How TEKsystems Global Services is helping retail brands break down data silos through platform modernization
Mohamed Barry
Data Analytics Partner Engineer, Google
Pradipta Dhar
Technical Lead - GCP Practice, TEKsystems Global Services
Retail brands face the challenges of data duplication and data silos as businesses and technology stacks grow — while consumer expectations remain high. These challenges not only increase the complexity of data management but can also lead to a rise in overall costs. By leveraging platform modernization services within Google Cloud, such as BigQuery, BigLake, and Dataplex, retail brands can help drive faster analytics, avoid unauthorized data access risks and reduce overall security and governance challenges. Let’s break down what retail brands experience and how Google Cloud can help overcome these challenges.
Data silos can increase complexities and lower productivity
Data silos refers to compartmentalization of information that arises when different departments within a retail organization manage their data independently. For example, marketing, sales, supply chain, logistics, and customer service teams often utilize distinct systems to track and store their data. This compartmentalization, although convenient initially, can lead to added complexities as processes grow and evolve with the growth of data volumes and business needs.
The retail industry is a mammoth data machine reliant on a well-oiled data analytics engine at its heart. The data flows in from different sources where it is then stored in different systems and in different formats. This data is also subject to change from time to time that can make cross departmental collaboration difficult and error prone. Here are how these challenges can impact retail businesses:
- Limited visibility and insights: Data silos prevent retailers from having a comprehensive view of their customers, operations, and overall business performance. This makes it difficult to gain insights into customer behavior, identify trends, and make informed decisions.
- Inefficient operations: Data silos can lead to inefficiencies in various operational areas, such as inventory management, supply chain optimization, and customer service. When data is scattered across different systems, it increases the risk of data inconsistencies.
- Limited marketing reach: Data silos prevent retailers from effectively targeting and personalizing their marketing campaigns. Without a unified view of customer data, it's challenging to understand customer preferences, interests, and purchase patterns.
- Limited access control: Data silos can make it difficult to implement and enforce consistent access control policies. This can increase the risk of unauthorized access to sensitive data.
The most efficient way to address concerns about data silos and security while working with retail data from various sources is to build a data mesh.
What is a Data Mesh?
A data mesh is a new approach to data architecture that emphasizes autonomy, governance, and scalability. It is designed to address the challenges of traditional data architectures, which are often monolithic and inflexible.
A data mesh is a decentralized, scalable, and modular architecture for managing data. It is designed to address the challenges of data silos, which can make it difficult to find, use, and share data across an organization.
In a data mesh, data is owned and managed by domain experts, who are responsible for ensuring that the data is accurate, complete, and up-to-date. The data is then published to a central catalog, where it can be discovered and used by other parts of the organization.
Building a secure data mesh with Google Cloud
A data mesh works well with an analytics lake house. An analytics lake house is an architecture that combines the benefits of a data lake and a data warehouse. It provides a centralized repository for data, while also allowing for the use of analytical tools and processes that are typically associated with data warehouses.
The data mesh can provide a way to manage the data in the lake house, while the lake house can provide a place to store and analyze the data. The key benefits of the lake house architecture in the retail industry are:
- Improved customer experience: Customers will no longer need to reach out to multiple different departments to get complete information on questions spanning department boundaries as the data will be easily accessible by all departments as long as they have jurisdiction over it.
- Improved efficiency at lower costs: Retailers will no longer need to bear extra expenses of storing duplicate data and moving data across teams/platforms.
- Securing data without silos: With fine-grained access control, data stewards can ensure data is kept secure with access being provided in accordance with the principle of least privileges.
- Improved data governance: With Dataplex, data stewards and administrators can build a logical data mesh and governance layer to implement governance, validation and cataloging of data from a single interface.
Retail organizations often work with a centralized data lake where data from different sources such as sales, marketing, inventory, supply chain, etc., come in. This data often comes in unprocessed. From there, different analytics teams fetch the data, process it and then generate reports for business users.
BigQuery enables data stewards to import data into native tables called Capacitor. They can grant access to the data at various asset levels. For example, the finance team would not have access to HR data and the supply chain team would not have access to sales data. For organizations in a multi-cloud architecture, BigLake also enables the creation of BigQuery assets on data residing outside of Google Cloud in AWS and Azure.
What about data residing in Cloud Storage? Data in this object store is neither easily queryable nor suitable for running analytics on directly. BigLake solves this problem by allowing the creation of BigLake tables on Cloud Storage data. This avoids data duplication and movement. Finally, you can apply fine-grained access controls on these tables to securely share these assets with different analytics teams while the data stays in place.
Dataplex plays the governing role in building this data mesh by organizing data in Cloud Storage and BigQuery into a hierarchy of lakes, zones, and assets. Within each lake, you can create zones for subcategories such as region or business unit. You can also separate zones into raw and curated data, do data validation and auto-discovery to flag pattern changes for incoming data that can potentially break downstream processes.
Once the data mesh is built out, analysts can use BigQuery to run their analytics on popular open source engines for things like sales predictions, supply-chain analysis, or inventory estimations. The Google Cloud services discussed in this article integrate seamlessly with other Google Cloud data portfolio services like Dataflow and Dataproc, allowing users to build robust and powerful data pipelines.
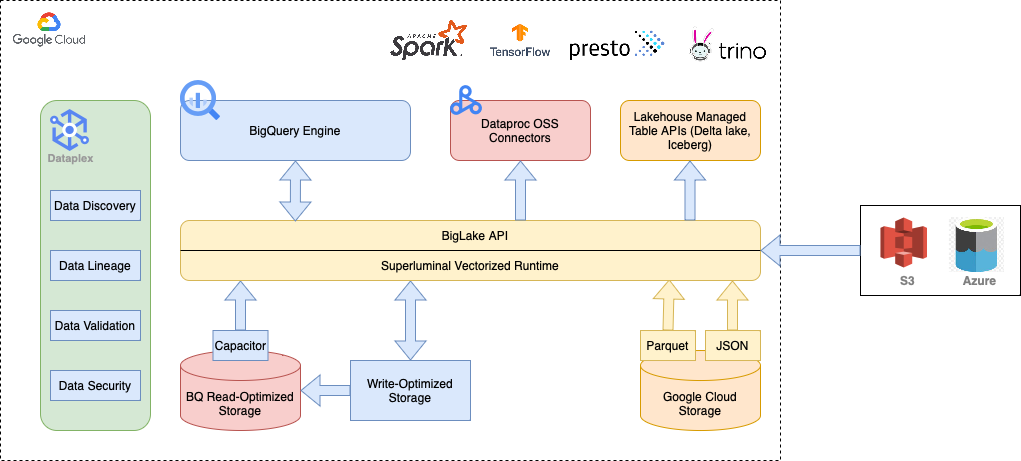
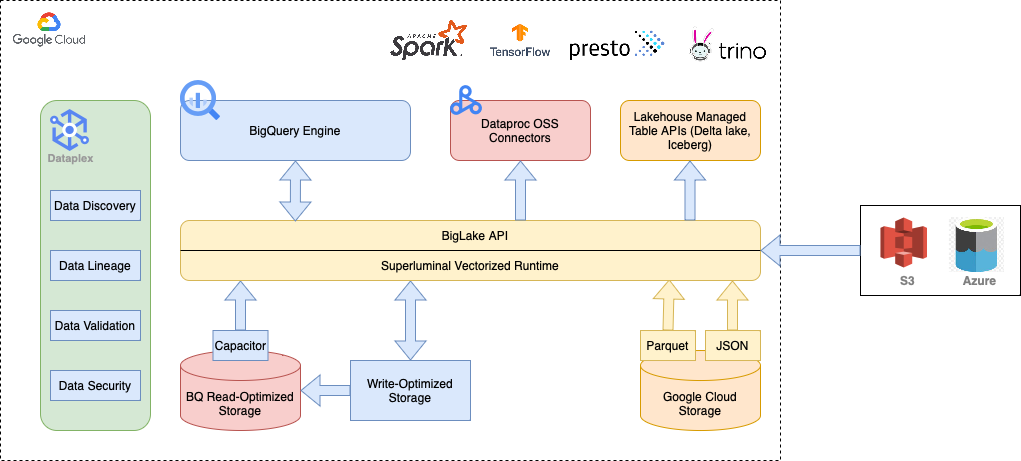
Google Cloud can simplify data management, enabling retail brands to exceed consumer expectations. Using these platform modernization services within Google Cloud, businesses can not only avoid data duplication, but they can also reduce costs and down silos without compromising on security.
To learn more about how TEKsystems Global Services is helping businesses take advantage of Google Cloud, please visit www.TEKsystems.com.