How to achieve more reliable analytics with the Monte Carlo integration for Looker
Molly Vorwerck
Head of Content, Monte Carlo
Try Google Cloud
Start building on Google Cloud with $300 in free credits and 20+ always free products.
Free trialAs companies increasingly leverage data-driven insights to drive innovation and maintain their competitive edge, it’s important that their data is accurate and reliable at all stages of the data pipeline.
While powerful solutions exist to model, transform, and visualize data, many data teams cite data quality and reliability as key pain points, costing them wasted revenue and up to 40 percent of their team’s time. In fact, 1 in 5 companies have lost a customer due to incomplete or erroneous data. Instead of working on projects that actually move the needle for their company, data professionals are forced to debug data pipelines and firefight broken dashboards.
To address this “data downtime,” data analysts, engineers, and scientists need an easy and collaborative way to monitor and alert for abnormalities in their data, from ingestion in the data warehouse or lake, through ETL and analytics. It’s also important they have the tools necessary to map their data lineage, keeping tabs on how changes in their data pipelines affect downstream sources, such as analytics and business intelligence reports.
How to monitor your data quality with with Monte Carlo integration for Looker
With Monte Carlo’s Looker integration, data teams can now identify and prevent inaccurate, missing, or erroneous data from breaking your analytics dashboards, delivering more reliable insights and eliminating data downtime.
The Looker integration for Monte Carlo enables users to seamlessly monitor and alert for issues with the data feeding their Looker dashboards, giving them a holistic view of the health and reliability of their data assets.
The Monte Carlo Data Observability engine automatically monitors key features of your data, including data freshness, distribution, volume, schema, and lineage. Without the need for manual threshold setting, you can find the answers to questions such as:
- When was my table last updated?
- Is my data within an accepted range?
- Is my data complete? (Did 2,000 rows suddenly turn into 50?)
- Who has access to our marketing tables? Who made changes to them?
- Where did my data break? Which tables or reports were affected?
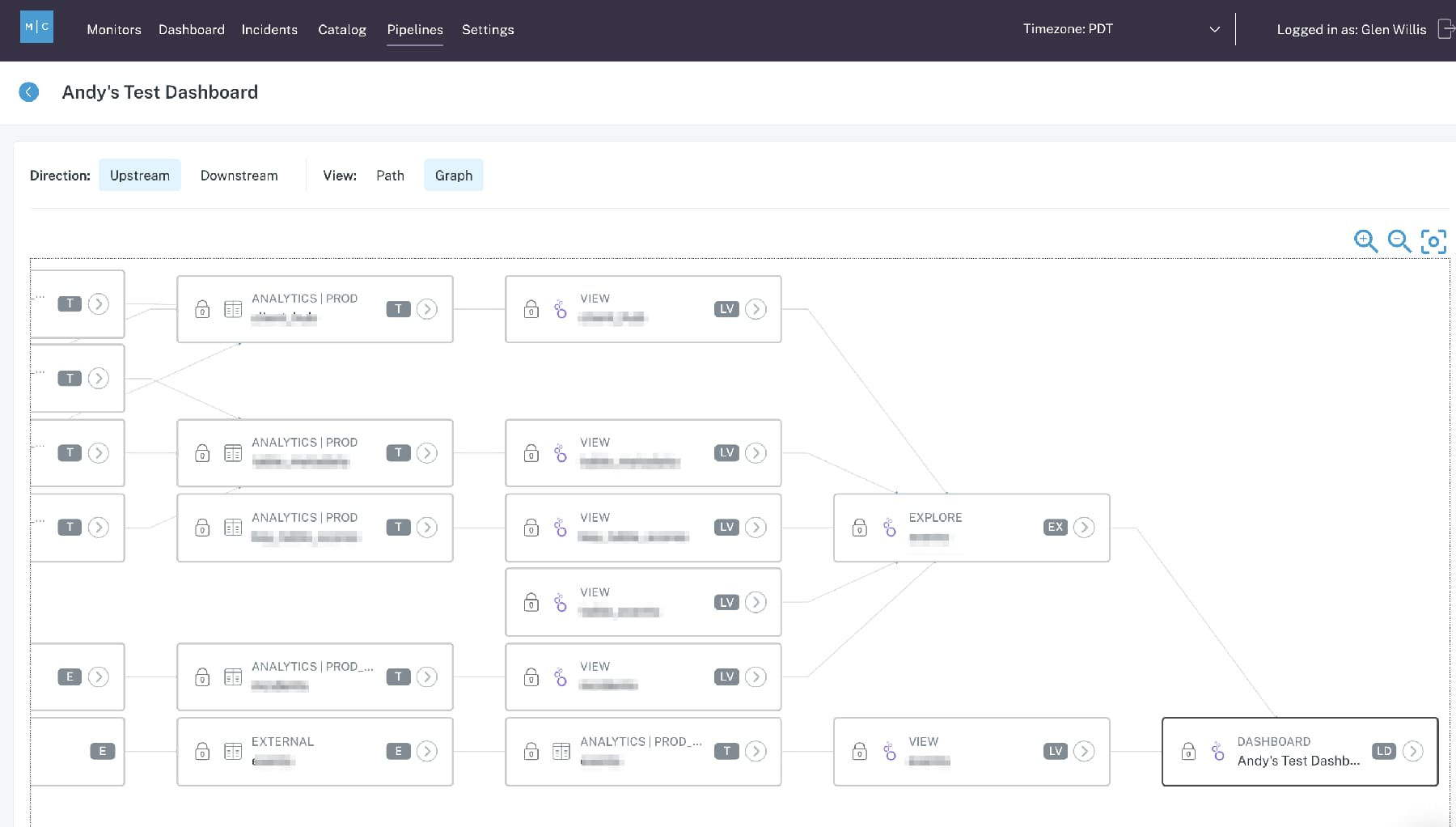
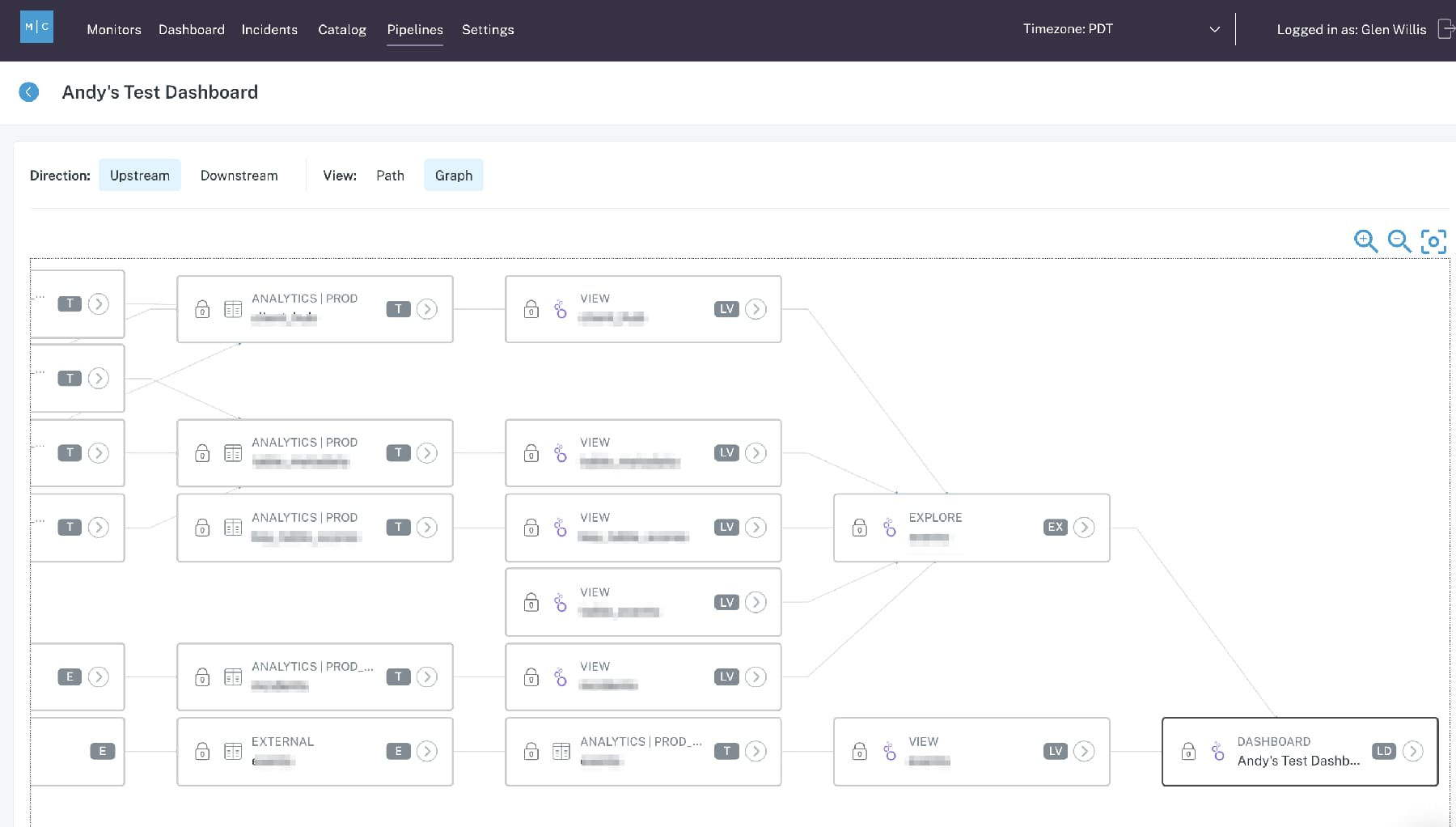
With this partnership, mutual customers can now trace field-level lineage all the way down to Looks, Explores, reports, and dashboards, facilitating greater visibility into the health of their data pipelines and the insights those pipelines deliver. Such functionality allows data teams to investigate why their Looker dashboards aren’t pulling the freshest data for stakeholders (for instance, is there a missing data set? A null value? Did someone use the CSV file type instead of XLS?).
One such customer, Compass, uses Monte Carlo to keep tabs on the upstream and downstream dependencies for their Looker dashboards.
“Monte Carlo’s monitoring feed gives me comfort that our Looker dashboards are healthy and everything’s working as designed. And on days where something goes wrong, I know my team will be the first to know and that we’ll be in command of the situation,” said Suvayan Roy, Senior Product Manager, Compass.
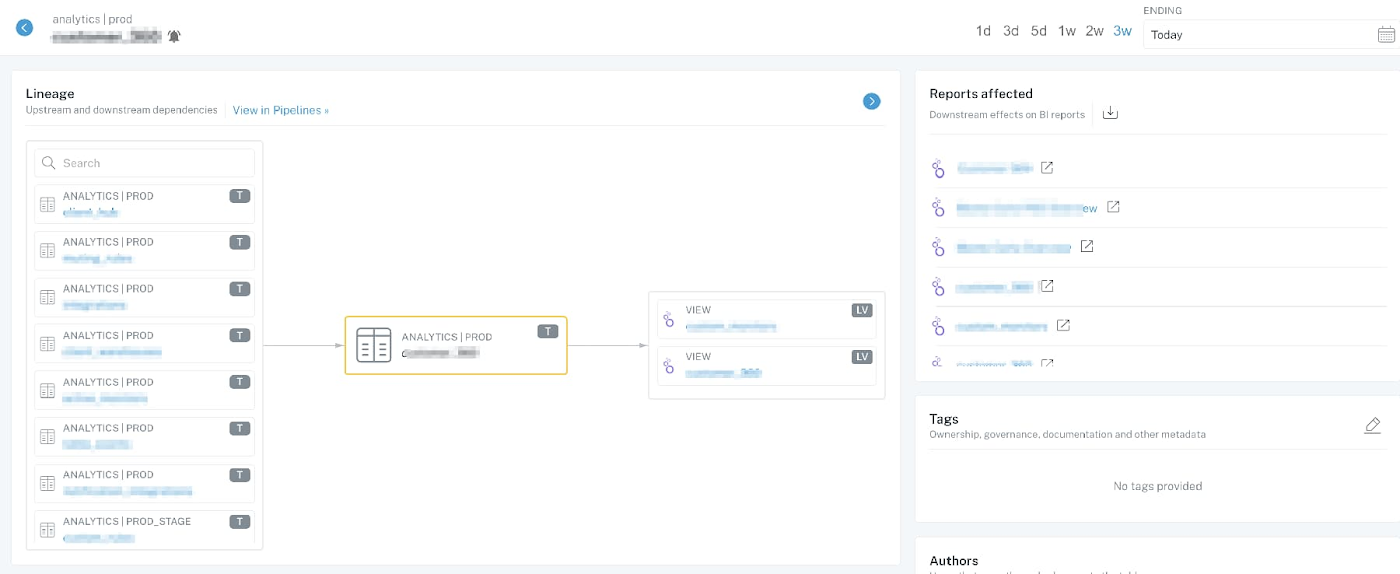
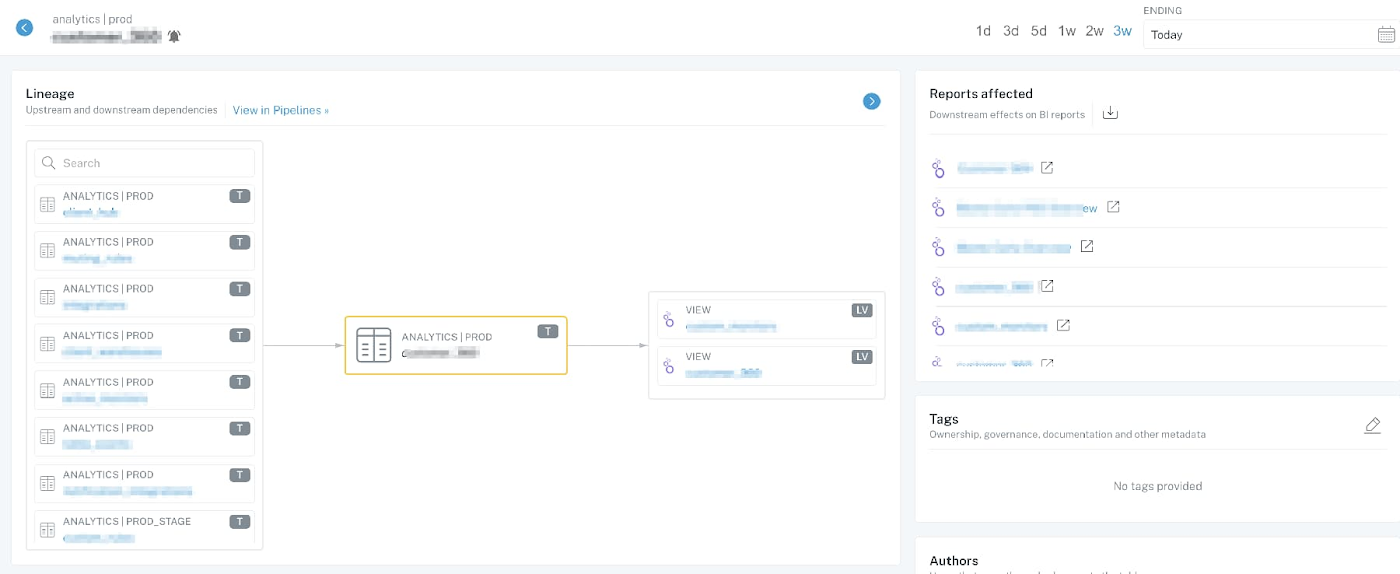
With this new integration, Looker and Monte Carlo have teamed up to streamline this process and give data teams visibility into their data health through full, end-to-end data observability.
How to get started with Monte Carlo and Looker
To get started with Monte Carlo and Looker:
- Create an API key on Looker, allowing Monte Carlo to access metadata about your dashboards, Looks, and other Looker objects.
- Connect Monte Carlo with your LookML code repository, allowing Monte Carlo to map dependencies between Looker objects and data warehouse tables.
For more details on the Monte Carlo - Looker integration (or to get started), please reach out to the teams at Monte Carlo and Looker.