No comparison: How MoneySuperMarket is turning their data into competitive differentiators
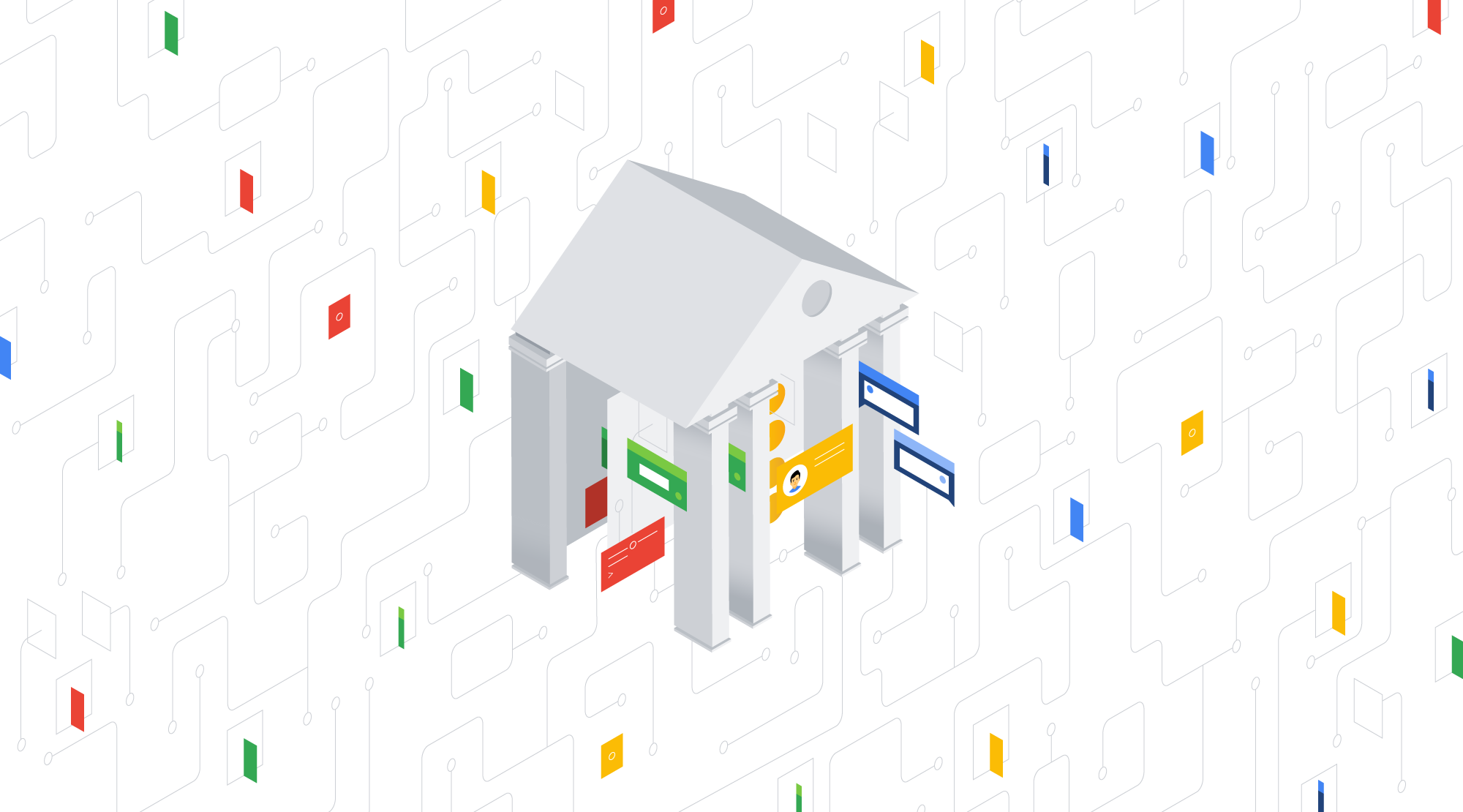
Aparna Sinha
Director of Product Management
Raffaello Martini
Customer Engineer
When customers in the UK want to compare prices for things like car insurance, home insurance, credit cards, and loans, they increasingly turn to MoneySuperMarket. With 13 million active users across the UK, MoneySuperMarket has become the country’s go-to price comparison website, saving its customers an estimated £2 billion a year. Its parent company, Moneysupermarket Group, comprises other popular brands like MoneySavingExpert, TravelSupermarket, and Decision Tech, and is an established member of the FTSE 250 index.
When customers use price comparison websites, they expect results that are fast and personalized to their unique needs. As a result, these price comparison sites can be complex to manage, with many moving parts that must work in unison. And with the breadth of MoneySuperMarket’s offerings, and the growing size of its business, it found that handling data became a bigger challenge.
In 2014, with growing traffic numbers and increasing popularity, Moneysupermarket Group found that its on-premises data warehouse was limiting its ability to scale quickly. As a result, it shifted to the cloud to mitigate the costs of storage, but still kept hold of its existing on-premises analytics solution. But as its data needs continued to grow, Moneysupermarket Group realized it needed to shift its analytics to the Google Cloud as well for the flexibility, scalability and ease of use it offers.
To make the change, Moneysupermarket Group used Cloud Data Transfer to extract its data to Cloud Storage buckets. From there, it used Google Kubernetes Engine (GKE) and Cloud Pub/Sub to orchestrate a process through containerized applications that cleans the data and loads it into BigQuery. Moneysupermarket Group also expanded its use of BigQuery as its cloud data warehouse, storing additional types of information such as events data from customer actions on touch points from different products and services,
The flexibility of GKE allows Moneysupermarket Group to use it for several other projects, including machine learning (ML) and web-facing APIs—using Python and mostly XGBoost as the ML classifier in the container application code. For example, it uses ML to serve its personalized customer recommendations, and GKE forms the backbone of the ML model training and inference pipelines. Each task in the model training pipeline—data extraction, feature engineering, model training, and model evaluation—runs as containerized applications in GKE and is orchestrated with Cloud Composer. The ML pipeline solution is automated, so it’s fast, requires little manual intervention, and easily scales to build multiple models for millions of customers. Using containerized applications for each pipeline task allows data scientists to make frequent incremental improvements through continuous integration and continuous deployment (CI/CD) practices with less fear than when they had the on-prem analytics solutions.
“With Google’s leadership in the Kubernetes ecosystem, Google Cloud delivers enterprise solutions, like Google Kubernetes Engine, based on their own learnings running services at scale,” says Harvinder Atwal, Head of Data Strategy and Advanced Analytics at Moneysupermarket Group. “This felt like the perfect fit for our data needs, as GKE features advanced networking, security, and operations support to reliably address our needs.”
With its new analytics platform, MoneySuperMarket has benefited most from the speed of development and running big tasks. In Harvinder’s own words, the most notable change has been the deployment time for its machine learning pipelines. "We went from eleven hours down to about five minutes," he says. That meant that the models could be updated every day instead of once a week, which, in turn, led to more relevant communications and offers, ultimately helping customers to save more money.
More than 80% of Google Cloud’s largest customers use GKE to run their workloads in production, and over 40% of GKE clusters are running stateful workloads. To learn more, visit cloud.google.com/kubernetes-engine.