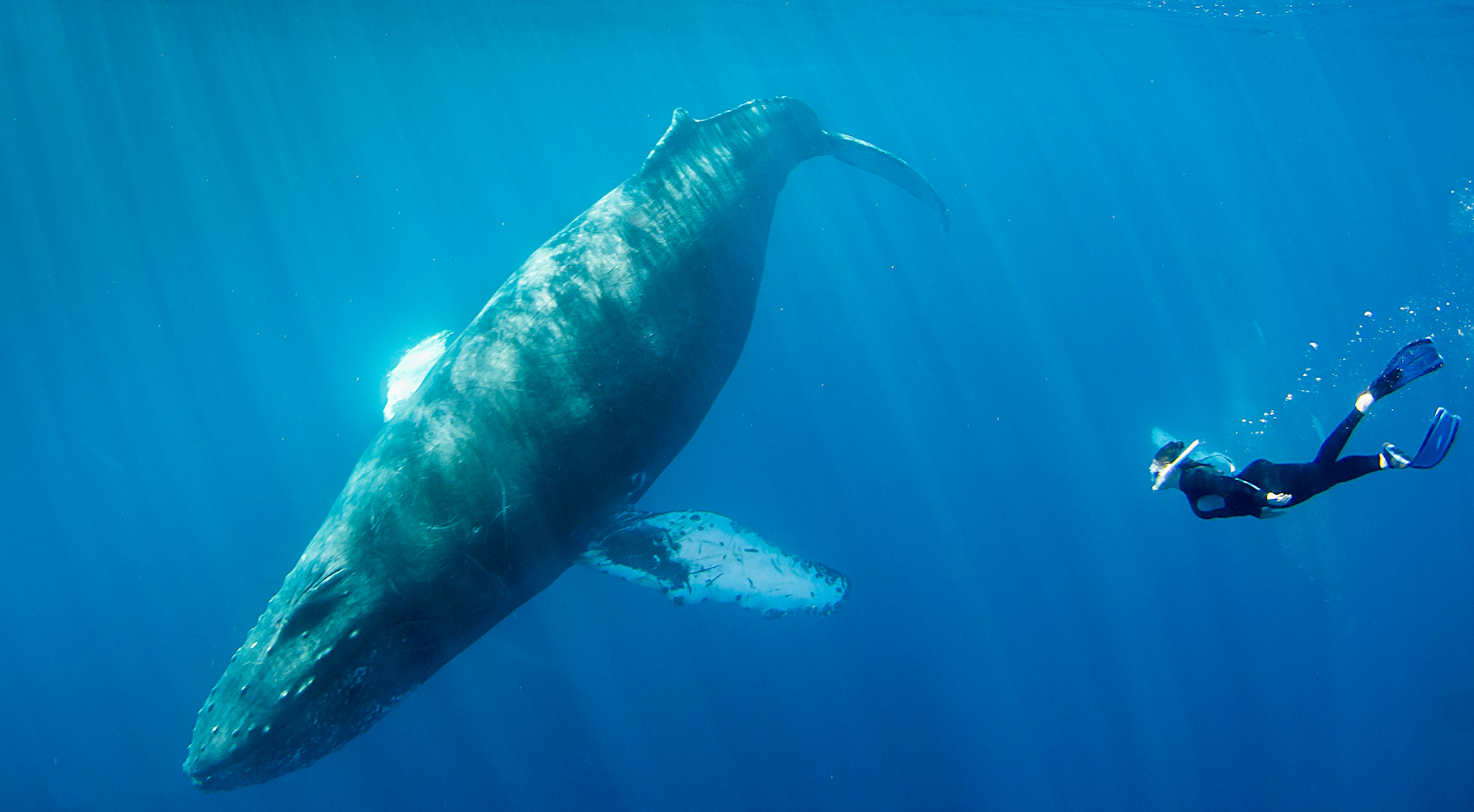
ESP’s work happens to sit at the confluence of two major movements — environmentalism and technology — that have evolved to take a stronger hand in shaping society in recent decades. As humanity has struggled to reign in carbon emissions and climate change in recent years, there is growing hope that technology might be able to pull us back from the precipice of climate catastrophe.
Language models and uncanny shapes
Our drive to communicate with each other across multiple and often complex languages means that we’ve already done a lot of the work to model and translate human languages.
When it comes to the communication of animals, we have none of this. No full Rosetta Stone exists for decoding what animals are talking about, although decades of knowledge built by animal researchers have already decoded more than many think.
This intersection of intrinsic knowledge, linguistic analysis, and gaps in understanding make it an excellent job for artificial intelligence.
AI is good at recognition tasks and finding patterns in data. Unlike a human trying to make sense of a sprawling dataset, AI doesn’t much care what the data is or what it might mean. Machine learning crunches through the data, building structure from the internal relationships of whatever it is fed.
At the Earth Species Project, that data comes in the form of sounds, motion, and video recorded in either the wild or captivity, sometimes with accompanying annotations from biologists on what the animal was doing at the time and in what context.
That dataset is growing. With the maturation of the Internet of Things, the ability to put cheap and reliable recording devices such as microphones or biologgers onto animals in the wild is easier than ever. This bioacoustic data can then be organized and analyzed with AI tools in ways that may help to uncover meaning, which can then be tested using generative approaches, where humans recreate the sounds of the animals to enable two-way communication.
In this task, researchers have taken a page or two from the human language playbook.
Raskin said that the Earth Species Project drew inspiration from the early research on natural language processing models, some of which has become the basis for generative AI. The core insight was that machine learning can turn semantic relationships into geometric relationships, and that a language can be represented as a shape defined by the relationship between its concepts.
For instance, the relationship of the word “dog” is related in language samples to words like “friend” and “fur” and “howl.” This map can be represented visually to give us a shape for entire languages.
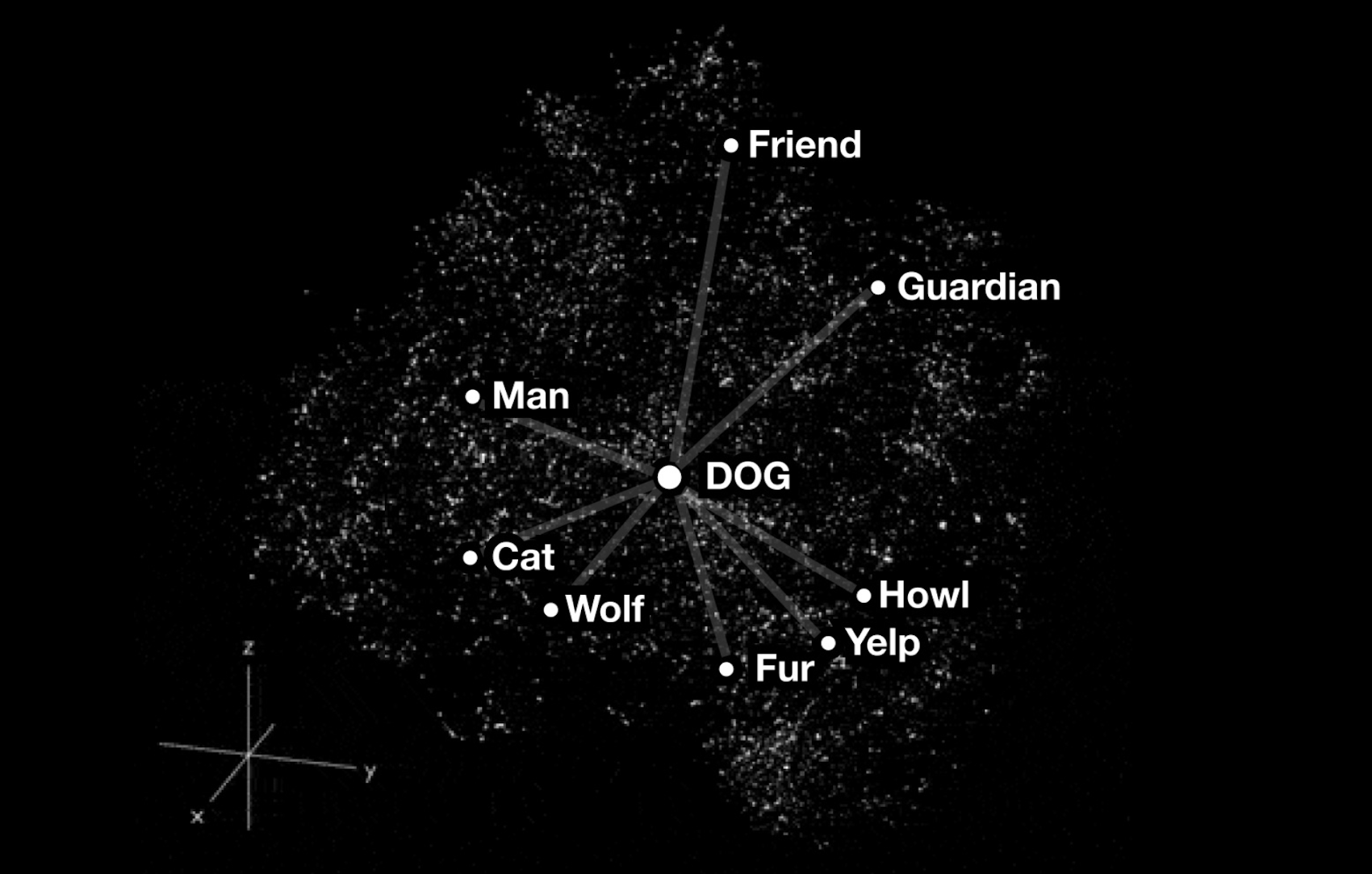
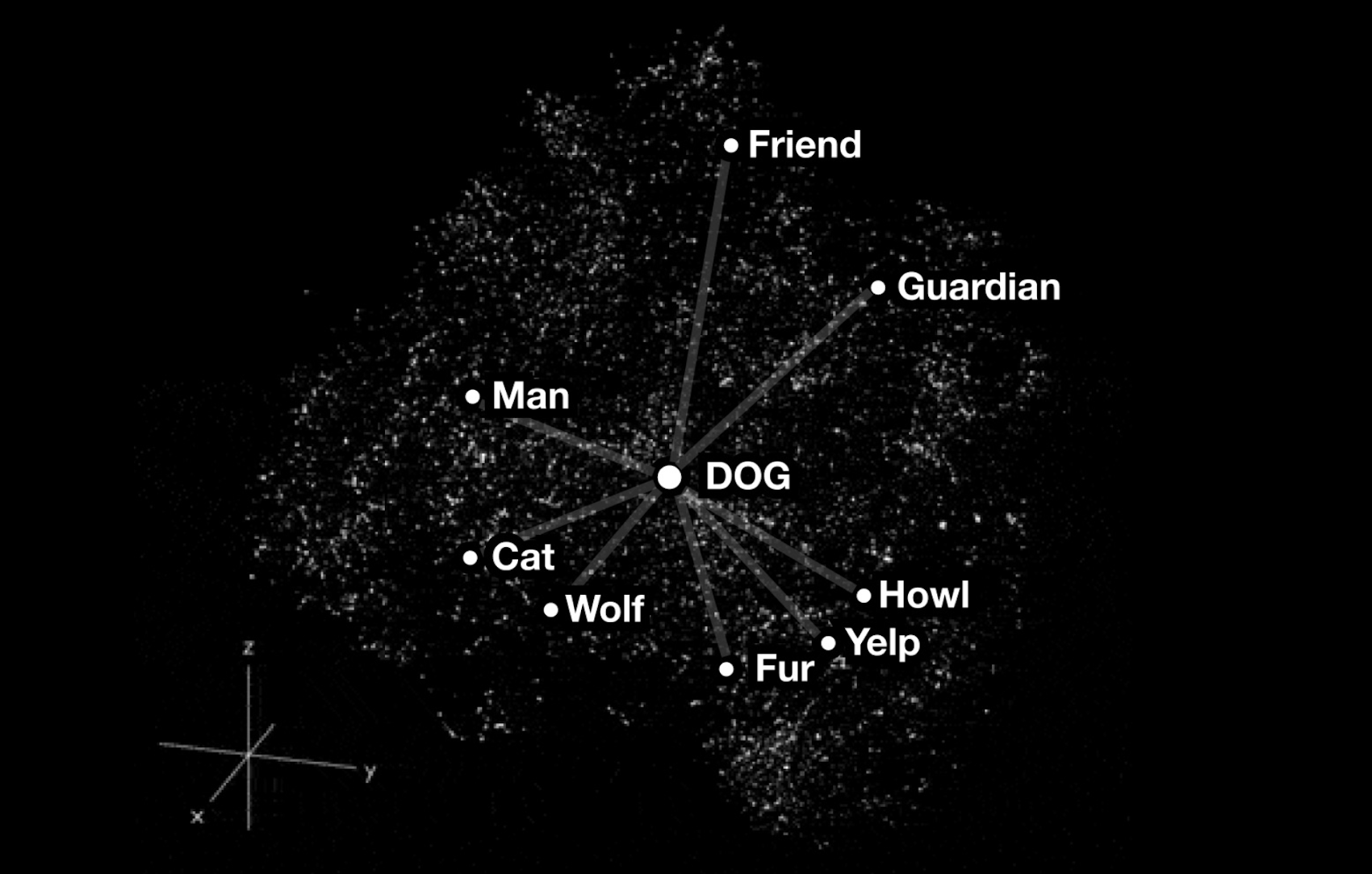
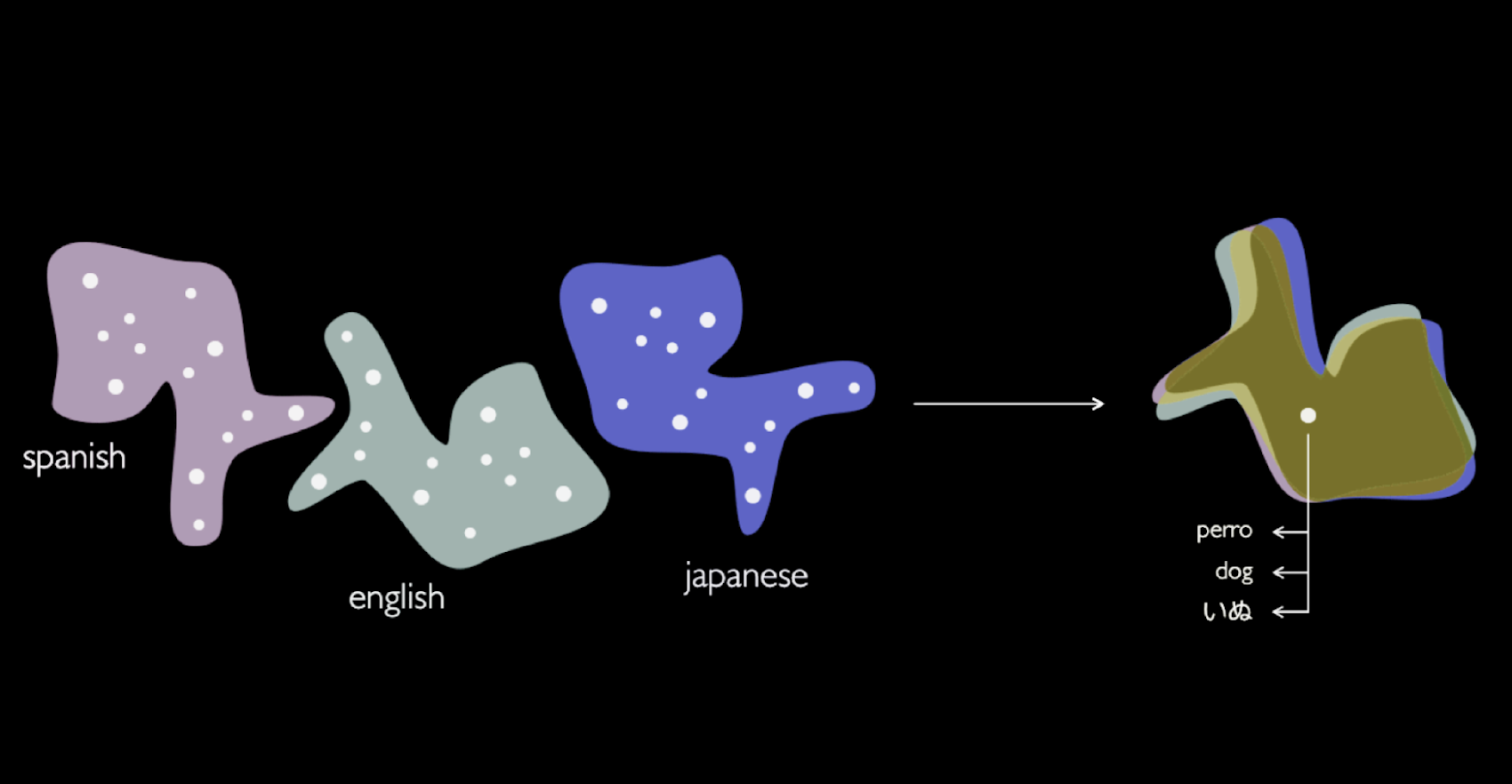
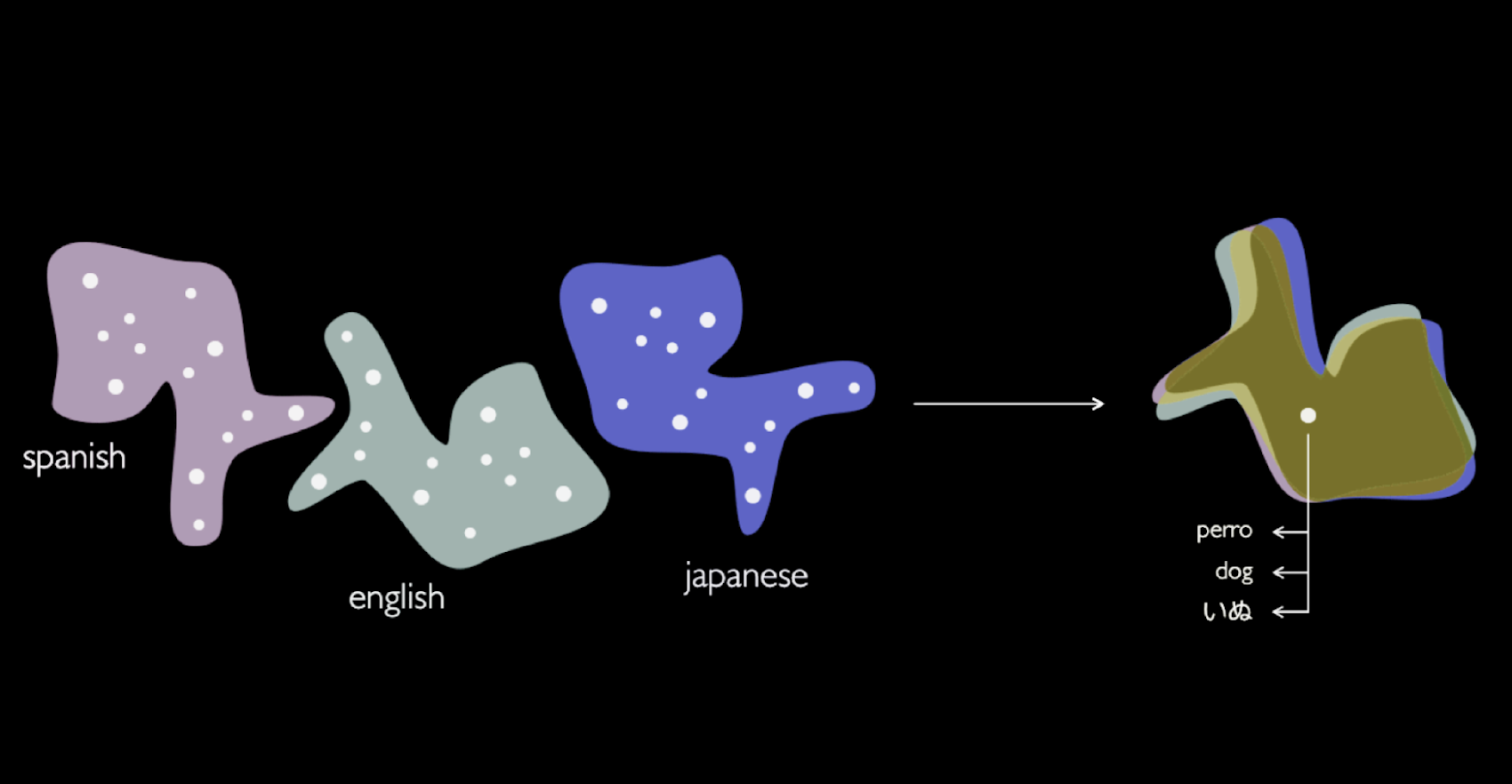
The interesting thing about the shape of these geometric language models is that, if you look at the shapes of different languages, patterns emerge between seemingly unrelated maps. For instance, the word “dog” appears in the approximate same place, no matter if the language map is for English, Spanish, or Japanese.
“If you want to hold just one thing in your mind about how AI works, it’s that it turns semantic relationships into geometric relationships. This is a core concept,” Raskin said. “The cool thing about these shapes — the technical term is embedding space or latent space — is that it encodes the semantic relationships of a language. While early translation of languages without Rosetta stones was accomplished by matching the shapes of languages to each other, the field has moved on to even more advanced techniques — and it is those more advanced techniques which ESP uses.”
Semantic relationships are the associations that exist between the meanings of words or phrases. Synonyms, antonyms, and homonyms are examples of semantic relationships in linguistics, and help us understand the differences or similarities between words. It is very unlikely that similar patterns exist for all forms of communication, regardless of species. But there may be some similarities. And that’s one of the things the Earth Species Project is trying to figure out.
“We're building on top of the research in the human domain,” Raskin said. “We're building models that work with bats, as well as with whales. With humans you almost always know you can ground truth at some point, you can always check. That's not the case with animals. So that also introduces new sets of scientific problems. Science always proceeds comparatively. And so that's what these tools do, you compare A to B.”
Building the fundamental AI tools to understand animal communication
Artificial intelligence is, fundamentally, a data-driven tool. Before the Earth Species Project can start translating what whales or crows are saying, it first needs to make sense of all its data. This starts with setting benchmarks, akin to a set of tests, that lay out the goals and parameters the AI must achieve to be successful. By setting the parameters, the AI will keep going until it achieves its goal, in a sense learning or figuring out the problem that has been set out for it over time.
“We've seen in the human domain that to really galvanize a field, you need a set of benchmarks across a wide variety of species and tasks,” Raskin said. “With the right benchmarks, the hope is to galvanize our understanding of animals, as well.”
ESP has recently developed BEANS, the Benchmark of Animal Sounds, which is the first-ever benchmark for animal vocalizations. It establishes a standard against which to measure the performance of ML algorithms on bioacoustics data.
ESP has also developed AVES, the Animal Vocalization Encoder based on self-supervision. AVES is the first-ever foundation model for animal vocalizations, useful for a wide variety of tasks such as detection and classification of signals.
“Partnerships are critical for advancing this field. We work with 40-plus biologists and institutions to gather the data,” ESP CEO Katie Zacarian said. “Then we turn it into something useful for them and us, adding it to the benchmark, so we know over time whether ours, and other people's algorithms, are getting better. With some partners, we work on helping them design new experiments or new data collection so that we can get into a feedback cycle with them. Their contributions, often built on many decades of research, are pivotal.”
We're building models that work with bats, as well as with whales. With humans you almost always know you can ground truth at some point, you can always check. That's not the case with animals.
Most AI needs to be trained on an existing dataset: for example, this is the sound of thousands of known birds, now here’s a bunch of recordings, identify the birds for me. Self-supervised learning works from little or no familiar data, and instead uses patterns from similar datasets, like the aforementioned semantic maps, to make reasonable inferences from new datasets. So using the same bird data to identify different kinds of bats, judging by distinctions in vocalization.
Google Cloud has been supporting the Earth Species Project on initiatives such as these by donating computing resources to power the project’s machine learning algorithms. The nonprofit has also been in conversation with Google’s audio language modeling team and the Office of the CTO for guidance and expertise.
“We've seen in the human domain that to really galvanize a field, you need a set of benchmarks across a wide variety of species and tasks,” Raskin said. “With the right benchmarks, the hope is to galvanize our understanding of animals, as well.”
Ethical translation and communication with animals
As the Earth Species Project’s technical roadmap advances, undertaking additional data collection and developing new benchmarks and foundation models, leading the way towards human-animal communication.
Raskin predicts this could be feasible within a few years.
“Can we do generative, novel animal vocalizations?” Raskin said. “We think that, in the next 12 to 36 months, we will likely be able to do this for animal communication. You could imagine if we could build a synthetic whale or crow that speaks whale or crow in a way that they can't tell that they are not speaking to one of their own. The plot twist is that we may be able to engage in conversation before we understand what we are saying.”
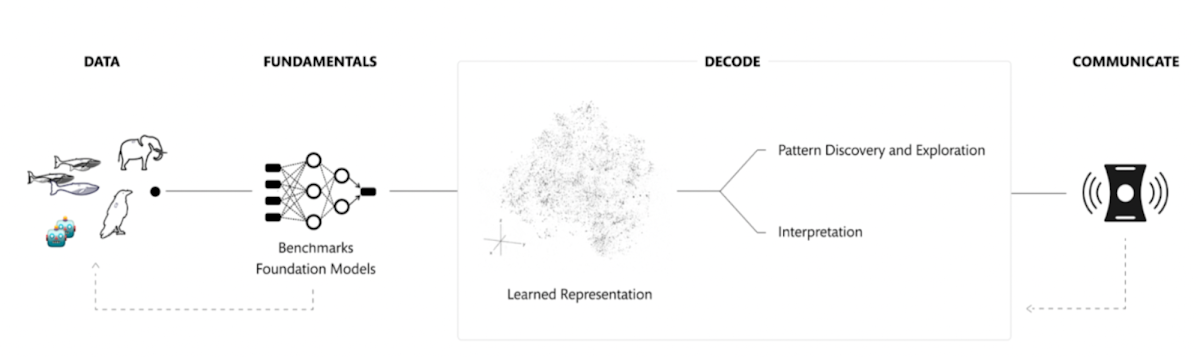
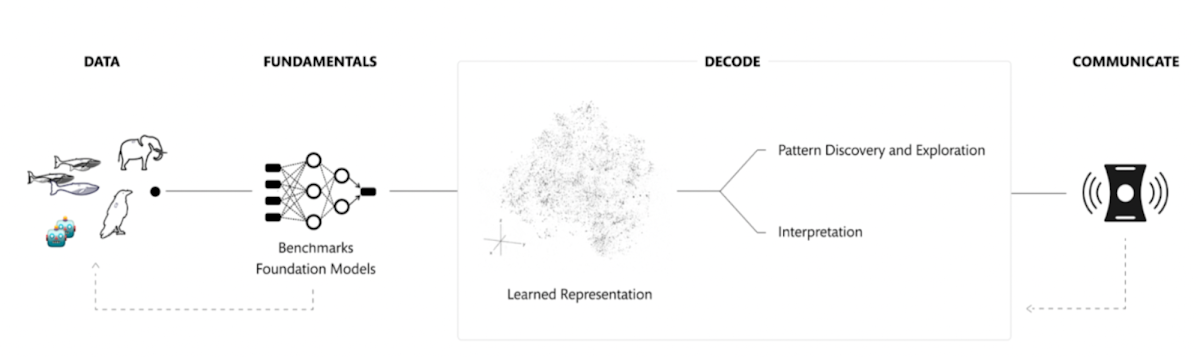
One notable area of concern is ethical issues, which the nonprofit takes very seriously. Raskin and his colleagues are already in conversation with biologists and other scientists and researchers about the responsible uses of these artificial intelligence methods, and they have prescribed having them on hand for any tests. The roadmap notes potential risks such as interfering with hunting and foraging or mating, should the wrong messages be sent to the animals.
An instructive example has been the way whale songs are known to go viral among the population in the ocean. Humanity could cause more harm to the animal kingdom than just giving a group of whales an ear worm.
“If we're not careful, you could disrupt a 34-million-year old culture,” Raskin said. “Which would be a monumental tragedy.”
Imagine a LaMDA or Bard, but for animal communication. Just like you can build a text chatbot in a human language you don’t understand, ESP believes they can build an audio chatbot for animal communication that nobody yet understands.
That’s why much of the nonprofit’s work has so far been in data collection and in creating the fundamentals — the benchmarks and foundation models that will drive future progress forward. The approach is no different than what companies and organizations around the world do with artificial intelligence and machine learning every day, just on a more ambitious and grandiose scale. Many of those companies are at the stage of figuring out what to do with all that collected data.
If AI can help us understand what animals are saying, what are the actual limits to our capabilities with AI?
And, if AI can help us understand animals, what will it teach us about ourselves? Raskin and Zacarian hope that the eventual translation of animal languages becomes one of those turning points in world history, like when whale songs were first discovered or the photo of The Blue Dot in 1990. These were moments that altered how the collective thinks and understands our world, and helped spur efforts to protect it.
“There are these moments in time, I think, when we get a shift in perspective and we see ourselves in a new way and that changes everything, transforms our relationship with ourselves, each other, the world around us — everything,” Raskin said. “Moments can become movements.”