Putting the AI in QA: How cloud helps manufacturers with quality inspection
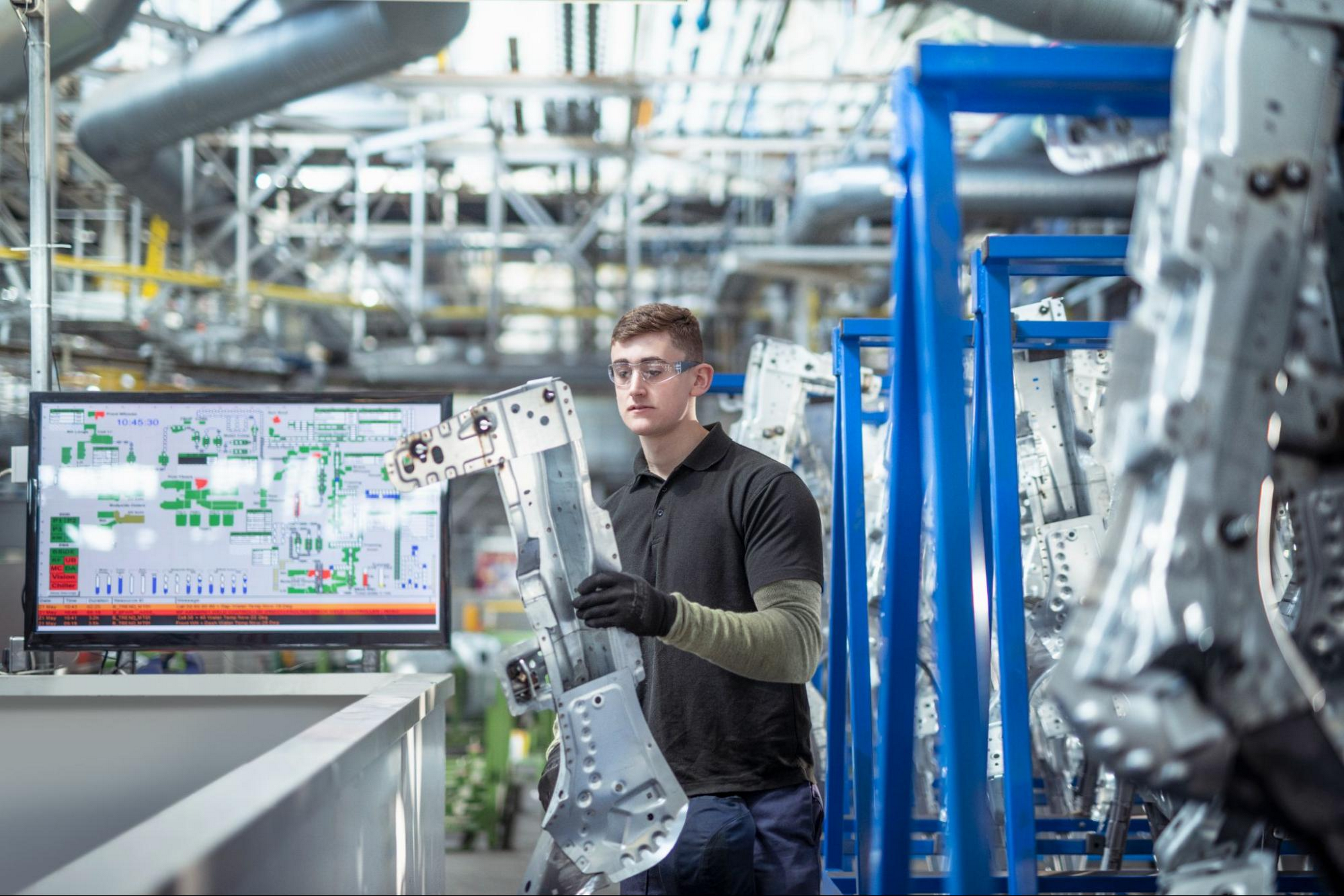
Hans Thalbauer
Managing Director, Supply Chain, Logistics & Manufacturing, Google Cloud
When factory spending on quality-related issues reaches 40%, AI can save both money and time.
Building a product right the first time, every time, is the goal of every manufacturer. Of course, the realities on the manufacturing floor make that kind of 100% efficiency nearly impossible. As such, manufacturers invest heavily in tools, processes, and people to ensure quality, because the cost of poor quality finished products can often be too much to overcome.
Add to that the persistence of supply chain shocks and continuing economic uncertainty, and manufacturers margin for error has rarely been thinner. Fortunately new cloud technologies are arriving just in time to help close the gap on quality, driving both efficiency and savings.
Even in the best of times, the American Society of Quality estimates that most manufacturers spend the equivalent of 15% to 20% of revenues on “true quality-related costs.” Some organizations go as high as 40% cost-of-quality in their total operations. Cost centers that affect quality in manufacturing come in three different areas:
Appraisal costs: Including verification of material and processes, quality audits of the entire system, supplier ratings.
Internal failure costs: Waste of resources or errors from poor planning or organization, scrap material that cannot be used, correction of errors on finished products, and failure of analysis regarding internal procedures.
External failure costs: Repairs and servicing of delivered products, warranty claims, complaints, returns.
Artificial intelligence is helping manufacturers improve in all these areas, which is why leading enterprises have been embracing it.
According to a 2021 survey of more than 1,000 manufacturing executives across seven countries interviewed by Google Cloud, 39% of manufacturers are using AI for quality inspection, while 35% are using it for quality checks on the production line itself. Computer vision—the ability for a computer to observe and identify objects or characteristics—has become a common tool for manufacturers to automate the quality assurance process, easing the burden of repetitive inspection tasks from workers on the factory floor and helping to speed up overall operations.


“AI-based visual inspection can bring a high level of robustness and accuracy never achieved with rule-based systems,” said Bruno Ménard, director of software at Teledyne DALSA, which makes imaging components and semiconductors. “When the parts being inspected have a high variation in shape and texture and are in a significantly changing environment, a well-trained AI-based system can resolve those variations with a great level of reliability. As a benefit of this improved accuracy, manufacturers can expect reduced production costs as a result.”
Manufacturers are under mounting pressure to save time and money wherever possible. One of the surest ways is to increase quality and efficiency by getting production right the first time. AI is helping to catch flaws faster, so fewer happen. It can also inform the entire operation to cut down on future mistakes.
Here’s a look at some of the most promising areas for AI improvements in manufacturing quality assurance.
How AI works for visual inspection
One of the most common uses of machine learning and computer vision is to spot irregularities. For instance, a machine learning algorithm can be trained to recognize a quality seam weld of two different pieces of metal. If the welding machine splashes or misses its target, the machine-learning algorithm can spot that irregularity and flag it, along with all the metadata about when it happened, what machine made the error, and the diagnostic status of the machine at the time.
Machine learning can also spot defects introduced within the production process. Instead of looking for irregularities, the algorithm can be trained to specifically look for defects. For instance, if a plant is printing circuit boards, the algorithm can be trained to spot scratches or dents or misalignments of the components.
Training such models can be time consuming, however, which is why the development of specialized models like those deployed in Google Cloud’s Visual Inspection AI, are so powerful. Traditionally, manufacturing AI depended on simple anomaly detection, which relied on vast libraries of images for the algorithm to compare against. The newer specialized models use an approach called active learning to get more quickly up to speed with a smaller set of images. This allows for not only faster deployment but also better iteration and accuracy over time.
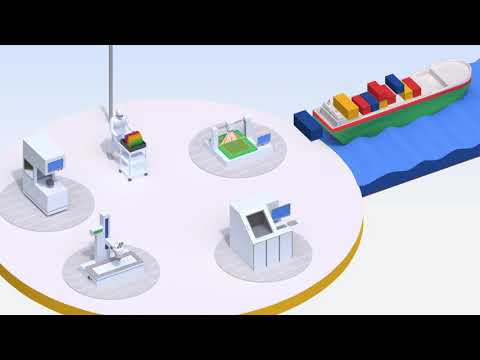
Computer vision for quality assurance
Computer vision for quality assurance can be found most often in automotive manufacturing (engine blocks, paint finish, door sheets), computer chips and wafers, batteries, solar panels, and more. Visual inspection is typically performed in-situ as the manufacturing process is being performed, utilizing sensors like high-speed cameras or spectrometers that, when combined with the machine learning algorithms, can detect errors and defects with high degrees of accuracy.
“AI is typically excellent for quantitative tasks, such as the ones traditionally performed by humans—locating and identifying defects such as surface scratches, missing or damaged PCB components, dust particles on an image sensor,” Ménard said.
Here are some of the most prominent examples of companies using computer vision and artificial intelligence for quality assurance:
Welding seams: To inspect welds, the artificial intelligence model is trained on what kinds of irregularities the algorithm should identify as errors, such as splatter or if the seam has collapsed during welding. Automotive manufacturers use visual inspection to inspect robot-welded seams for anomalies at critical structural joints on the chassis.
Automobile parts: Visual inspection is used in a variety of cases in quality assurance in automobile manufacturing. For instance, Renault uses Google Cloud’s Visual Inspection AI to implement more accurate quality controls with a significantly reduced time to market at a lower cost. And Ford is using the same tool to look for defects at the stamping station for metal sheets in its cars.
Lithium-ion battery sheets: Lithium batteries of all sizes are made of positively charged sheets (cathodes), negatively charged sheets (anodes) and a separator sheet placed between them with a liquid or solid electrolyte layer to facilitate connections between the sheet. While the concept of lithium-ion batteries is simple, the manufacture can be sensitive. Line scan cameras are mounted in the production facility to inspect the sheets as they move quickly by, looking for surface defects like scratches, dents or bent edges.
AI-based visual inspection can bring a high level of robustness and accuracy never achieved with rule-based systems
Printed circuit boards: Visual inspection can inspect dozens of objects on printed circuit boards (PCBs) to detect misplaced, damaged, or missing screws, springs, components and soldering issues. FIH Mobile, a subsidiary of powerhouse electronics manufacturer Foxconn, uses Google Cloud’s Visual Inspection to ensure the quality of its PCBs.
Silicon wafers: Computer chip manufacturers use computer vision to find wafer or chip defects or cracks in the die. Kyocera Communications Systems has been able to, “create highly accurate models with as few as 10 to 20 defective images with Visual Inspection AI.”
Diamonds: The image that comes to people’s minds when they think of inspecting diamonds is squinting through a loupe to determine if a diamond is real. But imaging hardware and AI can help sort through large batches of diamonds (like the tiny diamonds used in encrusted bands or equipment), looking for the tell-tale signs of fake diamonds.
Solar panels: AI can be used to inspect solar panels either during the production, or to check for damage while deployed in the field. In the manufacturing process, line scan cameras can check to make sure the panels are not dented or scratched during production. In the field, drones equipped with infrared cameras can hover over a solar array and check for hot spots on the panels, indicating that they are either broken or not working at optimal efficiency.
New solutions to challenging problems
Implementing AI on the manufacturing floor has long been seen as a significant engineering challenge. Old machines are not always easy to retrofit with new sensors like high-speed line cameras or infrared cameras, spectrometers, or even barcode readers. In addition, expertise in manufacturing hardware or imaging technology does not mean that a company also has the knowledge to implement robust data analytics and artificial intelligence solutions.
“Data and expertise” are, according to Ménard, the primary challenges of instituting AI for visual inspection.
“Until recently,” he continued, “the amount of data available has a direct implication on the quality of the results and the speed of learning. Also, the sheer scope of optimization and the rapid pace of new research and tools can be overwhelming, even to seasoned practitioners. Fortunately, the latest advances in AI research and technology have signaled the introduction of new tools that are easier to use; require less time and expertise, in both AI and programming; and most importantly, require less data to achieve good deployment accuracy.”


Solutions are emerging, such as Google Cloud’s Visual Inspection AI, that aim to solve many of the problems that manufacturers find when trying to develop AI for quality assurance. Google’s offerings require no special expertise in AI or computer vision from manufacturing engineers and can be implemented quickly to build accurate models with as few as 10 labeled images.
From this base, the service only grows more accurate over time as it’s exposed to more images. Visual Inspection AI can locate and identify the specific defect within each part, which reduces the time spent by engineers to diagnose problems, rework parts, and implement process improvements. With labor shortages and a generation of retirements, getting more eyes on the floor, even if they are programmed, can prove invaluable.