Eating our own dogfood: Building an AI-driven business at Google Cloud Consulting
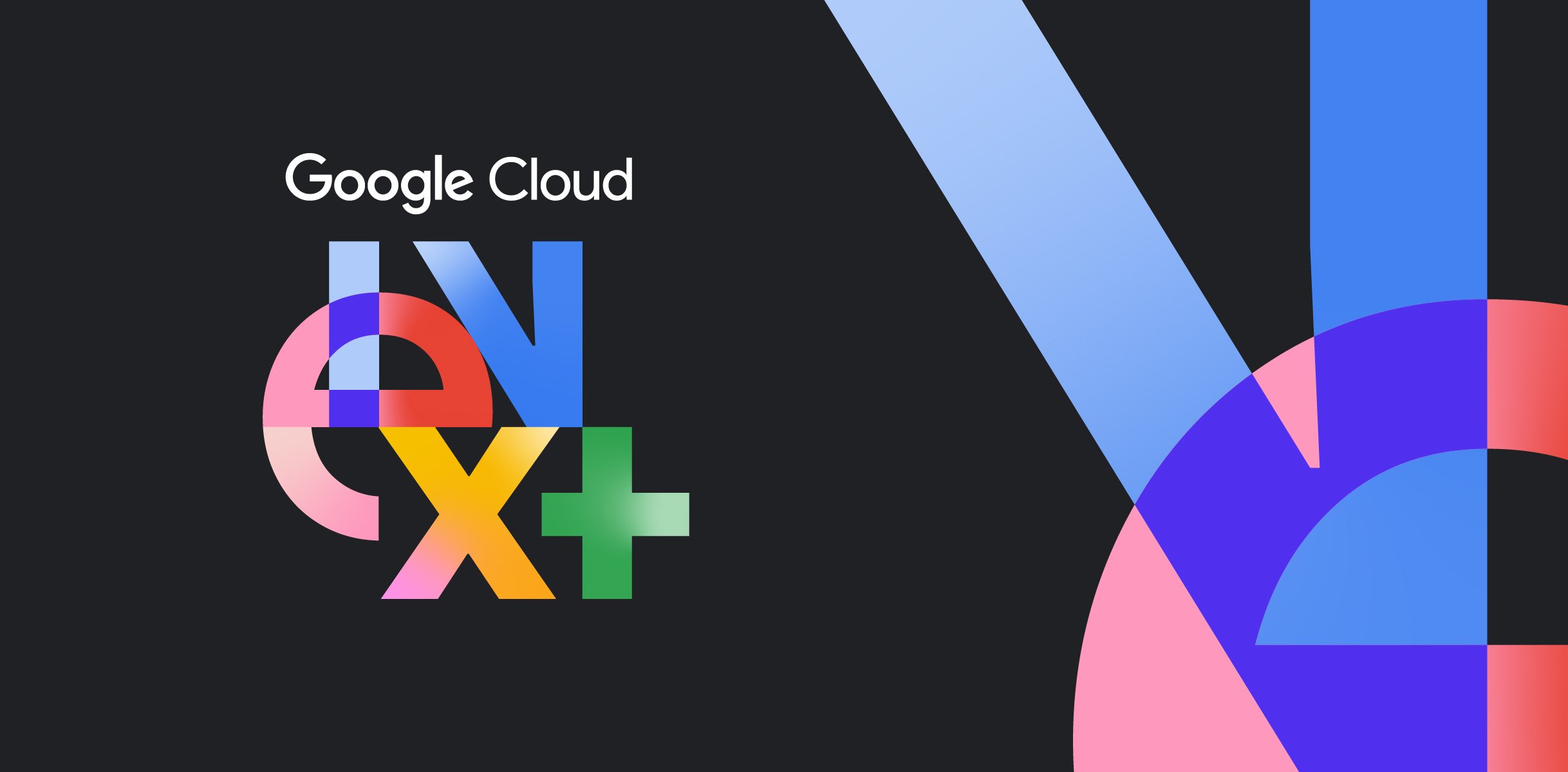
Bob Greenlees
AI Product Lead, GCC LEAP
Ashutosh Gupta
Director - GSD Core services, GCC Automation & Tools lead
At Google, we have a strong culture of dogfooding products — using and testing them internally to improve the experience for our own teams and customers. This practice is at the heart of Project LEAP, our internal effort within Google Cloud Consulting (GCC) to leverage Vertex AI in every part of our business.
This week at Next ‘24, we’re excited to share some of the ways we’re using Vertex AI products internally to create an AI-powered services business. Here are two of the exciting applications we’ve been developing on this journey.
1. GCC Assist: The AI-powered information hub
Google Cloud Consulting has a large corpus of rich data, including practical delivery experience, architecture models, best practice guidance, delivery guides, and templates. We have built an incredible repository of knowledge, but there was no simple way to quickly enable a user to tap into it and benefit.
Leveraging Vertex AI Vector Search and generative AI to summarize and synthesize information with simple natural language interactions was a huge opportunity to unlock the value of this data for everyone on our teams. The idea was simple: Create a centralized, AI-powered assistant that could intuitively understand and respond to natural language queries grounded in our data. With Vertex AI, GCC users now have a personal AI subject matter expert available on demand to help them find service delivery insights, best practice playbooks, service offerings based on customer use cases, and other key operational resources.
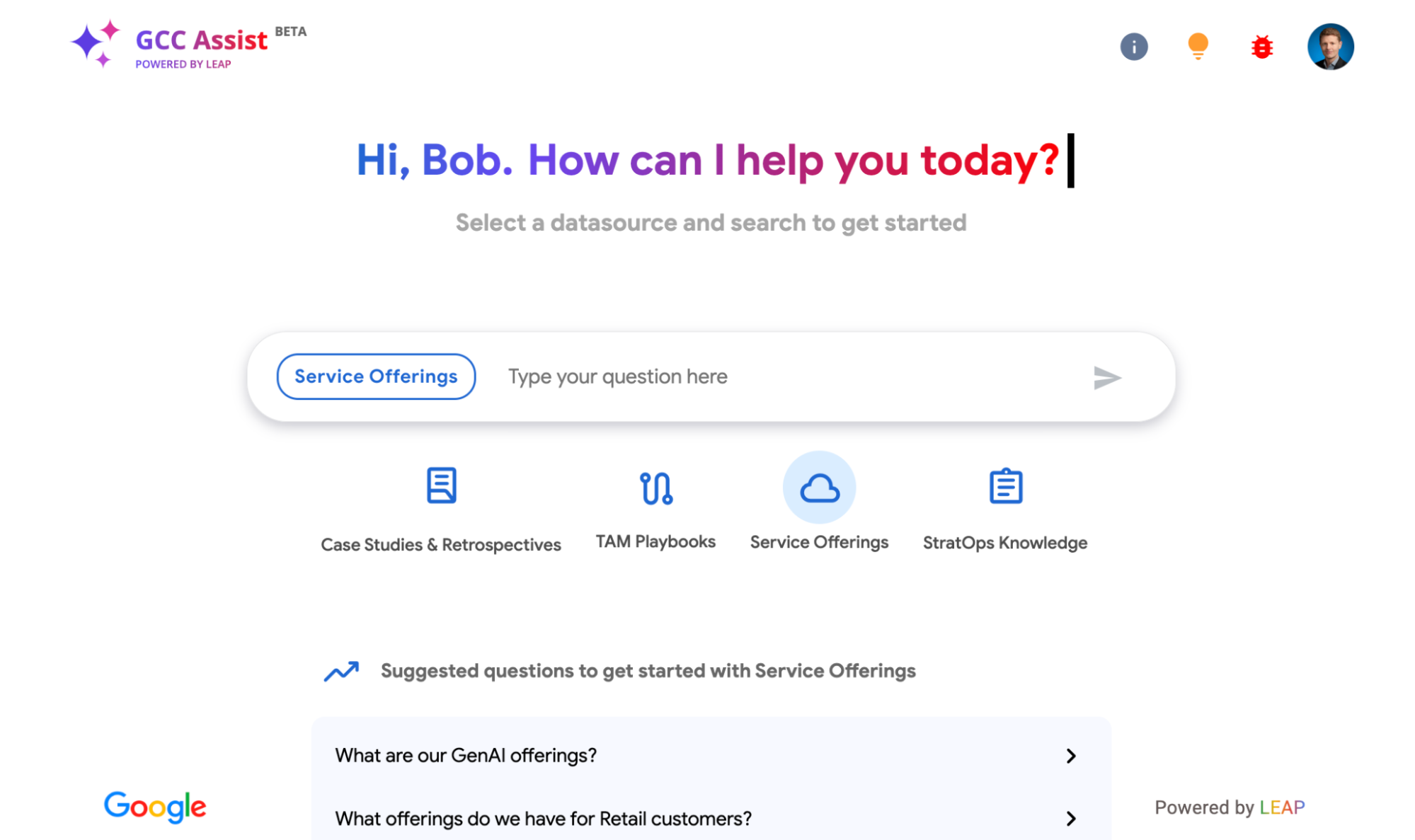
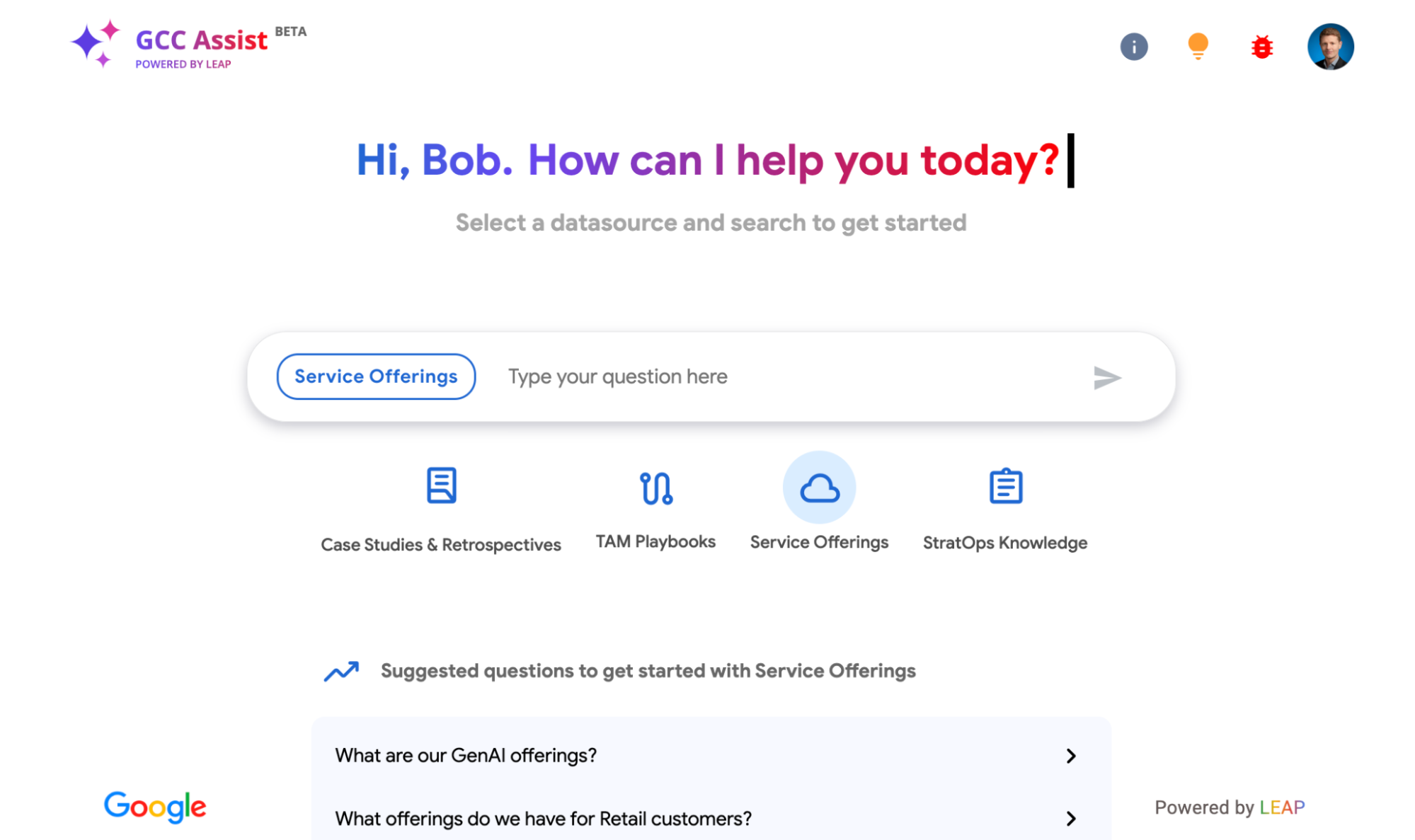
Already, we’re seeing how Vertex AI is helping our teams reduce the time it takes to search for information, prepare for customer meetings, and deliver our services. With this hub of information right at their fingertips, team members are now able to find answers and information faster, providing better support and more effective guidance to our customers.
One example of this is from Dan Roiter, a Director in Google Cloud Consulting: "I had an important meeting with a customer to discuss how we can enable a Teradata to BigQuery migration. I turned to GCC Assist with some of the inquiries I might have for a deep technical expert in this area. I was able to see sample projects, reference architecture, examples of benefits, and watchpoints. I was prepared for the customer meeting, and we were able to move forward with the program.”
2. GCC Scribe: Your generative AI contract drafting partner
Our teams also help scope customer engagements and develop statements of work (SOWs) to capture and document project plans with a customer. Research and drafting can take hours and often require several people with different areas of expertise.
To help us accelerate this process, we created GCC Scribe — a Vertex AI Vector Search powered tool to help teams write and deliver contracts and SOWs faster. With Scribe, users can search for SOWs using natural language to describe specific implementation scenarios and generate an SOW draft based on templates and previously approved examples. Scribe also provides automated contract review, which uses AI to review a contract before a user submits it, helping to flag issues and accelerate the approval process.
Challenges on the journey
We know from speaking with our customers and through our own everyday experiences that testing gen AI is usually rapid, helping to deliver quick proof-of-concepts. However, developing meaningful, production-ready gen AI applications requires building useful features with a clear impact and careful considerations around data quality, data availability, security, and privacy.
As we developed the Assist and Scribe applications, we encountered several key challenges that we encourage you to keep in mind as you start implementing your own AI projects.
-
Data preparation: The fuel for great gen AI experiences is rich and relevant data. We partnered with upstream data owners, consume the right data, normalize it, and augment it with metadata for accurate citations. This assessment helped show current and future opportunities for the data.
-
App vs. platform: To maximize the long term value, we decided to take a platform approach to building new apps rather than building them in isolation. Data is ingested once and then made available for multiple purposes and use cases. While the platform approach requires some upfront planning, it ultimately can help accelerate future feature development.
-
Personalization at scale: Every day we see new innovation emerging in interfaces, such as gen AI-powered chat features. Within Assist, users can click different buttons to ask questions within certain domains. This allows us to (1) set user expectations, (2) provide prompt engineering for each data set, and (3) provide a simple level of access control based on role type.
-
Security and privacy considerations: When it comes to data and AI in enterprise applications, security and privacy are critical concepts, which need to be considered in the early stages of planning. Addressing these issues from the beginning can help ensure that security and privacy are a fundamental part of designing features and making architectural decisions.
Our biggest takeaways from Project LEAP
Based on our experiences with implementing AI at GCC, the biggest lessons we learned along the way came back to the same central themes: data challenges, the importance of user-centric design, and truly understanding the considerations specific to AI in professional contexts.
Here are four recommendations that can help you navigate the path to successful Gen AI implementations:
-
Always do live demos and seek early feedback. “In theory” doesn’t get you far in AI deployments. Real, in-person and virtual demos are key for gaining executive buy-in and collecting accurate feedback about ideas or new product features.
-
Talk to your users. Good user experience is all about understanding the people using a product. Make sure you establish processes for users to provide constructive feedback and enable them to test things for themselves — even if it’s a work in progress.
-
Invest and take the first step. What you build now with gen AI is just a starting point, but it will be worth the investment. Your success at building with and embracing gen AI will likely be a differentiator in the future — the sooner you get started, the better.
-
Solve for yourself, solve for others. Every project is a white-label opportunity in another department, division, or company. If you build something useful for yourself, you will have opportunities to expand it.
We’re excited to share more at Google Cloud Next and stay tuned for future posts that dive into the technical implementation and underpinnings for these two solutions.
Within Google Cloud Consulting, we provide guidance to customers on how to test and productionize GenAI use cases with Vertex AI. We know every customer is on their own cloud journey and we want to meet you exactly where you are. Learn more about how Google Cloud Consulting can help you learn, build, operate and succeed.
Thank you to Kaitlin Ardiff, Gautam Bajaj, Daniel Massarsky, Robin Singh, Crispin Velez, and Vineet Verma for their leadership and collaboration on these initiatives and Sunil Rao & Lee Moore for their enthusiastic sponsorship of innovation and staying on the cutting edge with Google Cloud AI products.