IT prediction: The era of workload-optimized, ultra-reliable infrastructure is upon us

Amin Vahdat
VP/GM, ML, Systems, and Cloud AI
Try Google Cloud
Start building on Google Cloud with $300 in free credits and 20+ always free products.
Free trialEditor's note: This post is part of an ongoing series on IT predictions from Google Cloud experts. Check out the full list of our predictions on how IT will change in the coming years.
Prediction: By 2025, over half of cloud infrastructure decisions will be automated by AI and ML
Google’s infrastructure is designed with scale-out capabilities to support billions of people, powering services like Search, YouTube, and Gmail every day. To do that, we’ve had to pioneer global-scale computing and storage systems and shorten network latency and distance limitations with new innovations. Along the way, we’ve come to see cloud infrastructure as more than a simple commodity — it’s a source of inspiration and new capabilities.
But even as the demand on the industry’s cloud infrastructure continues to increase, there are simultaneously plateaus in the efficiency available from the underlying hardware. In the past, we saw annual performance gains of 30-40%, levels that often enabled a single infrastructure configuration to meet the needs of the vast majority of workloads. As these improvements have slowed and new workloads such as AI/ML and analytics have emerged, we have seen a corresponding explosion in the variety and capability of infrastructure. While empowering, the burden of picking the right combination of infrastructure components for a given workload still falls on an organization’s cloud architects.
But by 2025, we predict that the burden and complexity of infrastructure decision making will disappear through the power of AI and ML automation, which will automatically combine purpose-built infrastructure, prescriptive architectures, and an ecosystem to deliver a workload-optimized, ultra-reliable infrastructure. The focus for cloud architects will therefore be on enabling business logic and innovation, rather than how that logic maps to underlying infrastructure.
Already, we are making investments to turn this vision into reality, building custom silicon like the Infrastructure Processing Unit (IPU) for our new C3 VMs or a liquid-cooled board for the new tensor processing unit. The latter, the TPU v4 platform, is likely the world’s fastest, largest, and most efficient machine learning supercomputer. It can train large-scale workloads up to 80% faster and 50% cheaper than alternatives. Put another way, TPU v4 will nearly double the performance of critical ML and AI services at half the cost, unlocking new possibilities for what organizations can achieve when leveraging large-scale learning and inference for business services.


These same IPUs and TPUs represent the foundation that will make it possible to automate cloud infrastructure decisions. They’ll be able to support the telemetry data and ML-based analytics for proactive infrastructure recommendations that will increase the performance and reliability of workloads.
Instead of determining hardware specifications and building the right infrastructure, you’ll only need to specify a workload. AI and ML will take over the burden and recommend, configure, and identify the best options based on your budgetary, performance, and scaling requirements. What is most exciting for us is how this will enable a much more rapid pace of service innovation, which is the primary end goal of great cloud infrastructure.
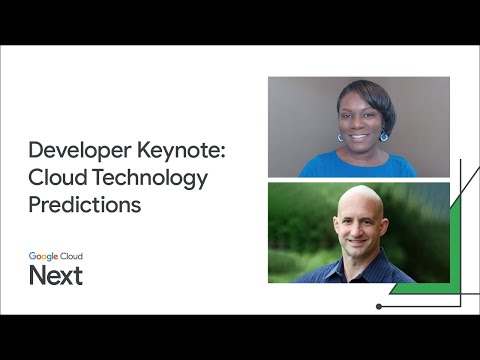