Not just compliance: reimagining DLP for today’s cloud-centric world
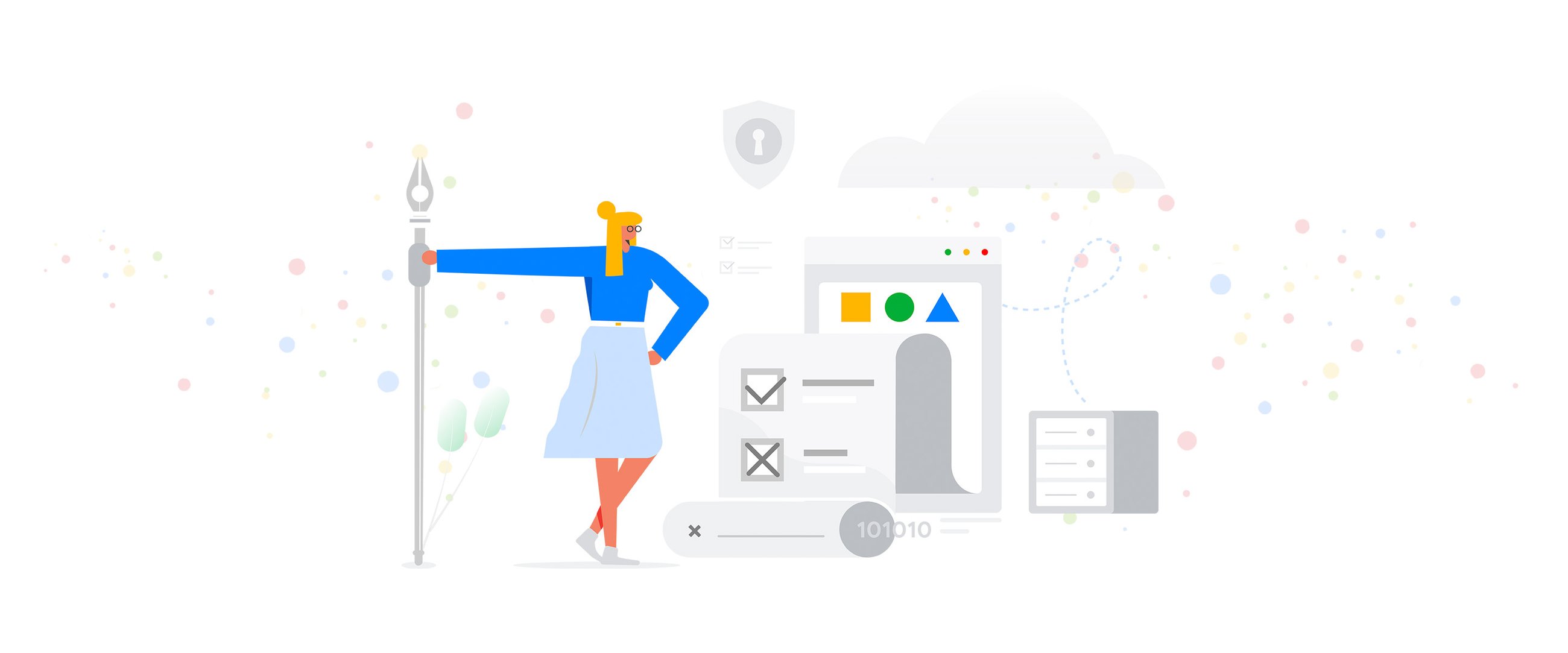
Scott Ellis
Senior Product Manager
Anton Chuvakin
Security Advisor, Office of the CISO, Google Cloud
As the name suggests, data loss prevention (DLP) technology is designed to help organizations monitor, detect, and ultimately prevent attacks and other events that can result in data exfiltration and loss. The DLP technology ecosystem—covering network DLP, endpoint DLP, and data discovery DLP—has a long history, going back nearly 20 years, and with data losses and leaks continuing to impact organizations, it continues to be an important security control.
In this blog, we’ll look back at the history of DLP before discussing how DLP is useful in today’s environment, including compliance, security, and privacy use cases.
DLP History
Historically, however, DLP technologies have presented some issues that organizations have found difficult to overcome, including:
Disconnects between business and IT
Mismatched expectations
Deployment headwinds
DLP alert triage difficulties
DLP solutions were also born in the era when security technologies were typically hardware appliances or deployable software—while the cloud barely existed as a concept—and most organizations were focused on perimeter security. This meant that DLP was focused largely on blocking or detecting data as it crossed the network perimeter. With the cloud and other advances, this is not the reality today, and often neither users nor the applications live within the perimeter.
This new reality means we have to ask new questions:
How do you reinvent DLP for today’s world where containers, microservices, mobile phones, and scalable cloud storage coexist with traditional PCs and even mainframes?
How does DLP apply in the world where legacy compliance mandates coexist with modern threats and evolving privacy requirements?
How does DLP evolve away from some of the issues that have hurt its reputation among security professionals?
DLP today
Let’s start with where some of the confusion around DLP use cases comes from. While DLP technology is rarely cited as a control in regulations today (here’s an example), for a few years it was widely considered primarily a compliance solution. Despite that compliance focus, some organizations used DLP technologies to support their threat detection mission, using it to detect intentional data theft and risky data negligence. Today, DLP is employed to support privacy initiatives and is used to monitor (and minimize the risk to) personal data in storage and in use.
Paradoxically, at some organizations these DLP domains sometimes conflict with each other. For example, if the granular monitoring of employees for insider threat detection is implemented incorrectly it may conflict with privacy policies.
The best uses for DLP today live under a triple umbrella of security, privacy, and compliance. It should cover use cases from all three domains, and do so without overburdening the teams operating it. Modern DLP is also a natural candidate for cloud migration due to its performance profile. In fact, DLP needs to move to the cloud simply because so much enterprise data is quickly moving there.
To demonstrate how DLP can work for compliance, security, and privacy in this new cloud world, let’s break down a Cloud DLP use case from each domain to illustrate some tips and best practices.
Compliance
Many regulations focus on protecting one particular type of data—payment data, personal health information, and so on. This can lead to challenges like how to find that particular type of data so that you can protect it in the first place. Of course, every organization strives to have well-governed data that can be easily located. We also know that in today’s world, where large volumes of data are stored across multiple repositories, this is easier said than done.
Let’s look at the example of the Payment Card Industry Data Security Standard (PCI DSS), an industry mandate that covers payment card data. (Learn more about PCI DSS on Google Cloud here.) In many cases going back 10 years or more, the data that was in scope for PCI DSS—i.e. payment card numbers—was often found outside of what was considered to be a Cardholder Data Environment (CDE). This pushed data discovery to the forefront, even before cloud environments became popular.
Today, the need to discover “toxic” data—i.e. data that can lead to possibly painful compliance efforts, like payment card numbers—is even stronger, and data discovery DLP is a common method for finding this “itinerant” payment data. When moving to the cloud, the same logic applies: you need to scan your cloud resources for card data to assure that there is no regulated data outside the systems or components designated to handle it.
This use case is something that should become part of what PCI DSS now calls “BAU,” or business as usual, rather than an assessment-time activity. A good practice is to conduct a periodic broad scan of many locations followed by a deep scan of “high-risk” locations where such data has been known to accidentally appear. This may also be combined with a deep and broad scan before each audit or assessment, whether it’s quarterly or even annually.
For specific advice on how to optimally configure Google Cloud DLP for this use case, review these pages.
Security
DLP technologies are also useful in security risk reduction projects. With data discovery, for example, somes obvious security use cases include detecting sensitive data that’s accessible to the public when it should not be and detecting access credentials in exposed code.
DLP equipped with data transformation capabilities can also address a long list of use cases focused on making sensitive data less sensitive, with the goal of making it less risky to keep and thus less appealing to cyber criminals. These use cases range from the mundane, like tokenization of bank account numbers, to esoteric, like protecting AI training data pipelines from intentionally corrupt data. This approach of rendering valuable, “theft-worthy” data harmless is underused in modern data security practice, in part because of a lack of tools that make it easy and straightforward, compared to, say, merely using data access controls.
Where specifically can you apply this method? Account numbers, access credentials, other secrets, and even data that you don‘t want a particular employee to see, such as customer data, are great candidates. Note that in some cases, the focus is not on making the data less attractive to external attackers, but reducing the temptation to internal attackers looking for a low hanging fruit.
Privacy
Using DLP for privacy presented a challenge when it was first discussed. This is because some types of DLP—such as agent-based endpoint DLP—collect a lot of information about the person using the system where the agent is installed. In fact, DLP was often considered to be a privacy risk, not a privacy protection technology. Google Cloud DLP, however, was born as a privacy protection technology even before it became a security technology.
However, types of DLP that can discover, transform, and anonymize data—whether in storage or in motion (as a stream)—present clear value for privacy-focused projects. The range of use cases that involve transforming data that’s a privacy risk is broad, and includes names, addresses, ages (yes, even age can reveal the person's identity when small groups are analyzed), phone numbers, and so on.
For example, let’s look at the case when data is used for marketing purposes (such as trend analysis), but the production datastores are queried. It would be prudent in this case to transform the data in a way that retains its value for the task at hand (it still lets you see the right trend), but destroys the risk of it being misused (such as by removing the bits that can lead to person identification).
There are also valuable privacy DLP use cases in the area where two datasets with lesser privacy risk are combined, creating a data set with dramatically higher risks. This may come, for example, from a retailer merging a customer’s shopping history with their location history (such as visits to the store). It makes sense to measure the re-identification risks and transform the datasets either before or after merging to reduce the risk of unintentional exposure.
What’s next
We hope that these examples help show that modern cloud-native DLP can be a powerful solution for some of today’s data challenges.If you’d like to learn more about Google Cloud DLP and how it can help your organization, here are some things to try:
First, adopt DLP as an integral part of your data security, compliance, or privacy program, not a thing to be purchased and used standalone
Second, review your needs and use cases, for example the types of sensitive data you need to secure
Third, review Google Cloud DLP materials, including this video and these blogs. For privacy projects, review our guidance on de-identification of personal data, specifically.
Fourth, implement one or a very small number of use cases to learn the specific lessons of applying DLP in your particular environment. For example, for many organizations the starting use case is likely to be scanning to discover one type of data in a particular repository.
We built Google Cloud DLP for this new era, its particular use cases, and its cloud-native technology. Check out our Cloud Data Loss Prevention page for more resources on getting started.